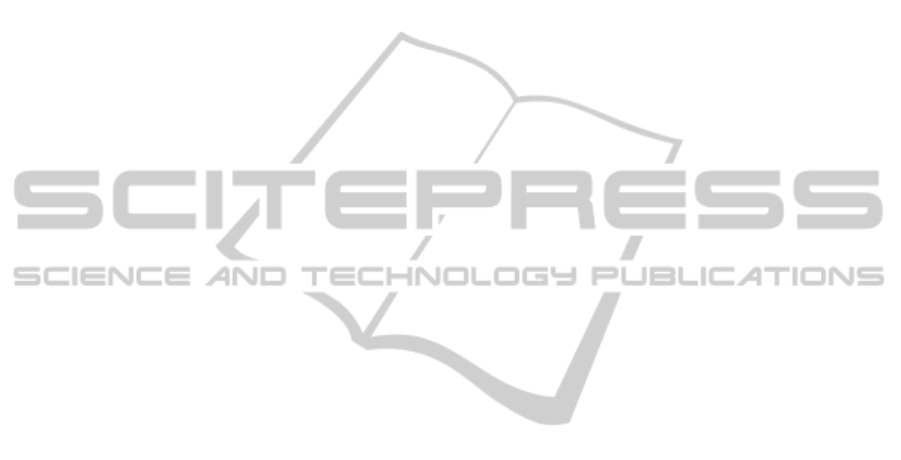
in terms of arrival time. Nevertheless the objective
function might miss some features, but can be rede-
fined as a weighted mean of total monetary cost for
the rider, deviation from the origin-destination trip for
the driver, number of transshipment stops, etc. An-
other way to deal with several features might be the
use of a multi-objective function and the computation
of Pareto optimal solutions.
To conclude, the problem described in this pa-
per can be seen as a new multi-modal transportation
design. The originality of our approach is its abil-
ity to also include a ride-sharing modality, along the
more common pedestrian, cycling, private car or pub-
lic transportations modes.
The particular interest of our work is in making
the service of ride-sharing and public transportation
more flexible and efficient. Specifically, in contrast to
the traditional ride-sharing service, our approach al-
lows to reduce the driver’s detour by using intermedi-
ate pick-up and drop-off locations for the rider, and to
increase the savings for both drivers and riders, com-
pared to the traditional ride-sharing service. The ride-
sharing service can be considered as a complement to
transit for public transportation, i.e. the ride-sharing
will improve transportation service in rural areas, dif-
ficult to serve by public transportation only.
This is the main reason that will incite the public
transport agencies to use ride-sharing to complement
their services. Despite the relative importance of inte-
grating ride-sharing into public transport services, as
far as we know, no previous work exists that allows
to deal with this problem in dynamic and real-time
context. One of the reasons could be the difficulty
to define and combine in real-time the two services:
ride-sharing and public transportation.
ACKNOWLEDGEMENTS
This research was partially funded thanks to the swiss
CTI grant 15229-1 PFES-ES received by the first au-
thor.
REFERENCES
Agatz, N. A. H., Erera, A. L., Savelsbergh, M. W. P., and
Wang, X. (2012). Optimization for dynamic ride-
sharing: A review. European Journal of Operational
Research, 223(2):295–303.
Ambrosino, D. and Sciomachen, A. (2014). An algorith-
mic framework for computing shortest routes in urban
multimodal networks with different criteria. Procedia
- Social and Behavioral Sciences, 108(0):139 – 152.
Operational Research for Development, Sustainability
and Local Economies.
Bast, H., Delling, D., Goldberg, A., M
¨
uller-Hannemann,
M., Pajor, T., Sanders, P., Wagner, D., and Werneck,
R. (2014). Route planning in transportation networks.
MSR-TR-2014-4 8, Microsoft Research.
Berbeglia, G., Cordeau, J., and Laporte, G. (2010). Dy-
namic pickup and delivery problems. European Jour-
nal of Operational Research, 202(1):8–15.
Bit-Monnot, A., Artigues, C., Huguet, M.-J., and Killi-
jian, M.-O. (2013). Carpooling : the 2 synchroniza-
tion points shortest paths problem. In 13th Workshop
on Algorithmic Approaches for Transportation Mod-
elling, Optimization, and Systems (ATMOS), volume
13328, page 12, Sophia Antipolis, France.
Chan, N. D. and Shaheen, S. A. (2012). Ridesharing in
north america: Past, present, and future. Transport
Reviews, 32(1):93–112.
Delling, D., Dibbelt, J., Pajor, T., Wagner, D., and Wer-
neck, R. (2013). Computing multimodal journeys
in practice. In Bonifaci, V., Demetrescu, C., and
Marchetti-Spaccamela, A., editors, Experimental Al-
gorithms, volume 7933 of Lecture Notes in Computer
Science, pages 260–271. Springer Berlin Heidelberg.
Delling, D., Sanders, P., Schultes, D., and Wagner, D.
(2009). Engineering route planning algorithms. In
Algorithmics of large and complex networks, Lecture
notes in computer science. Springer.
Herbawi, W. and Weber, M. (2012). The ridematching prob-
lem with time windows in dynamic ridesharing: A
model and a genetic algorithm. In Proceedings of the
IEEE Congress on Evolutionary Computation, CEC
2012, Brisbane, Australia, June 10-15, 2012, pages
1–8. IEEE.
Liu, L., Yang, J., Mu, H., Li, X., and Wu, F. (2014). Ex-
act algorithms for multi-criteria multi-modal shortest
path with transfer delaying and arriving time-window
in urban transit network. Applied Mathematical Mod-
elling, 38(9-10):2613–2629.
M
¨
uller-Hannemann, M., Schulz, F., Wagner, D., and Zaro-
liagis, C. (2007). Timetable information: Models
and algorithms. In Geraets, F., Kroon, L., Schoebel,
A., Wagner, D., and Zaroliagis, C., editors, Algorith-
mic Methods for Railway Optimization, volume 4359
of Lecture Notes in Computer Science, pages 67–90.
Springer Berlin Heidelberg.
Murueta, P. O. P., Garc
´
ıa, E., and de los Angeles Junco Rey,
M. (2014). Finding in multimodal networks without
timetables. In VEHICULAR 2014 : The Third Interna-
tional Conference on Advances in Vehicular Systems,
Technologies and Applications.
Pyrga, E., Schulz, F., Wagner, D., and Zaroliagis, C.
(2008). Efficient models for timetable information in
public transportation systems. J. Exp. Algorithmics,
12:2.4:1–2.4:39.
ICEIS2015-17thInternationalConferenceonEnterpriseInformationSystems
486