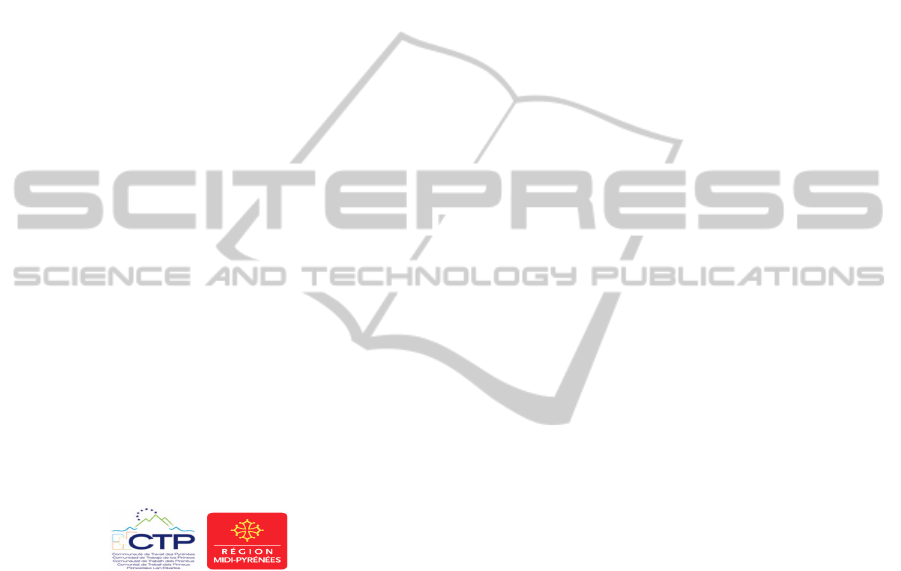
results demonstrate the importance of taking into
account the time criteria (temporal information)
in tag-based user’s profile derivation or even in
other kinds of online social network that are
considered evolving. However, the gain rates of
the time-awareness approach studied in this work
are still low and require more studies to enhance
its effectiveness. Our perspective is to improve
the effectiveness of the time-awareness approach by
study of different time-awareness methods to select
ones that fit the best with each adopted social network.
The popularity of online social networks offers
a variety of available data that are heterogeneous
in terms of structure and utility. To build a
relevant user’s profile from each social network
data, it’s necessary to take into account the data
characteristics in the user profiling process. Our
long-term perspective consists in taking into account
this suggestion in order to find out an effective user’s
interests extraction technique for each type of data.
Finally, we expect to propose a platform that extracts
the information and derives a social dimension of
user’s profile according to the type and the specific
characteristics of each studied social network.
ACKNOWLEDGEMENTS
This presentation was subsidized by the Pyrenean
Working Community and the Region Midi-Pyr
´
en
´
ees
(Toulouse, France).
REFERENCES
Cabanac, G. (2011). Accuracy of inter-researcher
similarity measures based on topical and social clues.
Scientometrics, 87(3):597–620.
Carmel, D., Zwerdling, N., Guy, I., Ofek-Koifman, S.,
Har’el, N., Ronen, I., Uziel, E., Yogev, S., and
Chernov, S. (2009). Personalized social search based
on the user’s social network. In Proceedings of the
18th ACM Conference on Information and Knowledge
Management, CIKM ’09, pages 1227–1236. ACM.
Cazabet, R., Amblard, F., and Hanachi, C. (2010).
Detection of overlapping communities in dynamical
social networks. In 2010 IEEE Second International
Conference on Social Computing (SocialCom), pages
309–314.
Ding, Y., Jacob, E. K., Caverlee, J., Fried, M., and Zhang,
Z. (2009). Profiling social networks: A social tagging
perspective. D-Lib Magazine, 15(3).
Gupta, M., Li, R., Yin, Z., and Han, J. (2010). Survey on
social tagging techniques. SIGKDD Explor. Newsl.,
12(1):58–72.
Ivan, C., Peter, B., and Tsvi, K. (2011). HetRec ’11:
Proceedings of the 2Nd International Workshop
on Information Heterogeneity and Fusion in
Recommender Systems. ACM, New York, NY,
USA.
Kim, H.-N., Alkhaldi, A., El Saddik, A., and Jo,
G.-S. (2011). Collaborative user modeling with
user-generated tags for social recommender systems.
Expert Systems with Applications, 38(7):8488–8496.
Koychev, I. (2009). Gradual forgetting for adaptation
to concept drift. In In Proceedings of ECAI
2000 Workshop Current Issues in Spatio-Temporal
Reasoning, pages 101–106.
Laniado, D. and Mika, P. (2010). Making sense of twitter.
In Patel-Schneider, P. F., Pan, Y., Hitzler, P., Mika,
P., Zhang, L., Pan, J. Z., Horrocks, I., and Glimm,
B., editors, The Semantic Web ISWC 2010, number
6496 in Lecture Notes in Computer Science, pages
470–485. Springer Berlin Heidelberg.
Li, D., Cao, P., Guo, Y., and Lei, M. (2013). Time
weight update model based on the memory principle
in collaborative filtering. Journal of Computers, 8(11).
Maloof, M. A. and Michalski, R. S. (2000). Selecting
examples for partial memory learning. Machine
Learning, 41(1):27–52.
Mezghani, M., P
´
eninou, A., Zayani, C. A., Amous, I.,
and Sedes, F. (2014). Analyzing tagged resources
for social interests detection. In ICEIS 2014 -
Proceedings of the 16th International Conference on
Enterprise Information Systems, Volume 1, Lisbon,
Portugal, 27-30 April, 2014, pages 340–345.
Tchuente, D., Canut, M.-F., Jessel, N., Peninou, A., and
Sdes, F. (2013). A community-based algorithm for
deriving users profiles from egocentrics networks:
experiment on facebook and DBLP. Social Network
Analysis and Mining, 3(3):667–683.
Zheng, N. and Li, Q. (2011). A recommender system
based on tag and time information for social
tagging systems. Expert Systems with Applications,
38(4):4575–4587.
AComparativeStudyofTwoEgocentric-basedUserProfilingAlgorithms-ExperimentinDelicious
639