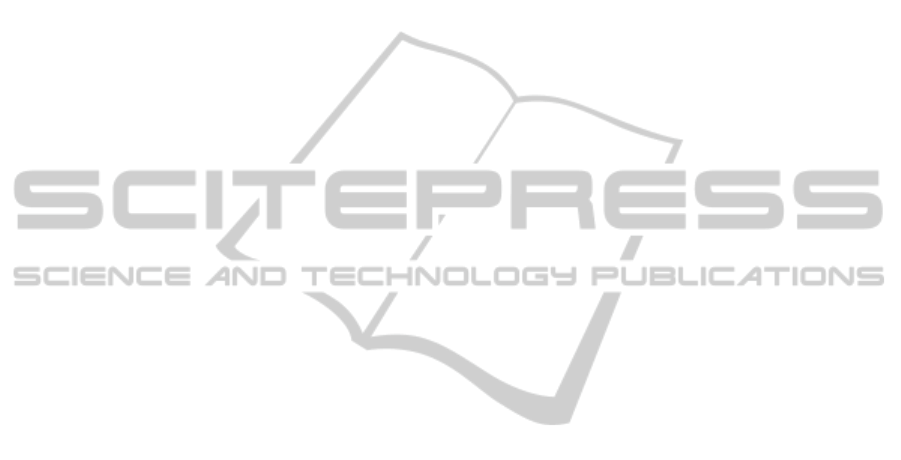
and AbnormalityCRU improve Abnormality;
AbnormalityCRU remains the most accurate measure
for identifying atypical users. Moreover, we can
observe that the quality of AbnormalityCRU is
similar to the one observed with the KNN technique:
75% of users identified as atypical receive recom-
mendations of quality equivalent to the worse 25% of
recommendations from the complete set of users.
In conclusion, we can say that the
AbnormalityCRU measure, which we propose,
is the most accurate measure: when it identifies a
user as atypical, he/she most likely will receive low
quality recommendations. Moreover, this measure
is independent of the recommendation technique:
it is efficient in both KNN and matrix factorization
techniques. This may be linked with the conclusion
made in (Ekstrand, 2014), which has shown that
different recommendation approaches (collaborative
user-user, collaborative item-item, content, etc.) tend
to fail on the same users.
However, although the high accuracy of
AbnormalityCRU , some users (from the com-
plete set) with a high RMSE are identified by none
of the Abnormality measures: it concerns 50% of the
users who have a RMSE greater than 2. This means
that further work has to be conducted to identify the
characteristics of these users.
5 CONCLUSION AND
PERSPECTIVES
Social recommender systems is the context of this
work. Our objective was to identify users who will
receive inaccurate recommendations, upstream of the
recommendation process, i.e. based only on the char-
acteristics of their preferences. We hypothesized that
users with preferences that differ from those of other
users will receive inaccurate recommendations. We
have referred these users to as atypical users. To val-
idate this hypothesis, we proposed several measures
for identifying atypical users, based on the similarity
of users’ preferences with other users, on the average
discrepancy of the ratings they provide in comparison
with the average rating of other users, on the consen-
sus of ratings on resources, or on users rating profile.
We have shown, on a state of the art dataset, that the
measure that uses all these criteria is the most accurate
one and allows to reliably anticipate that a user will
get inaccurate recommendations, with either a KNN
or a matrix factorisation technique.
In a further work, we will focus on the proposition
of a new recommendation approach, to provide atyp-
ical users with high quality recommendations. In par-
allel, it will be interesting to investigate the reasons
why some users do get inaccurate recommendations
and are not identified by any of the measures studied,
as mentionned in the previous section. Specifically, a
user may be atypical on a subset of items, which is
not considered by the measures studied here.
REFERENCES
Adomavicius, G. and Tuzhilin, A. (2005). Toward the next
generation of recommender systems: A survey of the
state-of-the-art. IEEE transactions on knowledge and
data engineering, 17(6):734–749.
Aggarwal, C. (2013). An introduction to outlier analysis. In
Outlier Analysis, pages 1–40. Springer New York.
Bellog
´
ın, A., Castells, P., and Cantador, I. (2011). Predict-
ing the performance of recommender systems: An in-
formation theoretic approach. In Proc. of the Third
Int. Conf. on Advances in Information Retrieval The-
ory, ICTIR’11, pages 27–39. Springer.
Bellog
´
ın, A., Said, A., and de Vries, A. (2014). The magic
barrier of recommender systems no magic, just rat-
ings. In Proc. of the 22nd Conf. on User Modelling,
Adaptation and Personalization (UMAP).
Billsus, D. and Pazzani, M. J. (1998). Learning collabora-
tive information filters. In Proc. of the Fifteenth Int.
Conf. on Machine Learning, ICML ’98, pages 46–54,
San Francisco, CA, USA. Morgan Kaufmann Publish-
ers Inc.
Bobadilla, J., Ortega, F., and Hernando, A. (2012). A col-
laborative filtering similarity measure based on singu-
larities. Inf. Process. Manage., 48(2):204–217.
Bobadilla, J., Ortega, F., Hernando, A., and Guti
´
errez, A.
(2013). Recommender systems survey. Know.-Based
Syst., 46:109–132.
Castagnos, S., Brun, A., and Boyer, A. (2013). When di-
versity is needed... but not expected! In IMMM 2013,
The Third Int. Conf. on Advances in Information Min-
ing and Management.
Del Prete, L. and Capra, L. (2010). differs: A mobile rec-
ommender service. In Proc. of the 2010 Eleventh Int.
Conf. on Mobile Data Management, MDM ’10, pages
21–26, Washington, USA. IEEE Computer Society.
Ekstrand, M. (2014). Towards Recommender Engineering.
Tools and Exp. for Identifying Recommender Differ-
ences. PhD thesis, Faculty of the University of Min-
nesota.
Ekstrand, M. and Riedl, J. (2012). When recommenders
fail: predicting recommender failure for algorithm se-
lection and combination. In Proc. of the sixth ACM
conf. on recommender systems, pages 233–236. ACM.
Ghazanfar, M. and Prugel-Bennett, A. (2011). ”fulfilling the
needs of gray-sheep users in recommender systems, a
clustering solution”. In 2011 Int. Conf. on Informa-
tion Systems and Computational Intelligence. Event
Dates: 18-20, January.
WEBIST2015-11thInternationalConferenceonWebInformationSystemsandTechnologies
388