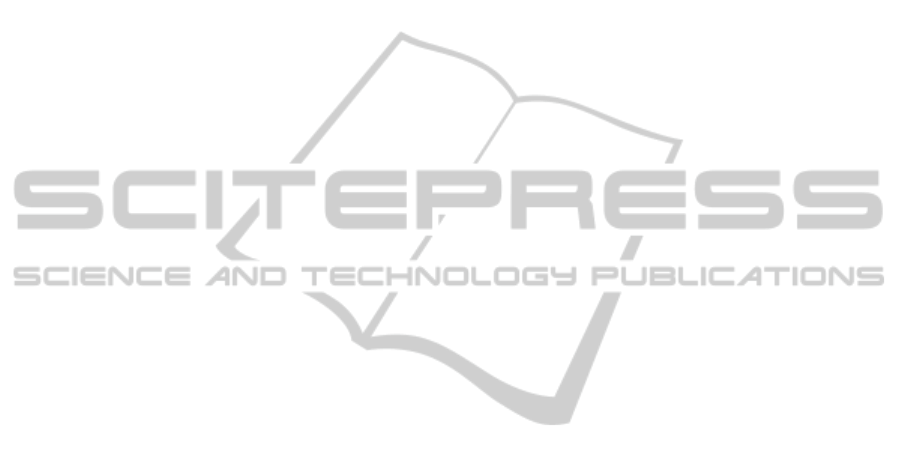
REFERENCES
Bates, T., 2005. Technology, e-learning and distance
education. London, Routledge.
Coffield, F., Moseley, D., Hall, E., & Ecclestone, K., 2004.
Learning styles and pedagogy in post-16 learning: a
systematic and critical review. London, UK, Learning
and Skills Research Centre/University of Newcastle.
Connolly, D., van Harmelen, F., Horrocks, I., McGuinness,
D. L., Patel-Schneider, P. F., & Stein, L. A., 2001.
DAML+OIL (March 2001) Reference Description.
W3C Note 18 December 2001.
Davis, E., 1990. Representations of Commonsense
Knowledge. San Mateo, CA, Morgan Kaufmann.
De Souza e Silva, A., 2006. From Cyber to Hybrid Mobile
Technologies as Interfaces of Hybrid Spaces. Space
and culture, 9(3), 261-278.
Dias, S. B., 2014. Towards an intelligent online learning
environment. A systemic approach. Saarbrücken,
Germany, LAP Lambert Academic Publishing.
Dias, S. B., & Diniz, J. A., 2013. FuzzyQoI model: A fuzzy
logic-based modelling of users’ quality of interaction
with a learning management system under blended
learning. Computers & Education, 69, 38-59.
Dias, S. B., Diniz, J. A., & Hadjileontiadis, L. J., 2014.
Towards an intelligent learning management system
under blended learning: trends, profiles and modelling
perspectives. In J. Kacprzyk, & L. C. Jain (Eds.),
Intelligent Systems Reference Library, Volume 59.
Berlin/Heidelberg, Springer-Verlag,
Doan, A., Madhavan, J., Domingos, P., & Halevy, A., 2002.
Learning to map between ontologies on the semantic
Web. Proc. of the 11th International World Wide Web
conference (pp. 662-673).
Felder, R. M., & Silverman, L. K., 1988. Learning and
teaching styles in engineering education. Engineering
Education, 78(7), 674-681.
Flynn, E., & Siegler, R., 2007. Measuring change: Current
trends and future directions in microgenetic research.
Infant and Child Development, 16(1), 135-149.
Garrison, D. R., & Kanuka, H., 2004. Blended learning:
Uncovering its transformative potential in higher
education. The Internet and Higher Educ., 7, 95-105.
Graf, S., 2007. Adaptivity in learning management systems
focussing on learning styles (Unpublished PhD Thesis),
Vienna Univ. Technology, Vienna, Austria.
Gruber, T. R., 1993. A translation approach to portable
ontologies. Knowledge Acquisition, 5(2), 199-220.
Hadjileontiadou, S. J., Nikolaidou, G. N., Hadjileontiadis,
L. J., & Balafoutas, G. N., 2003. A Fuzzy Logic
Evaluating System to Support Web-based
Collaboration Using Collaborative and Metacognitive
Data, In Proc. of the 3rd IEEE International
Conference on Advanced Learning Technologies
(ICALT’03) (pp. 96-100). Athens, Greece.
Johnson, L., Adams Becker, S., Cummins, M., Estrada, V.,
Freeman, A., & Ludgate, H., 2013. NMC Horizon
Report: 2013 Higher Education Edition. Austin, Texas,
The New Media Consortium.
Johnson, L., Adams Becker, S., Estrada, V., & Freeman, A.,
2015. NMC Horizon Report: 2015 Higher Education
Edition. Austin, Texas, The New Media Consortium.
Jonassen, D. H., & Grabowski, B. L., 1993. Handbook of
individual differences, learning, and instruction.
Hillsdale, New Jersey, Lawrence Erlbaum Associates.
Laskey, B. K., 2008. MEBN: A language for first-order
Bayesian knowledge bases. Artificial Intelligence,
172(2-3), 140-178.
Liu, Y., 2014. Meditations on the semantic net: Oriented
library information service in cloud computing era. In
S. Li, Q. Jin, X. Jiang, & J.J. Park (Eds.) Frontier and
future development of information technology in
medicine and education (pp. 1863-1870). LNEE,
Volume 269 (pp. 1863-1870). Netherlands, Springer.
Lukasiewicz, T., & Straccia, U., 2008. Managing
uncertainty and vagueness in description logics for the
Semantic Web. Web Semantics: Science, Services and
Agents on the World Wide Web, 6(4), 291-308.
Ma, Z., Zhang, F., Yan, L., & Cheng, J., 2014. Fuzzy
Semantic Web Ontology Mapping. In Fuzzy Knowledge
Management for the Semantic Web (pp. 157-180).
Berlin-Heidelberg, Springer.
McGuinness, D. L., Fikes, R., Hendler, J., & Stein, L. A.,
2002. DAML+OIL: An ontology language for the
semantic Web. IEEE Intelligent Syst., 17(5), 72-80.
Mikroyannidis, A., 2012. A semantic framework for cloud
learning environments. In C. Lee (Ed.), Cloud
computing for teaching and learning: strategies for
design and implementation (pp. 17–31). Hershey, PA,
IGI Global.
Oliver, M., & Trigwell, K., 2005. Can ‘blended learning’ be
redeemed?. E-learning and Dig. Media, 2(1), 17-26.
Paquette, G., 2014. Technology-based instructional design:
evolution and major trends. In J. M. Spector, M. D.
Merrill, J. Elen, & M. J. Bishop (Eds.), Handbook of
Research on Educational Communications and
Technology (pp. 661-671). New York, Springer.
Peters, O., 2001. Learning and teaching in distance
education: Analysis and interpretations from an
international perspective. London, Kogan Page.
Reeve, J., & Tseng, C. -M., 2011. Agency as a fourth aspect
of students’ engagement during learning activities.
Contemporary Educational Psychol., 36(4), 257-267.
Van Merriënboer, J. J., Kirschner, P. A., & Kester, L., 2003.
Taking the load off a learner's mind: Instructional
design for complex learning. Educational psychologist,
38(1), 5-13.
Voorsluys, W., Broberg, J., & Buyya, R., 2011.
Introduction to cloud computing. Cloud Comp., 1-41.
Wankel, C., & Blessinger, P., 2013. Increasing student
engagement and retention in e-learning environments
(Web 2.0 and blended learning technologies). Howard
House, Wagon Lane, Bingley, UK, Emerald Group.
Whittaker, J., 2009. Graphical models in applied
multivariate statistics. Hoboken, NJ, Wiley Pub.
Widyantoro, D. H., & Yen, J., 2001. Incorporating Fuzzy
Ontology of Term Relations in a Search Engine. Proc.
of the 1st BISC International Workshop on Fuzzy Logic
and the Internet (pp. 155-160). Berkeley, USA.
CSEDU2015-7thInternationalConferenceonComputerSupportedEducation
194