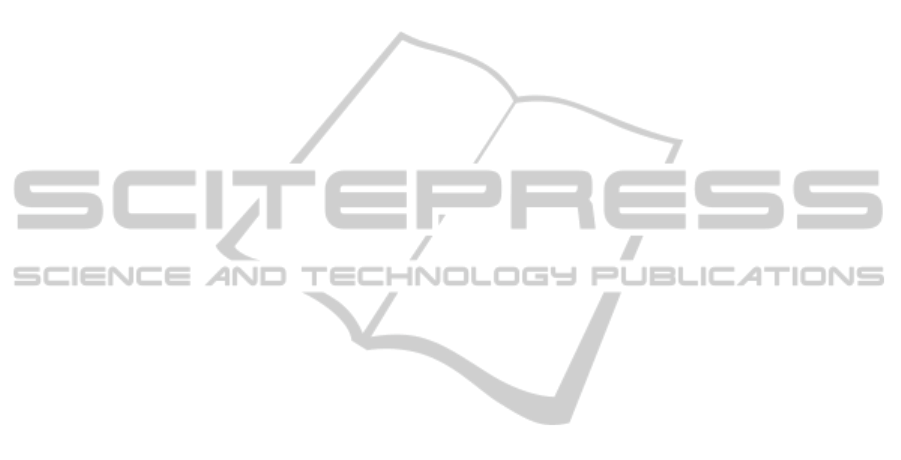
that users typically access the news service with only
one device (either smartphone or tablet) at different
times of the day, but especially in the evening. Sec-
ondly, sufficient training data have to be available to
learn user preferences and variations in these prefer-
ences over different contextual situations. In context-
aware recommender systems, the cold start problem
is strengthened by the fragmentation of the consump-
tion data over different contextual situations. Context
generalization can be a partial solution, but choosing
the right contextual aspect to optimally broaden the
context is often difficult.
ACKNOWLEDGEMENTS
This research was performed in the context of the
iMinds-MIX Stream Store project, which is cofunded
by iMinds, a research institute founded by the Flem-
ish Government.
REFERENCES
Adomavicius, G. and Tuzhilin, A. (2005). Toward the
next generation of recommender systems: a survey
of the state-of-the-art and possible extensions. IEEE
Transactions on Knowledge and Data Engineering,
17(6):734–749.
Adomavicius, G. and Tuzhilin, A. (2008). Tutorial on
context-aware recommender systems. In Proceedings
of the second ACM conference on Recommender Sys-
tems (RecSys ’08).
Adomavicius, G. and Tuzhilin, A. (2011). Context-aware
recommender systems. In Ricci, F., Rokach, L.,
Shapira, B., and Kantor, P. B., editors, Recommender
Systems Handbook, pages 217–253. Springer US.
Baltrunas, L. and Ricci, F. (2009). Context-based splitting
of item ratings in collaborative filtering. In Proceed-
ings of the Third ACM Conference on Recommender
Systems, RecSys ’09, pages 245–248, New York, NY,
USA. ACM.
Cantador, I., Bellog
´
ın, A., and Castells, P. (2008).
News@hand: A semantic web approach to recom-
mending news. In Nejdl, W., Kay, J., Pu, P., and
Herder, E., editors, Adaptive Hypermedia and Adap-
tive Web-Based Systems, volume 5149 of Lecture
Notes in Computer Science, pages 279–283. Springer
Berlin Heidelberg.
De Pessemier, T., Dooms, S., and Martens, L. (2014).
Context-aware recommendations through context and
activity recognition in a mobile environment. Multi-
media Tools and Applications, 72(3):2925–2948.
Følstad, A. (2008). Living labs for innovation and develop-
ment of information and communication technology:
A literature review. Electronic Journal of Organiza-
tional Virtualness, 10:99–131.
Han, B.-J., Rho, S., Jun, S., and Hwang, E. (2010). Music
emotion classification and context-based music rec-
ommendation. Multimedia Tools Appl., 47(3):433–
460.
Hopfgartner, F., Kille, B., Lommatzsch, A., Plumbaum, T.,
Brodt, T., and Heintz, T. (2014). Benchmarking news
recommendations in a living lab. In Kanoulas, E.,
Lupu, M., Clough, P., Sanderson, M., Hall, M., Han-
bury, A., and Toms, E., editors, Information Access
Evaluation. Multilinguality, Multimodality, and Inter-
action, volume 8685 of Lecture Notes in Computer
Science, pages 250–267. Springer International Pub-
lishing.
Kenteris, M., Gavalas, D., and Mpitziopoulos, A. (2010). A
mobile tourism recommender system. In Proceedings
of the The IEEE symposium on Computers and Com-
munications, ISCC ’10, pages 840–845, Washington,
DC, USA. IEEE Computer Society.
Lee, H., Kim, J., and Park, S. (2007). Understanding col-
laborative filtering parameters for personalized rec-
ommendations in e-commerce. Electronic Commerce
Research, 7(3-4):293–314.
Li, L., Wang, D., Li, T., Knox, D., and Padmanabhan,
B. (2011). Scene: A scalable two-stage personal-
ized news recommendation system. In Proceedings of
the 34th International ACM SIGIR Conference on Re-
search and Development in Information Retrieval, SI-
GIR ’11, pages 125–134, New York, NY, USA. ACM.
Papagelis, M., Plexousakis, D., and Kutsuras, T. (2005). Al-
leviating the sparsity problem of collaborative filter-
ing using trust inferences. In Herrmann, P., Issarny,
V., and Shiu, S., editors, Trust Management, volume
3477 of Lecture Notes in Computer Science, pages
224–239. Springer Berlin Heidelberg.
Reuters Institute for the Study of Journalism
(2014). Digital News Report. Available at
http://www.digitalnewsreport.org/.
Ricci, F. (2010). Mobile recommender systems. Informa-
tion Technology & Tourism, 12(3):205–231.
Ricci, F. (2012). Contextualizing recommendations. In
ACM RecSys Workshop on Context-Aware Recom-
mender Systems (CARS ’12), In conjunction with the
6th ACM Conference on Recommender Systems (Rec-
Sys ’12). ACM.
Said, A., Bellog
´
ın, A., and de Vries, A. (2013). News
recommendation in the wild: Cwis recommendation
algorithms in the nrs challenge. In Proceedings of
the 2013 International News Recommender Systems
Workshop and Challenge. NRS, volume 13.
Shani, G. and Gunawardana, A. (2013). Tutorial on
application-oriented evaluation of recommendation
systems. AI Communications, 26(2):225–236.
Telematica Instituut / Novay (2009). Duine Framework.
Available at http://duineframework.org/.
Thomson Reuters (2008-2013). Open Calais. Available at
http://www.opencalais.com/.
Weiss, A. S. (2013). Exploring news apps and location-
based services on the smartphone. Journalism & Mass
Communication Quarterly, 90(3):435–456.
Yu, Z., Zhou, X., Zhang, D., Chin, C.-Y., Wang, X., and
men, J. (2006). Supporting context-aware media rec-
ommendations for smart phones. Pervasive Comput-
ing, IEEE, 5(3):68–75.
TheInformationValueofContextforaMobileNewsService
369