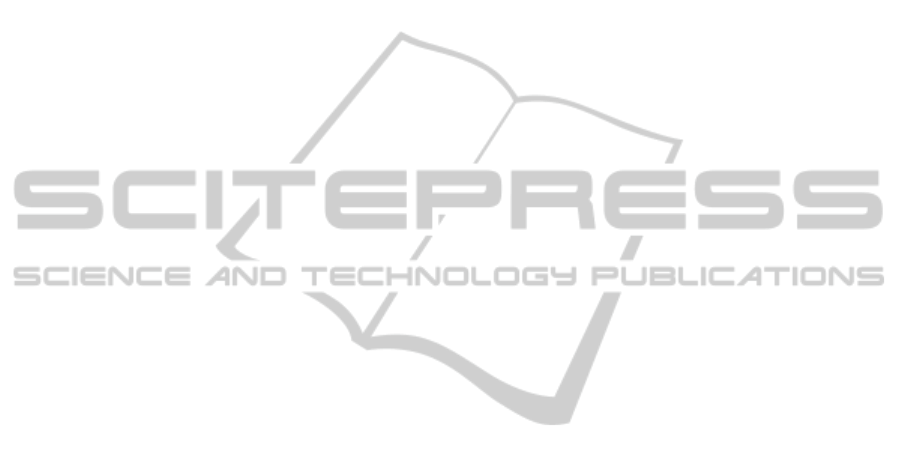
bles of numbers which are too large to comprehend
by university managers. On the other hand, students
have no support for determining which combinations
of subjects are more suitable, specially in their first
academic semester when they have no experience in
what means being an online learner.
In this paper we have described the use of chord
diagrams for visualizing intra-semester enrollment
data, namely the combinations of subjects taken by
students simultaneously. The number of subjects (and
their relationships) visualized as chord diagrams is
determined by a threshold, trying to capture as many
students as possible. We have created diagrams that
include 95% of the students, but they are quite com-
plex, so probably a threshold of 90% is enough for
exploration purposes. Currently now, university man-
agers are using tabular data for detecting programme
bottlenecks; we expect to introduce and evaluate the
use of chord diagrams as a simple way to visualize
such information as part of an internal institutional in-
novation project. These visualizations will be part of
an enrollment support system that will guide students
and their mentors through the large amount of subject
combinations, according to both their personal inter-
ests and background, but taking into account the aca-
demic performance of previous students with similar
enrollment patterns.
Current and future work in this topic should in-
clude the creation of more complex visualizations in-
volving more than two semesters, using concentric
chords or a 3D version, arranging chords in an imag-
inary 3D cone or cylinder. More experimentation for
determining the best number of subjects and the color-
ing scheme is also needed, as well as the information
provided by the interaction with the chord diagram.
ACKNOWLEDGEMENTS
This work is supported by Spanish Ministry of Sci-
ence and Innovation project MAVSEL (ref. TIN2010-
21715-C02-02).
REFERENCES
Bostock, M., Ogievetsky, V., and Heer, J. (2011). D
3
data-
driven documents. IEEE Transactions on Visualiza-
tion and Computer Graphics, 17(12):2301–2309.
Campbell, J., DeBlois, P., and Oblinger, D. (2007). Aca-
demic analytics: A new tool for a new era of educa-
tional research. EUCAUSE Review, 42(4):40–57.
Card, S. K., Mackinlay, J. D., and Shneiderman, B. (1999).
Readings in information visualization - using vision to
think. Academic Press.
Draper, G. M., Livnat, Y., and Riesenfeld, R. F. (2009).
A survey of radial methods for information visualiza-
tion. IEEE Transactions on Visualization and Com-
puter Graphics, 15(5):759–776.
G
´
omez-Aguilar, D. A., Su
´
arez-Guerrero, C., Theron-
S
´
anchez, R., and Garc
´
ıa-Pe
˜
nalvo, F. (2010). Visual
analytics to support e-learning. In Advances in Learn-
ing Processes.
Grau-Valldosera, J. and Minguill
´
on, J. (2014). Rethink-
ing dropout in online higher education: The case of
the universitat oberta de catalunya. The International
Review of Research in Open and Distance Learning,
15(1).
Holten, D. (2006). Hierarchical edge bundles: Visualiza-
tion of adjacency relations in hierarchical data. IEEE
Transactions on Visualization and Computer Graph-
ics, 12(5):741–748.
Kiernan, M., Woodroffe, M., and Thomas, P. (2004). Open
24/7: The journey from e-user to e-learner. In Nall, J.
and Robson, R., editors, Proceedings of World Con-
ference on E-Learning in Corporate, Government,
Healthcare, and Higher Education 2004, pages 95–
97, Washington, DC, USA. AACE.
McCandless, D. (2009). Information is beautiful. Collins,
London.
Park, J.-H. and Choi, H. J. (2009). Factors influencing adult
learners’ decision to drop out or persist in online learn-
ing. Educational Technology & Society, 12(4):207–
217.
Sac
´
ın, C. V., Chue, J., Peche, J. P., Alvarado, G., Vinatea,
B., Estrella, J., and Ortigosa, A. (2011). A data min-
ing approach to guide students through the enrollment
process based on academic performance. User Model-
ing and User-Adapted Interaction, 21(1-2):217–248.
Shneiderman, B. (1996). The eyes have it: A task by
data type taxonomy for information visualizations. In
Proceedings of the IEEE Symposium on Visual Lan-
guages, pages 336–343.
Siraj, F. and Abdoulha, M. A. (2009). Uncovering hid-
den information within university’s student enroll-
ment data using data mining. In Al-Dabass, D., Tri-
weko, R., Susanto, S., and Abraham, A., editors, Asia
International Conference on Modelling and Simula-
tion, pages 413–418. IEEE Computer Society.
Tyler-Smith, K. (2006). Early attrition among first time
elearners: A review of factors that contribute to drop-
out, withdrawal and non-completion rates of adult
learners undertaking elearning programmes. Journal
of Online Learning and Teaching, 2(2).
Yau, N. (2011). Visualize This: The FlowingData Guide to
Design, Visualization, and Statistics. John Wiley &
Sons.
GRAPP2015-InternationalConferenceonComputerGraphicsTheoryandApplications
516