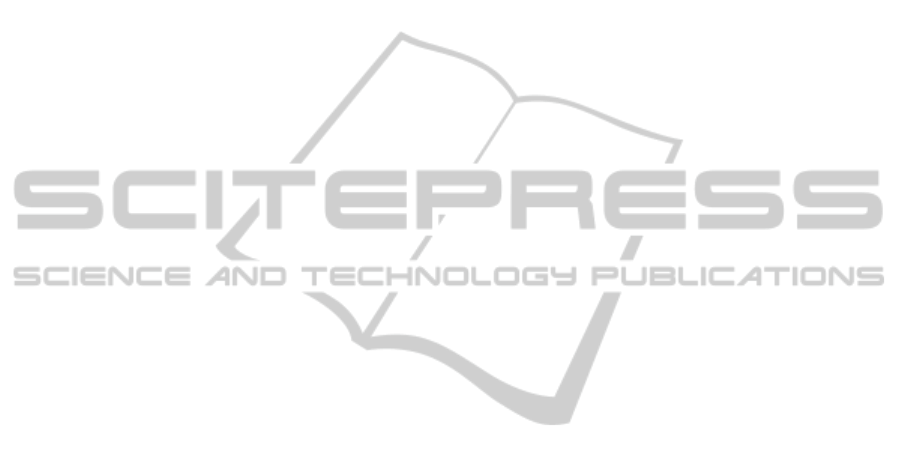
approaches do not need to be tuned for databases of
different image understanding applications, we
believe that a carefully designed system would be a
solution for generalizability. We worked in single
scale in this study and it is a fact that some of the PSL
shape structures such as circulars or ellipticals, or the
star shape generated by
and
could only be met
explicitly at some particular scales. Hence, as a future
work, we intend to examine the method in multiscale.
Moreover, we plan to extend the dictionary by (i)
judiciously quantizing the shape parameter space to
generate the shape varieties, (ii) deriving new shape
classes by various combinations of the current shape
classes that would be filtered by a feature selection
method. Finally, we need to consider the localization
of the descriptors on the image in a more accurate
coding scheme. SymPaD will also be examined in
various databases as a future work.
REFERENCES
Marr, D., 1982. Vision: A computational investigation into
the human representation and processing of visual
information, Henry Holt and Co. Inc., New York, NY,
2-46.
Koenderink, J. J., 1984. The structure of images. Biological
cybernetics, 50(5), 363-370.
Griffin, L. D., 2007. The second order local-image-
structure solid. In IEEE Transactions on Pattern
Analysis and Machine Intelligence, 29(8), 1355-1366.
Lowe, D. G., 1999. Object recognition from local scale-
invariant features. In 7th IEEE international conference
on Computer vision, Vol. 2, pp. 1150-1157.
Dalal, N., and Triggs, B., 2005. Histograms of oriented
gradients for human detection. In IEEE Computer
Society Conference on Computer Vision and Pattern
Recognition, (Vol. 1, pp. 886-893).
Csurka, G., Dance, C., Fan, L., Willamowski, J., and Bray,
C., 2004. Visual categorization with bags of keypoints.
In Workshop on statistical learning in computer vision,
ECCV (Vol. 1, No. 1-22, pp. 1-2).
Jurie, F., and Triggs, B., 2005. Creating efficient codebooks
for visual recognition, In 10th IEEE International
Conference on Computer Vision, (1), 604-610.
Crosier, M., and Griffin, L. D., 2010. Using basic image
features for texture classification. International Journal
of Computer Vision, 88(3), 447-460.
Griffin, L. D., and Lillholm, M., 2007. Feature category
systems for 2nd order local image structure induced by
natural image statistics and otherwise. In IS&T/SPIE
Electronic Imaging, 6492(09). International Society for
Optics and Photonics.
Lillholm, M., and Griffin, L. D., 2008. Novel image feature
alphabets for object recognition. In ICPR (pp. 1-4).
Newell, A. J., and Griffin, L. D., 2014. Writer identification
using oriented Basic Image Features and the Delta
encoding. Pattern Recognition, 47(6), 2255-2265.
Aslan, S., Akgül, C. B., and Sankur, B., 2014. Symbolic
feature detection for image understanding. In
IS&T/SPIE Electronic Imaging (pp. 902406-902406).
International Society for Optics and Photonics.
LeCun, Y. A., Bottou, L., Orr, G. B., and Müller, K. R.,
2012. Efficient backprop. In Neural networks: Tricks of
the trade (pp. 9-48). Springer Berlin Heidelberg.
Griffin, L. D., Lillholm, M., and Nielsen, M., 2004. Natural
image profiles are most likely to be step edges. Vision
Research, 44(4), 407-421.
Calonder, M., Lepetit, V., Strecha, C., and Fua, P., 2010.
Brief: Binary robust independent elementary features.
In ECCV 2010 (pp. 778-792).
Muja, M., and Lowe, D. G., 2012. Fast matching of binary
features. In 9th IEEE Conference on Computer and
Robot Vision, pp. 404-410.
Law, Marc T., Nicolas Thome, and Matthieu Cord, 2014.
Bag-of-Words Image Representation: Key Ideas and
Further Insight, Fusion in Computer Vision. Springer
International Publishing, 29-52.
Shekar, B. H., Holla, K. R., and Kumari, M. S., 2013. KID:
Kirsch Directional Features Based Image Descriptor. In
Pattern Recognition and Machine Intelligence (pp. 327-
334). Springer Berlin Heidelberg.
SymPaD:SymbolicPatchDescriptor
271