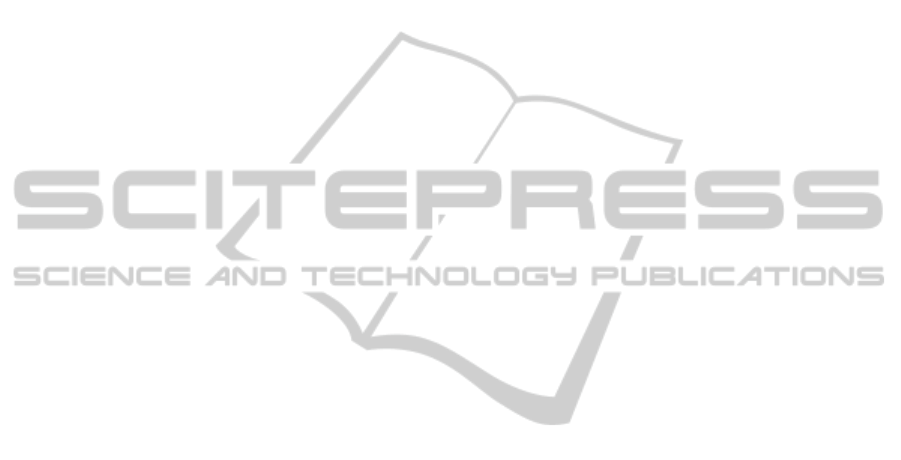
reach a small number of users. We measured the
cascade size and indeed, most of the cascades reach
less than 100 users. Only about 100 cascades in the
data set reach 1000 users, and less than 10 over 10
million users.
Using this measure the most influential users
were those with over 10 million followers, like
Justin Bieber, Louis Tomlinson, and Selena Gomez.
An interesting exception is a user who only had a
few thousand followers, but two of the followers had
many more and the latter’s retweet helped the tweet
to reach millions of further users.
It is for further study, which users were the most
influential among the “average users”, using e.g. the
measure that relates the number of original messages
and retweets to the number of followers of the user.
Mentions could also be calculated, but this is also
for further study.
We also checked how many followers of Justin
Bieber (as of Dec. 2014) could be found in our
original data set. There were about 554000 of them,
i.e. 13 % of 4.15 million. This is slightly less than 1
% of 58 million. We used this subset to calculate
some values for the correction coefficient maximum,
because we have collected the actual follower sets
for those followers of Justin Bieber. In the
calculation we used a subset of about 1.6 billion
rows of the entire table with 7-8 billion rows. For the
point values (exactly 1,2,3, 50,100, 200, 300, 400
followers) pi would be 0.91-0.95, i.e. the overlap is
small. For the range of 200-400 pi drops to 0.76.
For those followers of Justin Bieber who retweeted
his message the coefficient dropped to 0.36 in our
data, meaning a strong overlap in their followers
In the future we will investigate further how
much the rudimentary influence measure we used in
this study overestimates the influence. Another issue
is the passivity of the users. In the current Twitter
user interface it is possible to mute and unmute
another user. It means that once the muted status is
on, the follower is still a follower, but it does not get
the tweets of the muted user. One can argue that a
lot of tweets issuing users might become muted.
ACKNOWLEDGEMENTS
The research of the first two authors was partially
supported by the Academy of Finland grant #268078
"Mining social media sites" (MineSocMed). The
support of University ITMO is also appreciated.
REFERENCES
“About Twitter, Inc. | About.” 2014. Twitter About.
Accessed December 8. https://about.twitter.com/
company.
Bakshy, Eytan, Jake M. Hofman, Winter A. Mason, and
Duncan J. Watts. 2011. “Everyone’s an Influencer:
Quantifying Influence on Twitter.” In Proceedings of
the Fourth ACM International Conference on Web
Search and Data Mining, 65–74. WSDM ’11. New
York, NY, USA: ACM. doi:10.1145/1935826.
1935845.
Bruns, Axel, and Stefan Stieglitz. 2013. “Towards More
Systematic Twitter Analysis: Metrics for Tweeting
Activities.” International Journal of Social Research
Methodology 16 (2): 91–108. doi:10.1080/13645579.
2012.756095.
Cha, Meeyoung, Hamed Haddadi, Fabrıcio Benevenuto,
and Krishna P. Gummadi. 2010. “Measuring User
Influence in Twitter: The Million Follower Fallacy.”
http://www.mpi-
soft.mpg.de/~gummadi/papers/icwsm2010_cha.pdf.
Cheng, Justin, Lada Adamic, P. Alex Dow, Jon Michael
Kleinberg, and Jure Leskovec. 2014. “Can Cascades
Be Predicted?” In Proceedings of the 23rd
International Conference on World Wide Web, 925–
36. WWW ’14. New York, NY, USA: ACM.
doi:10.1145/2566486.2567997.
Da Cruz, Josemar Faustino, and Ronaldo Menezes. 2015.
“Characterizing the Importance of Non-Famous Users
in Information Spread in Social Networks.” Accessed
January 6. http://my.fit.edu/~cruzj2012/wordpress/wp-
content/uploads/2014/11/source.pdf.
Galuba, Wojciech, Karl Aberer, Dipanjan Chakraborty,
Zoran Despotovic, and Wolfgang Kellerer. 2010.
“Outtweeting the Twitterers - Predicting Information
Cascades in Microblogs.” In Proceedings of the 3rd
Wonference on Online Social Networks, 3–3.
WOSN’10. Berkeley, CA, USA: USENIX
Association.
http://dl.acm.org/citation.cfm?id=1863190.1863193.
Hui, Cindy, Yulia Tyshchuk, William A. Wallace, Malik
Magdon-Ismail, and Mark Goldberg. 2012.
“Information Cascades in Social Media in Response to
a Crisis: A Preliminary Model and a Case Study.” In
Proceedings of the 21st International Conference
Companion on World Wide Web, 653–56. WWW ’12
Companion. New York, NY, USA: ACM.
doi:10.1145/2187980.2188173.
Kwon, Joseph, and Ingoo Han. 2013. “Information
Diffusion with Content Crossover in Online Social
Media: An Empirical Analysis of the Social
Transmission Process in Twitter.” In 2013 46th
Hawaii International Conference on System Sciences
(HICSS), 3292–3301. doi:10.1109/HICSS.2013.268.
Romero, Daniel M., Wojciech Galuba, Sitaram Asur, and
Bernardo A. Huberman. 2011. “Influence and
Passivity in Social Media.” In Proceedings of the 20th
International Conference Companion on World Wide
WEBIST2015-11thInternationalConferenceonWebInformationSystemsandTechnologies
496