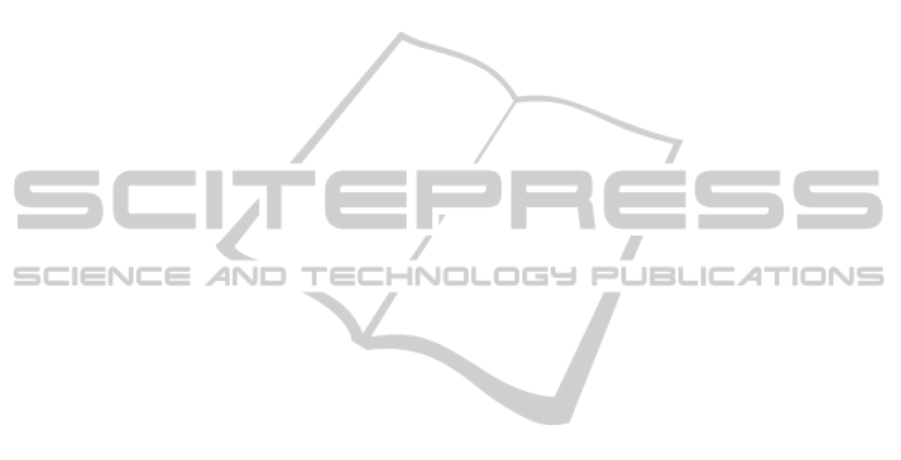
continuous improvement of the recommendations
using these combinations of methods.
Our future research will focus on the
examination and incorporation of additional machine
learning and decision methods specifically targeting
proactive decision support. Moreover, we will
extend our method filtering approach with a
feedback loop, which will support collection of data
about the effectiveness of the recommended
decisions and will utilize the collected data as a basis
for improving the recommendation generation
process. Finally, we will test and evaluate our
approach in real maintenance scenarios in the oil &
gas and automotive industries.
ACKNOWLEDGEMENTS
This work is partly funded by the European
Commission project FP7 STREP ProaSense “The
Proactive Sensing Enterprise” (612329).
REFERENCES
Aissani, N., Beldjilali, B., Trentesaux, D., 2009. Dynamic
scheduling of maintenance tasks in the petroleum
industry: A reinforcement approach. Engineering
Applications of Artificial Intelligence, 22(7), 1089-
1103.
Besnard, F., & Bertling, L., 2010. An approach for
condition-based maintenance optimization applied to
wind turbine blades. Sustainable Energy, IEEE
Transactions on, 1(2), 77-83.
Besnard, F., Patriksson, M., Stromberg, A.,
Wojciechowski, A., Fischer, K., Bertling, L., 2011. A
stochastic model for opportunistic maintenance
planning of offshore wind farms.In PowerTech, IEEE
Trondheim, pp. 1-8.IEEE.
Bouvard, K., Artus, S., Berenguer, C., Cocquempot, V.,
2011. Condition-based dynamic maintenance
operations planning & grouping. Application to
commercial heavy vehicles. Reliability Engineering &
System Safety, 96(6), 601-610.
Castro, I. T., Huynh, K. T., Barros, A., Berenguer, C.,
2012. A predictive maintenance strategy based on
mean residual life for systems subject to competing
failures due to degradation and shocks. In Proc. of the
11th International Probabilistic Safety Assessment and
Management Conference & the Annual European
Safety and Reliability Conference-PSAM 11/ESREL
2012, pp. 375-384.
Chen, X. J., Zhang, Z. G., Tong, Y., 2014. An Improved
ID3 Decision Tree Algorithm. In Advanced Materials
Research, Vol. 962, pp. 2842-2847.
Elwany, A. H., Gebraeel, N. Z., 2008. Sensor-driven
prognostic models for equipment replacement and
spare parts inventory. IIE Transactions, 40(7), 629-
639.
Engel, Y., Etzion, O., Feldman, Z., 2012. A basic model
for proactive event-driven computing. In Proceedings
of the 6th ACM International Conference on
Distributed Event-Based Systems, pp. 107-118.ACM.
Gaddam, S. R., Phoha, V. V., Balagani, K. S., 2007. K-
Means+ ID3: A novel method for supervised anomaly
detection by cascading K-Means clustering and ID3
decision tree learning methods. Knowledge and Data
Engineering, IEEE Transactions on, 19(3), 345-354.
Garg, A., Deshmukh, S. G., 2006. Maintenance
management: literature review and directions. Journal
of Quality in Maintenance Engineering, 12(3), 205-
238.
Huynh, K. T., Barros, A., Berenguer, C., 2012.
Maintenance decision-making for systems operating
under indirect condition monitoring: value of online
information and impact of measurement uncertainty.
Reliability, IEEE Transactions on, 61(2), 410-425.
Ivy, J. S., Nembhard, H. B., 2005. A modeling approach to
maintenance decisions using statistical quality control
and optimization. Quality and Reliability Engineering
International, 21(4), 355-366.
Jardine, A. K., Lin, D., Banjevic, D., 2006. A review on
machinery diagnostics and prognostics implementing
condition-based maintenance. Mechanical systems and
signal processing, 20(7), 1483-1510.
Jin, C., De-lin, L., Fen-xiang, M., 2009. An improved ID3
decision tree algorithm. In Computer Science &
Education. ICCSE'09. 4th International Conference
on, pp. 127-130. IEEE.
Kaiser, K. A., Gebraeel, N. Z., 2009. Predictive
maintenance management using sensor-based
degradation models. Systems, Man and Cybernetics,
Part A: Systems and Humans, IEEE Transactions on,
39(4), 840-849.
Muller, A., Suhner, M. C., Iung, B., 2007. Maintenance
alternative integration to prognosis process
engineering. Journal of Quality in Maintenance
Engineering, 13(2), 198-211.
Pal, M., & Mather, P. M., 2003. An assessment of the
effectiveness of decision tree methods for land cover
classification. Remote sensing of environment, 86(4),
554-565.
Peng, Y., Dong, M., Zuo, M. J., 2010. Current status of
machine prognostics in condition-based maintenance:
a review. The International Journal of Advanced
Manufacturing Technology, 50(1-4), 297-313.
Wu, S. J., Gebraeel, N., Lawley, M. A., Yih, Y., 2007. A
neural network integrated decision support system for
condition-based optimal predictive maintenance
policy. Systems, Man and Cybernetics, Part A:
Systems and Humans, IEEE Transactions on, 37(2),
226-236.
Zikopoulos, P., Eaton, C., 2011. Understanding big data:
Analytics for enterprise class hadoop and streaming
data. McGraw-Hill Osborne Media.
ICEIS2015-17thInternationalConferenceonEnterpriseInformationSystems
494