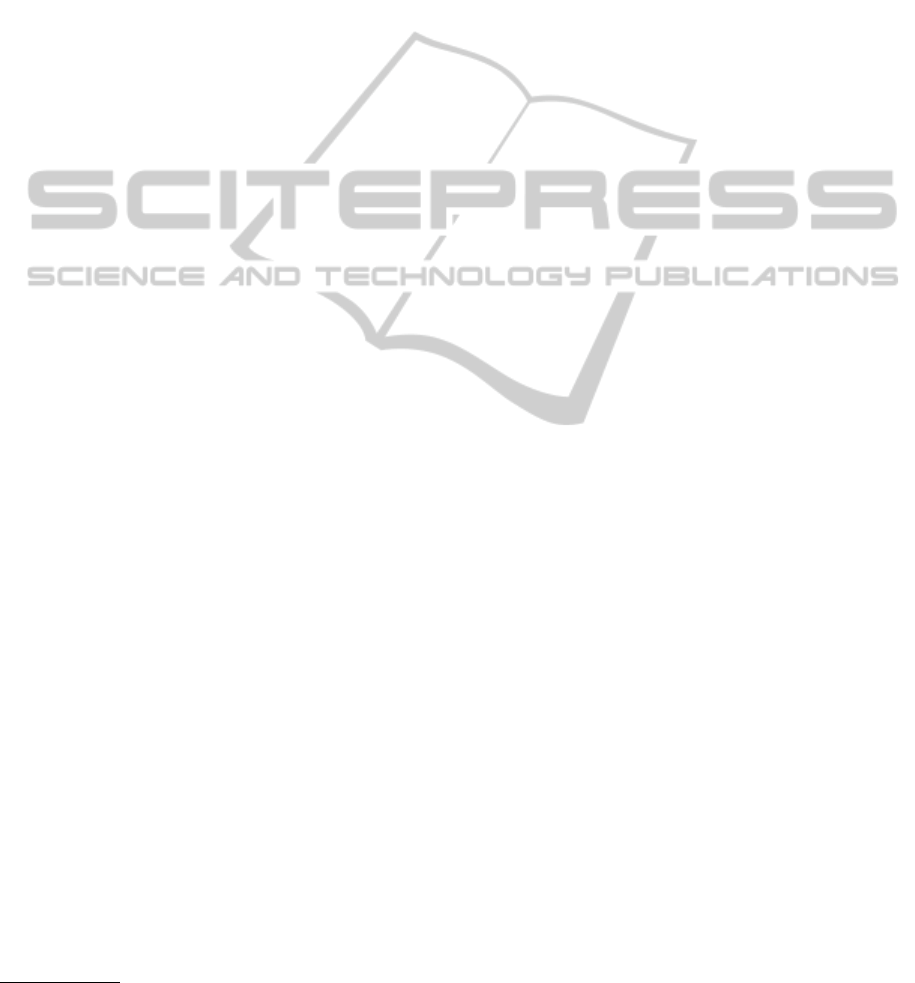
parsing techniques. The feature extraction achieved
98.85% F-measure, which is quite satisfactory.
A study of the features that contributed most to
the success of the classification task was conducted.
For both classifiers, the feature contribution shows the
importance of using more sophisticated, NLP-based
tools in this classification task. Additionally, the com-
plexity of the classification task was shown by the
analysis of the feature value variations between the
different readability levels.
In both scenarios, with five readability levels (A1
to C1) or with three-levels (A, B or C), the classi-
fiers here developed achieved good results with an
accuracy of 75.11% and 81.44%, respectively, and
most of their errors are within one-level distance
from the expected results. For comparative proposes,
the five-levels classifier developed presents good re-
sults against the best classifier of the LX-CEFR sys-
tem (Branco et al., 2014)(section 2), which just got
a maximum accuracy of 30%, while only using the
average number of syllables per word in the classifi-
cation task. For evaluation proposes, the corpus used
in the classifiers here presented is the same used by
LX-CEFR system but with more 112 texts.
The systems here presented has already been
made available to the general public through a web
form
9
and it can easily be extended by adding new
features or metrics of interest to the task at hand. Tak-
ing into account the small size of the corpus anno-
tated according to the readability level in the five-level
scale defined by QuaREPE (Grosso et al., 2011a), it
may prove useful to investigate unsupervised learning
techniques, i.e. techniques that do not depend on a
previously classified corpus, for example, using tech-
niques of cluster analysis, which allows to group a set
of objects into clusters via their similarities.
ACKNOWLEDGEMENTS
This work was supported by national funds through
Fundac¸
˜
ao para a Ci
ˆ
encia e a Tecnologia (FCT) with
reference UID/CEC/50021/2013. The authors grate-
fully acknowledge the use of the corpus classified ac-
cording to the Framework for Teaching Portuguese
Abroad and provided by the Instituto Cam
˜
oes.
REFERENCES
A
¨
ıt-Mokhtar, S., Chanod, J.-P., and Roux, C. (2002).
Robustness Beyond Shallowness: Incremental Deep
9
https://string.l2f.inesc-id.pt/demo/classification.pl
(accessed in March 2015).
Parsing. Natural Language Engineering, 8(3):121–
144.
Baptista, J., Mamede, N., and Gomes, F. (2010). Auxil-
iary Verbs and Verbal Chains in European Portuguese.
In Proceedings of the 9
th
International Conference
on Computational Processing of the Portuguese Lan-
guage (PROPOR’10), pages 110–119, Porto Alegre,
RS, Brazil. Springer.
Beaman, K. (1984). Coordination and Subordination Re-
visited: Syntactic Complexity in Spoken and Writ-
ten Narrative Discourse. In Coherence in Spoken and
Written Discourse, volume 12, pages 45–80. Ablex,
Norwood, NJ.
Bouckaert, R. R., Frank, E., Hall, M., Kirkby, R., Reute-
mann, P., Seewald, A., and Scuse, D. (2013). WEKA
Manual for Version 3-7-11. Hamilton, New Zealand.
Branco, A., Rodrigues, J., Costa, F., Silva, J., and Vaz, R.
(2014). Rolling out Text Categorization for Language
Learning Assessment Supported by Language Tech-
nology. In Proceedings of the 11
th
International Con-
ference on Computational Processing of Portuguese
(PROPOR’14), volume 8775, pages 256–261, S
˜
ao
Carlos, Brazil.
Brown, J. and Eskenazi, M. (2004). Retrieval of Authentic
Documents for Reader-Specific Lexical Practice. In
Proceedings of InSTIL/ICALL Symposium 2004, vol-
ume 17, pages 25–28, Venice, Italy.
Curto, P. (2014). Classificador de textos para o ensino de
portugu
ˆ
es como segunda l
´
ıngua. Master’s thesis, Insti-
tuto Superior T
´
ecnico - Universidade de Lisboa, Lis-
boa.
Figueirinha, P. (2013). Syntactic REAP.PT. Exercises on
Word Formation. Master’s thesis, Instituto Superior
T
´
ecnico - Universidade de Lisboa, Lisboa.
Flesch, R. (1943). Marks of Readable Style: A Study in
Adult Education (Contributions to education). Num-
ber 897. Columbia University, Teachers College, Bu-
reau of Publications, New York, United States.
Fry, E. (1968). A readability formula that saves time. Jour-
nal of Reading, 11(7):513–578.
Fulcher, G. (1997). Text difficulty and accessibility:
Reading formulae and expert judgement. System,
25(4):497–513.
Grosso, M. J., Soares, A., de Sousa, F., and Pascoal,
J. (2011a). QuaREPE - Quadro de Refer
ˆ
encia
para o Ensino de Portugu
ˆ
es no Estrangeiro. Docu-
mento Orientador. Lisboa: Minist
´
erio da Educac¸
˜
ao
e Ci
ˆ
encia/Direc¸
˜
ao Geral de Inovac¸
˜
ao e Desenvolvi-
mento Curricular.
Grosso, M. J., Soares, A., de Sousa, F., and Pascoal, J.
(2011b). QuaREPE - Quadro de Refer
ˆ
encia para o
Ensino de Portugu
ˆ
es no Estrangeiro. Tarefas, Activi-
dades, Exerc
´
ıcios e Recursos para a avaliac¸
˜
ao. Lis-
boa: MEC/DGIDC.
Gunning, R. (1952). The Technique of Clear Writing.
McGraw-Hill, New York, USA.
Gunning, R. (1969). The FOG Index after twenty years.
Journal of Business Communication, 6(2):3–13.
Klare, G. (1963). The measurement of readability. Iowa
State University Press, Ames, USA.
CSEDU2015-7thInternationalConferenceonComputerSupportedEducation
42