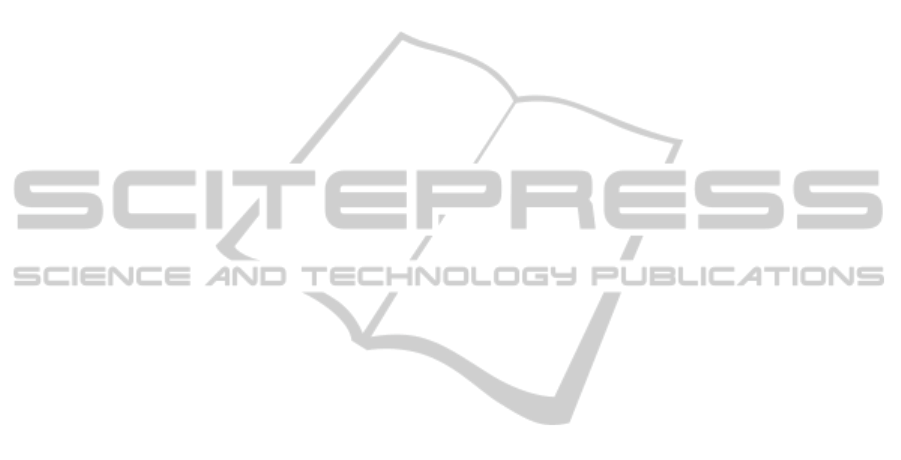
in differential privacy makes it computationally inten-
sive (Dwork, 2006).
One of the pioneer work in personalized privacy
is by Aggrawal & Yu (Aggarwal and Philip, 2008).
They achieved personalized privacy through the use
of k-anonymity by allowing a user to select an integer,
i, (where 1 6i 6 n) to indicate his/her privacy prefer-
ence. This implies that in an anonymised table, T, the
user must be included in a QI-group with at least size
i. A drawback of this is that it might be difficult for
users to set a realistic k-value in real-life especially in
Crime Reporting System where users might be under
duress or shock as a result of the crime. Also, setting
a realistic k-value implies that users must understand
the principle of k-anonymity.
An equally novel approach in achieving personal-
ized anonymisation using the concept of k-anonymity
is the work of Xiao and Tao, 2006. In their work,
an individual specifies the degree of privacy protec-
tion for his/her sensitive values. Their solution as-
sumes that each sensitive attribute has a classifica-
tion tree and each record owner specifies a guarding
node in the tree. Guarding nodes depend on user’s
personal privacy preferences and indicates how users
want their sensitive values to be represented. A ma-
jor drawback of their approach is that a guarding node
requires that a hierarchy-tree be defined on sensitive
attribute. However, hierarchical trees are difficult to
define on numerical and transactional data. Another
drawback is that in real-life, it is unclear how indi-
vidual record owners would set their guarding node
(Aggarwal and Philip, 2008).
Gedik and Liu, 2008 achieved personalized k-
anonymity by allowing users to specify their preferred
k-anonymityvalue. A setback of this is that users may
need to understand the concept of k-anonymity in or-
der to be able to choose an appropriate k-value which
may not be practical in real-life.
Another research targeted towards including users
privacy preference in k-anonymity is the work of
Kabir and Bertino, 2011. In their approach, they only
considered the privacy level of individuals who do not
care about the disclosure of their details. Their work
did not encompass the personal privacy preference of
individuals who care about their privacy.
We therefore note that the issue of incorporating
users preference to cope with anonymization of data
in a manner that is usable in real-life is yet to be stud-
ied. This study is necessary in order to generate re-
liable anonymized reported crime data for third party
service providers.
5 METHODOLOGY
The user study approach was used in order to deter-
mine factor(s) that influence people’s privacy during
crime report in real-life. We conducted this prelim-
inary survey in the University of Cape Town, South
Africa. Twenty-four participants were recruited to
source user experiences with reporting crimes. The
participants consisted of twenty users who had been
affected by crime before and only four users whom
had never been personally affected by crime. Ques-
tionnaires and face-to-face interviews were used to
gather user’s privacy preference during crime report-
ing. The questionnaire was designed to confirm the
validity of the claims in the research of Xiao and Tao,
2006, and Gedik and Liu, 2008 that users have dif-
ferent privacy levels. In addition we also designed
the questionnaire to confirm the claims of Chuang and
Kuo, 2011 that users find it easier to determine their
privacy level using a three-tier privacy level. Addi-
tionally the questionnaire aimed to gather other fac-
tors such as gender, age and crime level that influ-
ences peoples’ privacy during crime reporting.
A three-tier privacy level preference consists of
low, neutral (medium) and high. It is conceived that
the willingness of an individual to share information
is inversely related to his/her privacy level preference.
Our choice of three-tier is based on the research of
Chuang and Kuo, 2011 that believe users can only
recognize their privacy requirements between three
levels. A high privacy level indicates an extreme pri-
vacy consciousness, whereas a low privacy level de-
picts a lower privacy consciousness. Therefore, neu-
tral privacy level is an intermediate.
5.1 Survey Analysis
Figure 1 and Table 3 illustrate both visual and quan-
titative contents of data collected. Such summary is
necessary to obtain preliminary information about the
relationship among the variables collected. All the
collected survey data comprised of 24 subjects and
eight categorical variables: Sex, Age group, Present
education level/Occupation, Highest education qual-
ification (HEQ), Victim of crime, Crime experienced,
Preferred privacy level (PPL), and Reason for choice
of privacy (RCP). All the subjects interviewed are
postgraduate students whose Occupation and HEQ
are exactly related. For example, a student enrolled
for PhD has a Masters degree as his/her HEQ. With-
out loss of generality, HEQ will consequently be
deleted from the analysis data. Table 3 and Figure 1
provide summaries of the different categories of each
of the variables left.
User-definedPrivacyPreferencesfork-AnonymizationinElectronicCrimeReportingSystemsforDevelopingNations
15