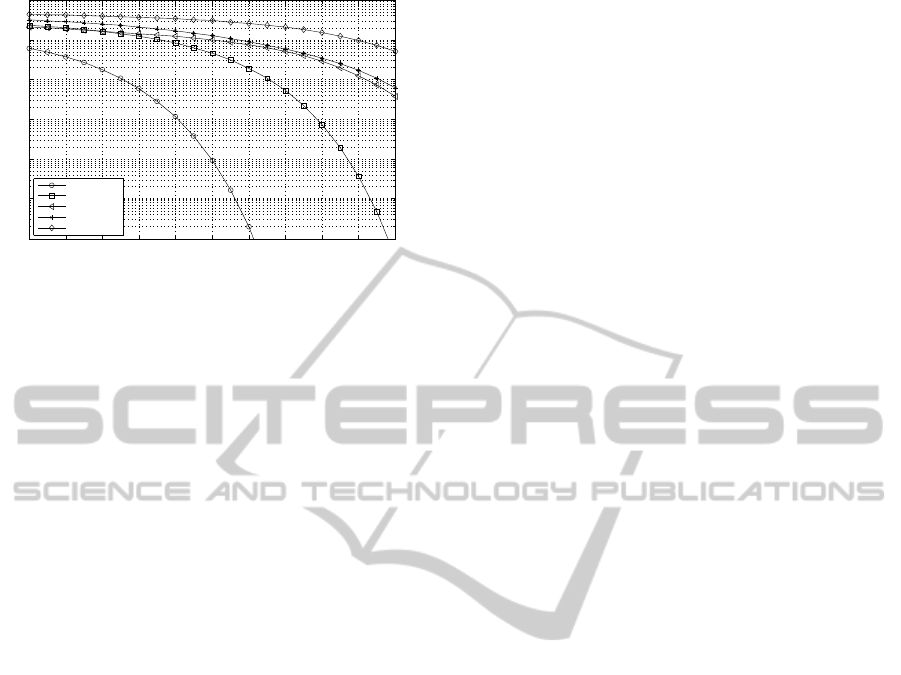
0 2 4 6 8 10 12 14 16 18 20
10
−6
10
−5
10
−4
10
−3
10
−2
10
−1
10
0
SNR (dB)
BER
BER for correlated GMD−assisted MIMO with various active layers (1 to 4), ρ=0.6
TM 256−0−0−0
TM 16−16−0−0
TM 64−4−0−0
TM 16−4−4−0
TM 4−4−4−4
Figure 16: BER comparison for a GMD-assisted MIMO
system with various active layers and a Tx/Rx correlation
factor ρ=0.6.
PDF and CCDF of the singular values as well as the
BER, comparing with the uncorrelated one. Several
transmission modes have been defined by the acti-
vation of a different number of layers. The analy-
sis takes into consideration various antennas’ correla-
tion indexes to show the robustness of GMD-assisted
MIMO systems against correlation.
The simulations outcomes demonstrate that an-
tennas’ correlation affects the SVD-assisted MIMO
channel performance by decreasing its throughput
and increasing the BER. This behaviour is caused by
the existence of predominant weak and strong lay-
ers with corresponding small and large valued singu-
lar values respectively, which are the particular layer
gain coefficient. In the case of GMD-assisted MIMO
systems the number of active layers leads to differ-
ent conclusions. The PDF and CCDF of the singu-
lar values and their geometric mean seems to be a
proper way to anticipate the SVD-assisted and GMD-
assisted MIMO systems performance.
As shown, for a given number of active lay-
ers, antennas’ correlation significantly spreads the
singular values CCDF curves dropping the overall
channel performance. Nevertheless the geometric
mean CCDF curves don’t significantly change with
correlation. As the separation between the singu-
lar values CCDF curves increases, the overall SVD-
assisted MIMO channel performance drops due to
the poor performance of weak layers (with low val-
ued gain coefficients). Conversely, the geometric
mean CCDF curve for a given number of active layers
doesn’t remarkably change with correlation, conclud-
ing that GMD-assisted MIMO systems are more ro-
bust against antennas’ correlation than SVD–assisted
ones. Then, in general terms GMD-assisted MIMO
systems performs better than SVD-assisted ones, spe-
cially when weak layers exist (particularly in corre-
lated channels).
The activation a different number of layers results
in distinct transmission modes which show different
performances as shown in the results. In order to
minimize the overall BER the same constellation size
as well as the same transmit power per layer should
be used. Although individual layers in GMD-assisted
MIMO systems perform in the same way as the gain
coefficient is the same, the appropriate usage of dif-
ferent constellations per layer can improve the overall
MIMO channel performance.
Activating a larger number of layers takes into ac-
count weak layers. In consequence, due to the low
valued singular values of weak layers the computed
geometric mean diminishes and the GMD-assisted
MIMO system performance drops. This outcome is
much more remarkable as the antennas’ correlation
increases. At the opposite side, activating just one
layer leads to the largest geometric mean value. Nev-
ertheless the best performance is not reached because
a high order constellation is transmitted. An interme-
diate number of active layers seems to be the most
appropriate solution which depends on the particular
correlation index.
REFERENCES
Ahrens, A., Cano-Broncano, F., and Benavente-Peces, C.
(2013). Power distribution for svd-aided mimo trans-
mission with receiver-side antennas correlation. In
10th IASTED International Conference on Signal Pro-
cessing, Pattern Recognition, and Applications (SP-
PRA), Innsbruck (Austria).
Benavente-Peces, C., Cano-Broncano, F., Ahrens, A.,
Ortega-Gonzalez, F., and Pardo-Martin, J. (2013).
Analysis of singular values pdf and ccdf on receiver-
side antennas correlated mimo channels. Electronics
Letters, 49(9):625–627.
Foschini, G. J. and Gans, M. J. (1998). On limits of wire-
less communications in a fading environment when
using multiple antennas. Wireless Personal Commu-
nications, 6:311–335.
Haykin, S. S. (2002). Adaptive Filter Theory. Prentice Hall,
New Jersey.
Janaswamy, R. (2002). Effect of element mutual coupling
on the capacity of fixed length linear arrays. Antennas
and Wireless Propagation Letters, IEEE, 1(1):157–
160.
Jiang, Y., Li, J., and Hager, W. W. (2005). Joint transceiver
design for mimo communications using geometric
mean decomposition. Signal Processing, IEEE Trans-
actions on, 53(10):3791–3803.
Kinjo, S. and Ohno, S. (2013). A tomlinson-harashima pre-
coding for correlated mimo channels. In Intelligent
Signal Processing and Communications Systems (IS-
PACS), 2013 International Symposium on, pages 257–
262.
Antennas'CorrelationInfluenceontheGMD-assistedMIMOChannels
Performance
333