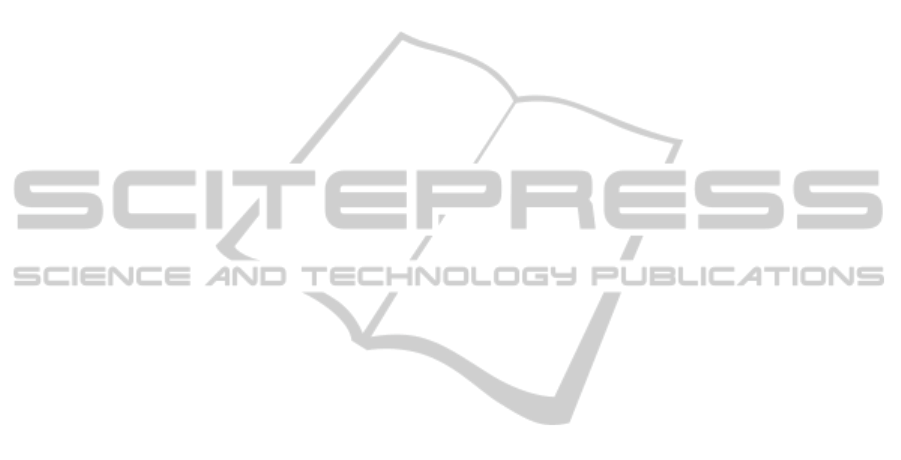
the idea of segmenting a scene and transferring the
information based on regions. Such an approach is
presented in (Tighe and Lazebnik, 2013b), the so-
called Superparsing. The main idea can be described
in three steps. First, a set of global image features,
GIST, Bag-of-Features & a color histogram are com-
puted. Then, for a given query image the most similar
images are retrieved from an annotated dataset. Sec-
ond, the query image and the retrieval set are over-
segmented, each segment creating a set of pixels that
contains some context information, so-called super-
pixels. Each superpixel is also described by a set of
features that cover shape, location, texture, color and
appearance information. The complete set of global
& local features is described in (Tighe and Lazebnik,
2013b). Third, for each superpixel in the query image
the most similar superpixels from the retrieval set are
used in order to obtain a label.
The approach has been extended by contextual in-
ference, cf. (Tighe and Lazebnik, 2013b). An addi-
tional classifier for geometric classes (horizontal, ver-
tical, sky) is evaluated and the semantic labels for the
regions are compared to their geometric counterpart.
For example, a street is a horizontal entity and a build-
ing a vertical one. Furthermore, the neighboring su-
perpixels are taken into account, for example, a car is
unlikely to be surrounded by water or the sky. Both
conditions are integrated into a conditional random
field and used for re-weighting the classwise proba-
bilities for the semantic class labels. In (Tighe and
Lazebnik, 2013a) an extension has been proposed that
combines the superparsing approach with the output
of different per object detectors in order to improve
the results for a given set of categories.
In (Farabet et al., 2013) another region-based ap-
proach has been proposed. Instead of extracting de-
signed local image descriptors, like SIFT or HOG, a
multi-scale convolutional network is integrated into
the image parsing. The input image is transformed
with a Laplacian pyramid and a convolutional net-
work is applied to the transformed images in or-
der to compute feature maps. Again instead of a
pixel-wise evaluation of these feature maps a set
of regions is evaluated that is created by either an
over-segmentation or a multi-scale approach creating
coarse and fine sets of regions using the same over-
segmentation algorithm.
These region-based scene representations are also
very similar to the coarser analysis that is performed
in the automotive industry. Here, a column based ap-
proach and depth differences in the 3D space are used
for creating the regions, the so-called stixels (Badino
et al., 2009). Natural scenes are then labeled based on
these stixels which allows for detecting a set of rele-
vant objects, like cars, persons or buildings.
Even though several extensions have been pro-
posed, a crucial part of these state-of-the-art meth-
ods is the underlying segmentation algorithm that
computes the superpixels. All region-based ap-
proaches are based on the segmentation algorithm
from (Felzenszwalb and Huttenlocher, 2004) in order
to create an over-segmentation. In this paper the in-
fluence of different superpixel methods will be eval-
uated. Based on a benchmark that evaluates the ef-
ficiency of different algorithms that compute super-
pixels, suitable methods are chosen. Furthermore,
a new method that computes a superpixel-like over-
segmentation of an image is presented that computes
the regions based on edge-avoiding wavelets. The
methods are then evaluated within the Superparsing
framework from (Tighe and Lazebnik, 2013b) on the
SIFT Flow and Barcelona dataset. The experiments
will show that the choice of the superpixel method
is crucial for the performance of the image parsing
and that the edge preserving property of the proposed
method can improve image parsing.
2 SUPERPIXEL METHODS
In the following section we will review a few su-
perpixel methods. An extensive evaluation of dif-
ferent superpixel methods is given in (Neubert and
Protzel, 2012). Here, the approaches were selected
under the aspects of segmentation accuracy, robust-
ness and computational efficiency. Segmentation ac-
curacy is defined by the over-segmentation error & the
overlap between the border of a semantic object and
a superpixel. Robustness is defined with respect to
transformations such as translation, rotation and scal-
ing. Computational efficiency describes the runtime
for computing a given number of superpixels on an
image. The efficient graph-based image segmenta-
tion algorithm from (Felzenszwalb and Huttenlocher,
2004), Quick Shift (Vedaldi and Soatto, 2008) and
Simple Linear Iterative Clustering (SLIC) (Achanta
et al., 2012) are in the set of best performing algo-
rithms for all criteria and, therefore, are evaluated for
image parsing.
2.1 Efficient Graph-based Image
Segmentation
The efficient graph-based image segmentation
method has been introduced in (Felzenszwalb and
Huttenlocher, 2004). It splits an image into regions
by representing it as a graph and combining similar
subgraphs. Therefore, an image is interpreted as a
OntheInfluenceofSuperpixelMethodsforImageParsing
519