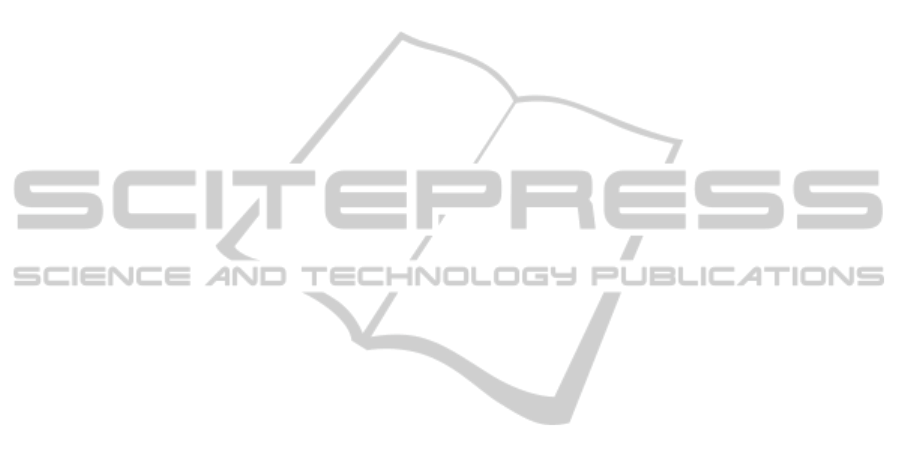
with reinforcement model and without reinforcement
model in real course. Once or twice evaluation
experiment can bring optimized parameters for our
platform.
At last but not least, extending the system for the
users who are not interested in gamification or
reward. There are three kinds of users whom our
existing model can't motivate. They are users who
have a clear learning target, have no interests in our
reward or in gamification. The first kind of users
don't need any extrinsic motivation to arouse their
motivation, they have enough intrinsic motivation.
While the second kind of users need us provide more
kinds of reward to inspire them. We have the close
option for the third kind of users, but that is not
enough. We can try to express our content or
platform with more creative and interesting way or
build some playful learning assistance tool to attract
these users.
REFERENCES
Learn to code. (2015). Retrieved February 22, 2015, from
http://www.codecademy.com/
Tele-TASK: More than video! (2015). Retrieved February
22, 2015, from http://www.tele-task.de/teletask/about/
MIT Game Lab (2013). Retrieved February 22, 2015,
from http://gamelab.mit.edu/event/guest-lecture-scott-
nicholson/
Bauer, M. (2015, February 13). Tele-TASK 2014.
Retrieved February 22, 2015, from
http://prezi.com/pgzubjrfu5nx/tele-task-
2014/?utm_campaign=share&utm_medium=copy.
Flappy Bird. (2014). Retrieved February 22, 2015, from
http://en.wikipedia.org/wiki/Flappy_Bird.
Ethics and Addiction in Games – Develop Conference.
(2012, January 13). Retrieved February 22, 2015, from
http://muslimgamer.com/ethics-addiction-games-
develop-conference/
Cameron, J., & Pierce, W. D. (1994). Reinforcement,
reward, and intrinsic motivation: A meta-analysis.
Review of educational research, 64(3), 363-423.
Deci, E. L., Koestner, R., & Ryan, R. M. (2001). Extrinsic
rewards and intrinsic motivation in education:
Reconsidered once again. Review of educational
research, 71(1), 1-27.
Deterding, S., Dixon, D., Khaled, R., & Nacke, L. (2011).
From game design elements to gamefulness: defining
gamification. Paper presented at the Proceedings of the
15th International Academic MindTrek Conference:
Envisioning Future Media Environments.
Hakulinen, L., Auvinen, T., & Korhonen, A. (2013).
Empirical study on the effect of achievement badges in
TRAKLA2 online learning environment. Paper
presented at the Learning and Teaching in Computing
and Engineering (LaTiCE), 2013.
Hamari, J., Koivisto, J., & Sarsa, H. (2014). Does
gamification work?--a literature review of empirical
studies on gamification. Paper presented at the System
Sciences (HICSS), 2014 47th Hawaii International
Conference on.
Hogarth, R. M., & Villeval, M. C. (2010). Intermittent
reinforcement and the persistence of behavior:
Experimental evidence (Working paper GATE 2010-
18). Retrieved from open archive HAL website:
https://halshs.archives-ouvertes.fr/halshs-
00514125/document.
Iosup, A., & Epema, D. (2013). On the Gamification of a
Graduate Course on Cloud Computing. Paper
presented at the The International Conference for High
Performance Computing, Networking, Storage and
Analysis. IEEE.
Lau, R. W., Yen, N. Y., Li, F., & Wah, B. (2014). Recent
development in multimedia e-learning technologies.
World Wide Web, 17(2), 189-198.
Lucero, A., Karapanos, E., Arrasvuori, J., & Korhonen, H.
(2014). Playful or Gameful?: creating delightful user
experiences. interactions, 21(3), 34-39.
Moritz, F., Siebert, M., & Meinel, C. (2010). Community
rating in the tele-lecturing context. Paper presented at
the Proceedings of the IAENG International
Conference on Internet Computing and Web Services
(ICICWS’10).
Nicholson, S. (2012). A user-centered theoretical
framework for meaningful gamification. Games+
Learning+ Society, 8(1).
Nicholson, S. (2013). Exploring gamification techniques
for classroom management. Paper presented at the
Games+ Learning+ Society 9.0 Conference.
Nicholson, S. (2015). A recipe for meaningful
gamification. Gamification in Education and Business
(pp. 1-20): Springer.
Prensky, M. (2001). Digital natives, digital immigrants
part 1. On the horizon, 9(5), 1-6.
Prensky, M. (2003). Digital game-based learning.
Computers in Entertainment (CIE), 1(1), 21-21.
Schillings, V., & Meinel, C. (2002). tele-TASK:
teleteaching anywhere solution kit. Paper presented at
the Proceedings of the 30th annual ACM SIGUCCS
conference on User services.
Shih, T. K., Squire, K., & Lau, R. W. (2010). Guest
editorial: special section on game-based learning.
IEEE Transactions on Learning Technologies, 3(4),
278-280.
Siebert, M., Moritz, F., Hambach, F., & Meinel, C. (2010).
Enriching E-Learning meta data with user generated
playlists. Paper presented at the Internet Technology
and Secured Transactions (ICITST), 2010
International Conference for.
CSEDU2015-7thInternationalConferenceonComputerSupportedEducation
184