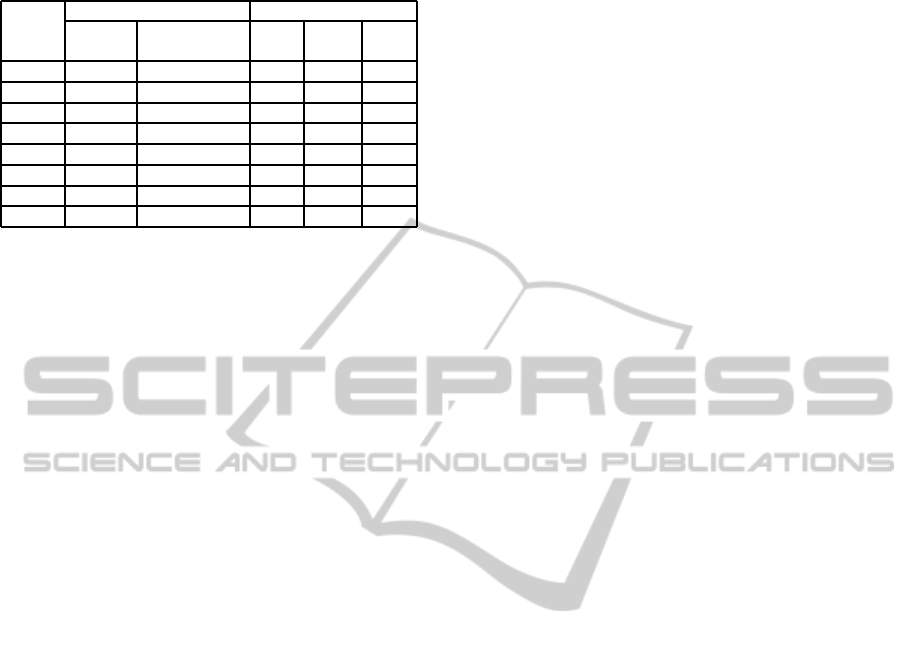
Table 3: Consumptions obtained in scenario C.
Train
Consumption (LGTT) Reduction
Actual DCOP Our C-A C-B
(A) (B) (C) (%) (%)
1 6.19 4.16 3.36 50% 26%
2 5.68 4.18 4.22 30% -5%
3 6.23 4.09 3.95 41% 10%
4 6.49 4.51 3.88 46% 23%
5 6.29 4.22 3.31 49% 24%
6 6.17 3.99 3.69 40% 8%
7 6.26 4.07 3.86 42% 11%
8 5.68 4.41 4.00 34% 6%
sented (Our column). The DCOP column represents
the best values obtained in this approach. It is em-
phasized that for all consumption values (measured
in LGTT), our approach is higher than for the other
competitors, except on a single opportunity (train 2),
where the DCOP is higher by 5%.
The feasibility of an automatic train driving sys-
tem seems significantly important. For example, for
a fuel consumption expenditure of approximately 250
million dollars per year, any cost savings above 6%
can have a significant impact on the competitiveness
of the freight transport sector.
5 CONCLUSIONS
This paper presented a collaborative approach for
sharing experiences in generating plans for driving
trains. The results obtained showed that the adopted
approach can be generalized and deployed at various
stations of a rail network. We showed that the effi-
ciency of recovery and adaptation tasks increases as
new cases are obtained. Such efficiency generates a
tendency to reduce efforts in planning and re-planning
driving plans. Obviously, if conditions change signif-
icantly, planning efforts increase, at least initially.
Finally, in terms of domain application, two re-
sults are important: in monetary terms, the gener-
ated driving plans can produce significant gains; and
in terms of reuse of experiences, the approach sug-
gested that good drivers should be used to drive trains
in several different stretches of a railroad, for a certain
time, in order to generate experiences. Such experi-
ments can then be used to generate good plans for less
experienced drivers. This helps rationalize the exper-
tise capable for driving trains efficiently. Future work
should follow the following directions: avoiding un-
necessary stops (Dordal et al., 2011), and ensuring the
certification of the information exchanged.
REFERENCES
Aamodt, A. and Plaza, E. (1994). Case-based reasoning:
Foundational issues, methodological variations, and
system approaches. AI Commun., 7(1):39–59.
Baeck, T., Fogel, D., and Michalewicz, Z. (2000). Evolu-
tionary Computation 1: Basic Algorithms and Opera-
tors. Basic algorithms and operators. Taylor & Fran-
cis.
Bajo, J., Corchado, J., and Rodr´ıguez, S. (2007). Intelli-
gent guidance and suggestions using case-based plan-
ning. In Weber, R. and Richter, M., editors, Case-
Based Reasoning Research and Development, volume
4626 of Lecture Notes in Computer Science, pages
389–403. Springer Berlin Heidelberg.
Borges, A., Dordal, O., Sato, D., Avila, B., Enembreck,
F., and Scalabrin, E. (2012). An intelligent system
for driving trains using case-based reasoning. In Sys-
tems, Man, and Cybernetics (SMC), 2012 IEEE Inter-
national Conference on, pages 1694–1699.
Company, G. R. S. (1979). Elements of railway signaling.
General Railway Signal.
D., E. and Houpt, P. (2011). Trip optimizer for railroads.
Technical report, IEEE Control Systems Society.
Dordal, O., Borges, A., Ribeiro, R., Enembreck, F., Scal-
abrin, E., and Avila, B. (2011). Strong reduction in
fuel consumption driving trains in bi-directional sin-
gle line using crossing loops. In Systems, Man, and
Cybernetics (SMC), 2011 IEEE International Confer-
ence on, pages 1597–1602.
Fang, W., Sun, J., Wu, X., and Yao, X. (2013). Re-
scheduling in railway networks. In Computational In-
telligence (UKCI), 2013 13th UK Workshop on, pages
342–352.
Gu, Q., Cao, F., and Tang, T. (2012). Energy efficient
driving strategy for trains in mrt systems. In Intelli-
gent Transportation Systems (ITSC), 2012 15th Inter-
national IEEE Conference on, pages 427–432.
Hengyu, L. and Hongze, X. (2012). An integrated intel-
ligent control algorithm for high-speed train ato sys-
tems based on running conditions. In Digital Man-
ufacturing and Automation (ICDMA), 2012 Third In-
ternational Conference on, pages 202–205.
Khan, M. J., Awais, M. M., Shamail, S., and Awan,
I. (2011). An empirical study of modeling self-
management capabilities in autonomic systems using
case-based reasoning. Simulation Modelling Practice
and Theory, 19(10):2256 – 2275.
Kolodner, J. (1993). Case-based Reasoning. Morgan Kauf-
mann Publishers Inc., San Francisco, CA, USA.
Loumiet, J., Jungbauer, W., and Abrams, B. (2005). Train
Accident Reconstruction and FELA and Railroad Lit-
igation. Lawyers & Judges Publishing Company.
Luke, S., Panait, L., Balan, G., and Et (2014). Ecj 21: A
java-based evolutionary computation research system.
Magalh˜aes Mendes, J. (2013). A comparative study of
crossover operators for genetic algorithms to solve the
job shop scheduling problem. WSEAS Transactions
on Computers, 12(4):164–173.
ICEIS2015-17thInternationalConferenceonEnterpriseInformationSystems
450