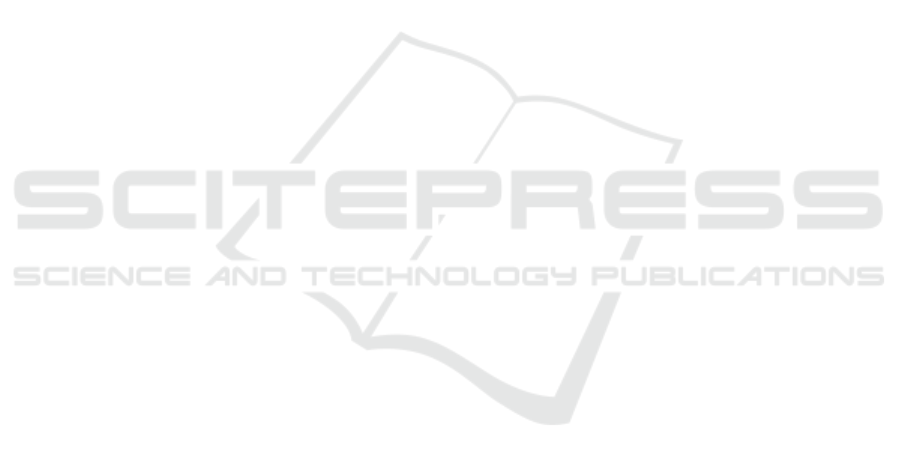
Edge based Foreground Background Estimation with Interior/Exterior
Classification
Gianni Allebosch, David Van Hamme, Francis Deboeverie, Peter Veelaert and Wilfried Philips
Image Processing and Interpretation Group (IPI), Department of Telecommunications and Information Processing
(TELIN), Ghent University - iMinds, St-Pietersnieuwstraat 41, 9000, Ghent, Belgium
Keywords:
Foreground Background, Edge Motion, Local Ternary Patterns, Interior.
Abstract:
Foreground background estimation is an essential task in many video analysis applications. Considerable
improvements are still possible, especially concerning light condition invariance. In this paper, we propose a
novel algorithm which attends to this requirement. We use modified Local Ternary Pattern (LTP) descriptors
to find likely strong and stable “foreground gradient” locations. The proposed algorithm then classifies pixels
as interior or exterior, using a shortest path algorithm, which proves to be robust against contour gaps.
1 INTRODUCTION
Over the years, many foreground background seg-
mentation methods have been proposed. Several of
them are variations on the classical Gaussian Mixture
Model (GMM) (Stauffer and Grimson, 1999) con-
cept. It is assumed that the frequency of an appear-
ance level (intensity, RGB . . . ) occurring at a cer-
tain pixel can be modelled statistically as a mixture
of Gaussian distributions. The more recent ViBe al-
gorithm (Barnich and Droogenbroeck, 2011) and its
successor ViBe+ (Droogenbroeck and Paquot, 2012)
are built on similar principles as the GMM, but store
the distributions as a collection of samples rather than
by the model parameters.
Pixel appearances can change drastically un-
der changing lighting conditions (Cristani et al.,
2010). The previously described methods handle
light changes poorly. Edge based approaches on
the other hand are able to produce stable results un-
der illumination changes (Gruenwedel et al., 2011).
However, moving objects are generally contiguous,
so additional contour filling strategies are required.
Foreground edges are prone to gaps, which classical
boundary filling techniques (floodfill, scanline fill . . . )
are unable to cope with. The robust filling of contours
with gaps is still a challenge.
Illumination invariant descriptors provide an al-
ternative solution. In the method of Heikkil
¨
a and
Pietik
¨
ainen (Heikkila and Pietikainen, 2006), Local
Binary Patterns (LBPs) are used to construct his-
tograms over a larger region. Different objects usually
have different textures, so they give rise to differing
LBP histograms. However, objects can have regions
with textures similar to the background, which results
in incomplete foreground masks. A recent method,
coined SuBSENSE (St-Charles et al., 2014) attempts
to overcome these issues by using the more advanced
Local Binary Similarity Patterns (Bilodeau et al.,
2013), additional color information, and a framework
for automatic local parameter tuning. Even though
these steps significantly improve the overall perfor-
mance, there are still considerable improvements pos-
sible for difficult illumination conditions. For in-
stance, the F-measure of SuBSENSE on the Night
Video sequences on the ChangeDetection.NET 2014
dataset is limited to only 53.9%, while still being the
highest ranking method to date (Wang et al., 2014).
In this paper, we describe a foreground back-
ground segmentation algorithm which attends to the
shortcomings described above. We combine the ben-
efits of edge based approaches with the robustness of
illumination invariant descriptors. Firstly, we assume
that each local image gradient value above noise level
is an indication for the possible presence of an edge.
Combining these features over a larger area increases
the robustness. As we will show in Section 2, this
information can be extracted from the Local Ternary
Pattern (LTP (Tan and Triggs, 2010)) descriptor.
Secondly, even with increased robustness, gaps
can still occur in the contour. We propose a fast
(O(n)), graph search based method that measures
how difficult it is to reach points from the corners of
the image, where the edge pixels serve as obstacles.
369
Allebosch G., Van Hamme D., Deboeverie F., Veelaert P. and Philips W..
Edge based Foreground Background Estimation with Interior/Exterior Classification.
DOI: 10.5220/0005358003690376
In Proceedings of the 10th International Conference on Computer Vision Theory and Applications (VISAPP-2015), pages 369-376
ISBN: 978-989-758-091-8
Copyright
c
2015 SCITEPRESS (Science and Technology Publications, Lda.)