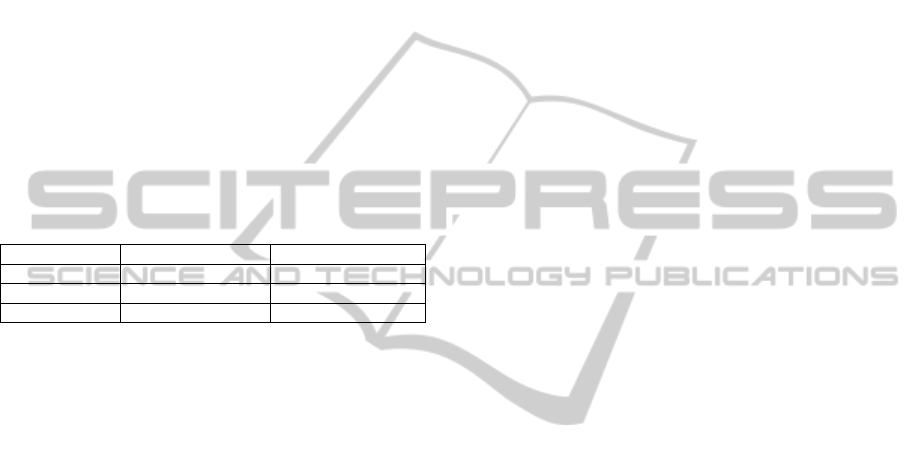
3 RESULTS AND DISCUSSION
Cross-validation statistics analysis was performed on
data for the three fields with a low-, high- and
variable-risk based on spatial distribution of CFU
(Table 1). These cross-validation statistics are used
to determine how well the indicator Kriging
equation interpolated the V. dahliae CFU numbers
for each of the three fields. The closer the RMSE is
to zero, the closer the prediction is to the measured
values (Robinson and Metternicht, 2006). All three
fields had RMSE values relatively close to zero
meaning that the model derived from the data points
in each of the respected fields accurately predicted
the probability of any point in space within the field
being greater than the threshold of 5 CFU/10 g of
soil.
Table 1: Cross-validation parameter root mean squared
error (RMSE).
Field RMSE
a
RMSSE
1 0.1133264 0.953032
2 0.3442308 1.145598
3 0.4960541 1.034625
a
Root mean squared error, the root value of the mean squared
error
b
Root mean squared standardized errors The closer to 1, the more
accurate the prediction of variability for that model
The RMSSE shows the model’s successfulness
in assessing variability. The closer the RMSEE is to
1, the more successful the prediction of variability
for that model was (Robinson and Metternicht,
2006). The calculations using the indicator Kriging
equations above for each of the three fields of
interest showed high levels of accuracy in predicting
and assessing variability. Each of the three equations
preformed well in regards to how accurate the
predictions of the established threshold probability
(CFU > 5 CFUs/10 g of soil) at points that were not
sampled.
Conditional probability maps were generated for
the three individual fields (Figure 2). These maps
spatially represented the probability of PED
incidence based on a 5 CFU/10 g of soil threshold. A
conditional probability map was generated of the
low-risk field (Figure 2A). Based on the 20 original
V. dahliae CFU values and a threshold value of 5
CFU/10 g of soil, the indicator Kriging model
developed for this field predicts a low incidence of
PED. The small portion of the field colored red had
a probability from 0.95 to 1 of PED. The majority of
the field, colored in blue had a probability between 0
and 0.1 for PED. A conditional probability map was
generated of the high-risk field (Figure 2B). The
majority of this field had a probability between 0.95
and 1 for PED. This is quite a contrast from the low-
risk field. Finally a conditional probability map was
generated of the variable-risk field (Figure 2C). The
result is a map where the probability of being above
the established PED threshold varied throughout the
field.
The visualized differences among these three
maps shows how the use of conditional probability
can be used to predict the spatial distribution of plant
diseases in the soil and provide and informational
tool for commercial potato growers. In an effort to
help reduce inoculum levels of V. dahliae and other
soilborne pathogens, growers will often elect to use
soil fumigants. For many years, soil fumigants such
as methyl bromide were used, with great
effectiveness, to eliminate soilborne plant pathogens
such as V. dahliae (Wilhelm and Paulus, 1980,
Wilhelm et al., 1961, Ebben et al., 1983). More
recently, the commercial agriculture industry has
phased out the use of methyl bromide due to its
negative effect on the environment (Thomas, 1996).
New soil fumigants such as metam sodium and
chloropicrin have taken the place of methyl bromide
but as researchers begin to better understand the role
of beneficial soil microorganism related to plant
health (Hayat et al., 2010) the use of any broad-
spectrum fumigant is being re-evaluated in a new
context. While these soil fumigants may control
soilborne pathogens, they may be, in effect, reducing
the beneficial soil microorganism populations that
assist in plant growth and natural defence against
plant pathogenic bacteria and fungi.
The accessibility of conditional probability maps
could become a useful informational tool for
growers implementing integrated pest management.
Rather than making crop management decisions for
a field’s acreage as a whole, a grower would be able
to assess each field individually, or even at the sub-
field level to determine problem fields or areas of
the field that would benefit from soil fumigation. If
the grower maintained a low-risk field (Figure 2A),
they could use conditional probability as a holistic
management tool to determine no need for
fumigation in that field based on the PED risk.
Conversely, if the grower assesses the conditional
probability for PED and the results indicate a high-
risk for PED above the established
threshold (Figure
2B), the grower may elect to treat with applications
of soil fumigants. Lastly, if a grower is managing a
variable-risk field for PED (Figure 2C), this would
allow the grower to make decisions based on a sub-
field management approach and only apply fumigant
to the portions of the field that present a greater
GISTAM2015-1stInternationalConferenceonGeographicalInformationSystemsTheory,ApplicationsandManagement
198