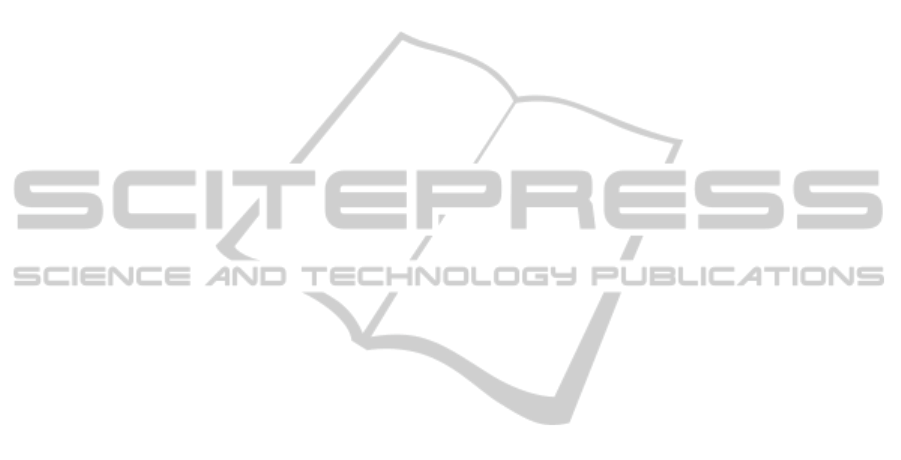
can detect the local minima and maxima and through
them perform deeper analysis of the trends. More spe-
cifically, we can find the intensity of the trend (i.e.
how fast or slow it changes) and the overall perfor-
mance of the trend (i.e., the percentage change from
the minimum to the maximum data point or the rever-
sal). The specific process needs, besides the trend de-
tection, the actual minima and maxima values over
the time series and more calculations on the trends’
data values. Such process will be extensively ana-
lyzed in future work.
REFERENCES
Balkin, S.D., Ord, K., 2000. Automatic Neural Network
Modeling for Univariate Time Series. International
Journal of Forecasting, (1)6,4, pp. 509-515.
Bao, D., 2008. A Generalized Model for Financial Time Se-
ries Representation and Prediction. Applied Intelli-
gence, (29), pp. 1-11, doi: 10.1007/s10489-007-00631.
Bao, D.N., Vy, N.D.K., Anh, D.T., 2013. A hybrid method
for forecasting trend and seasonal time series. 2013
IEEE RIVF International Conference on Computing
and Communication Technologies, Research, Innova-
tion, and Vision for the Future (RIVF), pp.203-208.
Chen, Y.S., Cheng, C.H., Tsai, W.L., 2014. Modeling Fit-
ting-Function-Based Fuzzy Time Series Patterns for
Evolving Stock Index Forecasting. Applied Intelli-
gence, doi: 10.1007/s10489-014-0520-6.
De Gooijer, J.G., Hyndman, R., 2006. 25 Years of Time Se-
ries Forecasting. International Journal of Forecasting,
(22), pp. 443-473.
Edwards, R., Magee, J., Bassetti, W.H.C., 2007. Technical
Analysis of Stocks and Trends. CRC Press. 9
th
edition.
Esling, P., Agon, C., 2012. Time-Series Data Mining. ACM
Computing Surveys (CSUR), (45)1,12.
Gardner, E.S.Jr., McKenzie, E., 1985. Forecasting Trends
in Time Series. Management Science, (31)10, pp. 1237-
1246.
Guerrero, V.M., Galicia-Vasquez, A., 2010. Trend Estima-
tion of Financial Time Series, Applied Stochastic Mod-
els in Business and Industry, (26), pp. 205-223.
Kovalerchuck, B., Vityaev, E., 2000. Data Mining in Fi-
nance: Advances in Relational and Hybrid Methods.
Kluwer Academic Publishers, ISBN 0792378040.
Muhlbauer, A., Spichtinger, P., Lohmann, U., 2009. Appli-
cation and Comparison of Robust Linear Regression
Methods for Trend Estimation. Journal of Applied Me-
teorology and Climatology [1558-8424] Spichtinger,
Peter, (48)9, pp.1961-1970.
Muller, K.R., Smola, J.A., Scholkopf, B., 1997. Prediction
Time Series with Support Vector Machines[C]. In Pro-
ceedings of International Conference on Artificial Neu-
ral Networks, Lausanne, pp. 999-1004.
Pring, M., 2002. Technical Analysis Explained. McGraw-
Hill. New York, NY, 4
th
edition. ISBN 0071226699.
Qin, L.P., Bai, M., 2009. Predicting Trend in Futures Prices
Time Series Using a New Association Rules Algorithm.
16
th
International Conference on Management Science
& Engineering 2009, pp. 1511-1517.
Rasheed, F., Alshalfa M., Alhajj, R., 2010. Efficient Perio-
dicity Mining in Time Series Databases Using Suffix
Trees. IEEE TKDE, (22)20, pp. 1-16.
Selvaratnam, S., Kirley, M., 2006. Predicting Stock Market
Time Series Using Evolutionary Artificial Neural Net-
works with Hurst Exponent Input Windows[C]. Ad-
vances in Artificial Intelligence, pp.617-626.
Xi-Tao, W., 2006. Study on the Application of ARIMA
Model in Time-Bargain Forecast[J]. E-Commerce and
Logistics, 22(15)139-140H.
White, H., Granger, C.W.J., 2011. Consideration of Trends
in Time Series, Journal of Time Series Econometrics,
(3)1,2.
Xylogiannopoulos, K., Karampelas, P., Alhajj, R., 2012.
Periodicity Data Mining in Time Series Using Suffix
Arrays, in Proc. IEEE Intelligent Systems IS’12.
Xylogiannopoulos, K., Karampelas, P., Alhajj, R., 2014.
Exhaustive Patterns Detection In Time Series Using
Suffix Arrays. , manuscript in submission.
Xylogiannopoulos, K., Karampelas, P., Alhajj, R., 2014.
Analyzing Very Large Time Series Using Suffix Ar-
rays, Applied Intelligence, (41)3, pp. 941-955.
Xylogiannopoulos, K., Karampelas, P., Alhajj, R., 2014.
Experimental Analysis on the Normality of pi, e, phi
and square root of 2 Using Advanced Data Mining
Techniques. Experimental Mathematics, (23)2.
Yang, Y., Webb, G., Wu, X., 2005. Data Mining and
Knowledge Discovery Handbook, Chapter 6. Springer.
ICEIS2015-17thInternationalConferenceonEnterpriseInformationSystems
352