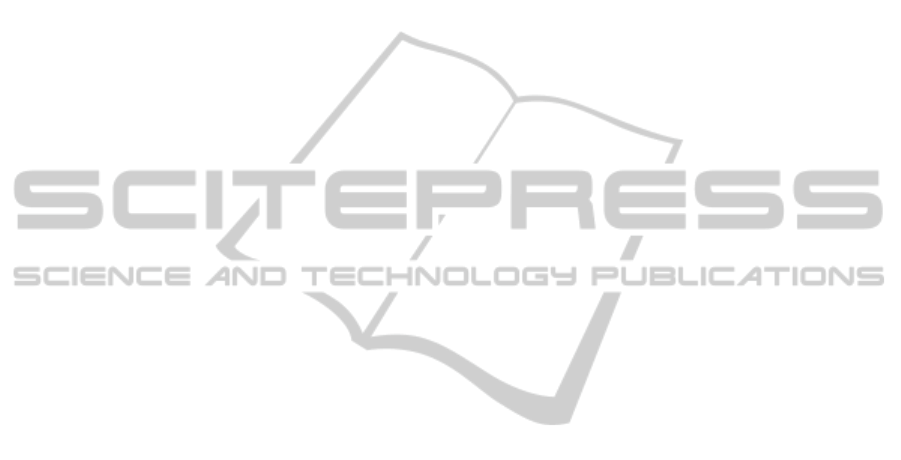
Scenario #2: You are at home, surfing the space of in-
ternet to buy your mom a present for Christmas which
is in two weeks. You are looking for a nice white
woolen sweater, winter-appropriate. Your budget is
unfortunately very limited.
While under pressure and having a reference prod-
uct, almost all participants would either Take an Im-
age of an item or describe it with the Combobox de-
sign. However, without the pressure factor and with
the lack of a reference product (context 2), the num-
ber of favorite votes for the Take or Use Image design
drops down to zero. On the other hand, Combobox in-
creases its advantage, with the Answer Questions de-
sign following. This means that, when asked about fa-
vorites according to context, 81% parted with a 48:33
ratio between participants that changed their favorite
vote for one context scenario and for both.
Looking at the item’s presentation, the ratio in fa-
vor of List and Grid changes. For the first situation,
Grid has slightly more votes (11 vs. 8), while in the
second situation; List has 10 votes and 3 more than
Grid. In the terms of change, 19% of participants did
not change anything, 43% changed at least one, while
38% changed both of their favorites.
Not much changed regarding the overall rating of
the favorite feedback strategy. Replacement is the
participants’ favorite revision option. However, only
a quarter of participants stood up to their previously
rated favorite design: 48% changed in both context
scenarios, and 29% in one of them.
Result 4: Only when under pressure, ’Take or Use a
Picture’ is a very popular preference elicitation strat-
egy among smartphone users according to the results
of our user study. Also the participants’ favorite pre-
sentation interface as well as favorite method to pro-
vide feedback depends on the context situations.
5 CONCLUSIONS AND FUTURE
WORK
This work described an accomplished interaction de-
sign process regarding mobile product recommender
systems using critiquing to elicit the user’s prefer-
ences. We focused on items whose appearance plays
an important role instead of technical features and
therefore selected a mobile clothing recommender
system as application scenario. The process resulted
in developing eleven interaction design alternatives
on an iPhone, categorized into three interaction ac-
tivities: the initial preference elicitation process, the
presentation of the resulting recommendations set and
the preference feedback process. As a result of an ex-
ecuted user study evaluating the implemented interac-
tive designs, we could inter alia show that the Com-
bobox preference elicitation strategy, the List view,
as well as the Replacement feedback strategy are the
most preferred design alternatives of a mobile prod-
uct recommender system concerning ease of use, ade-
quacy and overall satisfaction. The study also showed
that contextual change heavily influences the partic-
ipants’ choice of favorites. Iti developers of mobile
product recommender systems should automatically
obtain the context situation and adapt the interfaces
accordingly. We have to note that the most suitable
interaction technique often depends on the recom-
mendation algorithm, type of users, data, context, de-
vice and recommendation task. However, the results
should be taken into account by developers of mobile
shopping recommender systems using critiquing as
primary feedback strategy. We assume that the result-
ing interaction techniques can be applied to all mobile
scenarios where the item’s appearance matters. We
therefore already started working on a mobile recipe
and restaurant recommender system to find out if our
results can be generalized. We will also evaluate the
most preferred designs in combination, since in a real
system, a user will interact with only one sequence of
the three identified interaction steps. Future develop-
ment also includes the integration of a recommenda-
tion algorithm with a large dataset evaluating the final
design, also with a larger number of evaluators and
different display sizes. Beyond explicit, interesting
will be to examine how an implicit form of feedback
would perform in terms of usability and user experi-
ence.
REFERENCES
Baltrunas, L., Ludwig, B., s. Peer, and Ricci, F. (2012).
Context relevance assessment and exploitation in mo-
bile recommender systems. Personal Ubiquitous
Comput., 16(5):507–526.
Garcin, F., Galle, F., and Faltings, B. (2014). Focal: a per-
sonalized mobile news reader. In Proceedings of the
8th ACM Conference on Recommender systems, pages
369–370. ACM.
Hinze, A. and Buchanan, G. (2005). Context-awareness
in mobile tourist information systems: challenges for
user interaction.
Konstan, J. A. and Riedl, J. (2012). Recommender systems:
from algorithms to user experience. User Modeling
and User-Adapted Interaction, 22(1-2):101–123.
Lamche, B., Trottmann, U., and W
¨
orndl, W. (2014). Active
Learning Strategies for Exploratory Mobile Recom-
mender Systems. In CaRR workshop, ECIR, Amster-
dam.
WEBIST2015-11thInternationalConferenceonWebInformationSystemsandTechnologies
584