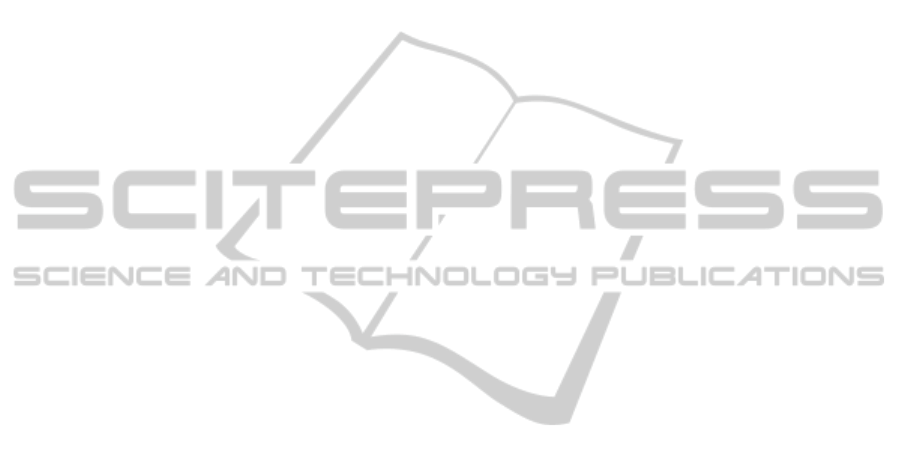
7 CONCLUSIONS
The problem of computer-assisted attribution of
fine-art paintings based on image analysis methods
is considered. A feature description of artistic
brushwork based on textural characteristics of
paintings is proposed. Selected image features in the
form of orientation angle distributions give
quantitative description of painter's artistic style and
provide suitable accuracy of features computation.
Feature evaluation does not require segmentation of
a single brushstroke and is not sensitive to image
acquisition conditions as opposed to the
conventional techniques. The results of computing
experiments showed the efficiency of the proposed
features for comparing artistic styles. The proposed
feature set may be used as a part of technological
description of fine art paintings for restoration and
attribution. The future research will be aimed at the
problems, remained untouched in the current
research. Firstly, as the art experts pay attention to
the geometry of the brushes used by the artists, the
research will be aimed on the frequency analysis of
the images. Secondly, the results of the experiment
presented above, showed that different values of the
feature extraction procedures provide capturing
different components of the painting's texture. So,
another aim of the research will be concerned with
multiscale feature description of paintings. Next, in
the presented research we tested the proposed
feature space and procedures on a limited number of
paintings. In particular, we invoked in experiments
only three paintings created by the same artist (F.
Rokotov) and eight portraits by other artists. In order
to obtain more accurate experimental results, we are
planning to extend our image dataset. It is important
to represent each artist in the dataset by a few
artworks. And at last, it is necessary to develop a
procedure for making decisions on similarity of
brushwork techniques and artistic styles.
ACKNOWLEDGEMENTS
This work is supported by the RFBR grants No 12-
07-00668 and No 15-07-09324.
REFERENCES
Obukhov, G., 1959. A brief glossary of fine art. Moscow,
The soviet painter. (In Russian).
Stone, D., Stork, D., 2010. Computer-assisted
Connoisseurship: The Interdisciplinary Science of
Computer Vision and Image Analysis in the Study of
Art, IP4AI3, Museum of Modern Art, New York, May
27, pp. 9-10.
Morelli, G., 1900. Italian Painters. Critical Studies of
Their Works. John Murray, London.
Berenson. B., 1903. The Study and Criticism of Italian Art.
George Bell and sons, London.
Ignatova, N, 1994. Analysis of oil painting textures. In
Fundamentals of Oil painting Examination. The
guidelines, Moscow, Grabar restoration Centre, vol. 1.
Johnson, C. R., Hendriks, E., Berezhnoy, I.J., Brevdo, E.,
Hughes, S.M., Daubechies, I., Li, J., Postma, E.,
Wang, J.Z., 2008. Image processing for artist
identification (Computerized analysis of Vincent van
Gogh's painting brushstrokes). Signal Processing
Magazine, IEEE. Vol. 25, No 4. - P. 37-48.
Polatkan, G., Jafarpour, S., Brasoveanu, A., Hughes, S.,
Daubechies, I., 2009. Detection of forgery in paintings
using supervised learning. ICIP2009, IEEE. P. 2921-
2924.
Lettner, M., M. Sablatnig, M., 2005. Texture Analysis for
Stroke Classification in Infrared Reflectogramms. H.
Kalviainen et al. Eds., SCIA 2005, Springer-Verlag
Berlin-Heidelberg, LNCS. Vol. 3540. - P. 459-469.
Shahram, M., Stork, D.G., Donoho, D., 2008. Recovering
layers of brush strokes through statistical analysis of
color and shape: an application to van Gogh's Self
portrait with grey felt hat. Computer Image Analysis in
the Study of Art. D. Stork, J. Coddington, Eds.: Proc.
of the SPIE. Vol. 6810. - P. 68100D-1 - 68100D-8.
Li, J., Yao, L., Hendriks, E., Wang, J. Z., 2012. Rhythmic
brushstrokes distinguish van Gogh from his
contemporaries: findings via automated brushstroke
extraction. IEEE TPAMI. Vol. 34, No 6. - P. 1159-
1176.
Sablatnig, R., Kammerer, P., Zolda, E., 1998. Structural
Analysis of Paintings Based on Brush Strokes. Proc.
of SPIE Scientic Detection of Fakery in Art. SPIE.
Vol.. 3315. – P. 87–98.
Murashov, D., 2014. Localization of differences between
multimodal images on the basis of an information-
theoretical measure, Pattern Recognition and Image
Analysis. Springer, Vol. 24, No 1. - P. 133-143.
Escolano, F., Suau, P., Bonev, B., 2009. Information
Theory in Computer Vision and Pattern Recognition. –
London, Springer Verlag.
Eberly, D., 1996. Ridges in Image and Data Analysis.
Klewer Academic Publishers.
Lindeberg, T., 1994. Scale-space Theory in Computer
Vision. Kluwer Academic Publishers.
Jähne, B. 2005. Digital Image Processing. 6th ed.
Springer.
VISAPP2015-InternationalConferenceonComputerVisionTheoryandApplications
226