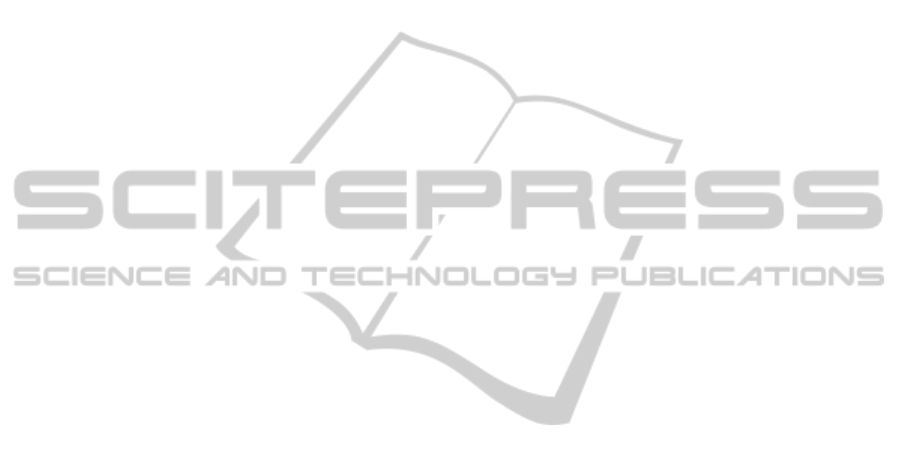
(duration dimension), educational difference (social
distance dimension), applications in common
(services dimension), inbox positive words
(emotional support dimension), and number of
mutual friends (structural dimension). In order to
build the prediction model, they considered data
from the entire relationship, for example “wall
words exchanged” variable counts every message
since the relationship initiated in the social media.
They used linear regression to determine the
variables weights, and they added an extra term to
the equation to take into account the network
structure.
In our approach, we are interested in the online
maintenance of relationships. We would like to
know how a relation is in a given moment: if it is
active or not. One possible benefit is to help people
in keeping friendships alive. The background about
relationship maintenance strategies helped us to
identify potential variables to compose the tie
strength metric. The maintenance strategies include,
for instance, continuity, time, stability, and
satisfaction. The maintenance strategies were used to
investigate 27 Facebook features that are used to
maintain friendships. Identified variables can
represent one or more maintenance strategy, for
example, “number of mutual friends” can be
understood as both stability and network strategy.
We submitted the variables, in a random order, to
the appreciation of ten users, aiming to know which
ones are more relevant to measure the maintenance
of a relationship. The result was variables v
1
to v
5
(see Table 3). For time dependent variables (v
2
to
v
5
), we determine a period of one month, in order to
be able to capture a view of the relationship
maintenance.
The selected variables are present in other social
networks, which makes our approach feasible to be
replicated in other environments. Gilbert and
Karahalios (2009) use variables as “wall intimacy
words”, which need content analysis, so that they
focus on English language. In our approach, the
selected variables do not rely on content analysis, so
that it is possible to compare relations of a person
with two friends using distinct idioms. While Gilbert
and Karahalios (2009) consider data during the
entire relation, we specify a period of analysis. They
retrieved all data and processed offline to calculate
the dimensions’ power. We did offline processing
only to define our variables and weights, but later
we use the metric in online experiments with real
users. The experiments give confidence to the
proposed metric, and they show that the metric was
calculated in a feasible time: users selected friends,
they waited the metric result and later they evaluated
the result.
The focus of our paper is not just to find a metric
to define tie strength, but also to provide an
interpretation to how to maintain online friendships.
Mathematically, linear regression and other types of
regression make sense but they do not provide as
much meaning to the equation they generate.
Basically, the only meaning we can get from the
equation is that it provides the best fit to the test data
set. The key point is that regressions require the
subjective evaluation on the result, i.e. users are
asked to evaluate their relationship with other users
and based on that the regression is calculated. On the
other hand, our approach brings the subjective
evaluation to the weights. The AHP allows us to
bring meaning right away to what is important to
users of social networks. If we find that w
i
= 3*w
j
, it
literally means that most of people believe that v
i
is
more important to define an online friendship than
v
j
.
Arnaboldi et al. (2013) use the same background
as us, which includes the four tie strength
dimensions (time, intimacy, intensity, and reciprocal
services) proposed by Granovetter (1973). In fact,
we complement our background with the dimensions
suggested by Stafford and Canary (1991) and Dindia
and Canary (1993). Arnaboldi et al. (2013) work
with 11 quantitative relational variables. We initiate
our investigation with 27 variables, which include
the 11 variables used by Arnaboldi et al. (2013),
except from “number of days since first
communication” and “number of days since last
communication”. Variables driven from user-filled
fields (such as “educational difference”) were not
considered by Arnaboldi et al. (2013) since the
information depends on cultural aspects and can
even not be provided by users. Instead of eliminate
variables at the beginning, we decided to submit the
27 variables to the appreciation of users, in order to
make them reason about the variables’ importance.
Five quantitative variables were selected, as follows:
v
1
to v
5
(see Table 3). The variable v
1
was not used
by Arnaboldi et al. (2013), however it is presented in
other important works, such as Gilbert and
Karahalios (2009) and Xiang et al. (2010).
Arnaboldi et al. (2013) compare different models
to predict tie strength, including models with
uncorrelated variables and with correlated ones.
They retrieved data from relations of 28 users, who
also evaluated the strength of friendships (using a
scale between 0 and 100). In our work, we asked
users to evaluate the provided tie strength values in
the first experiment, and we observed that it is a
WEBIST2015-11thInternationalConferenceonWebInformationSystemsandTechnologies
318