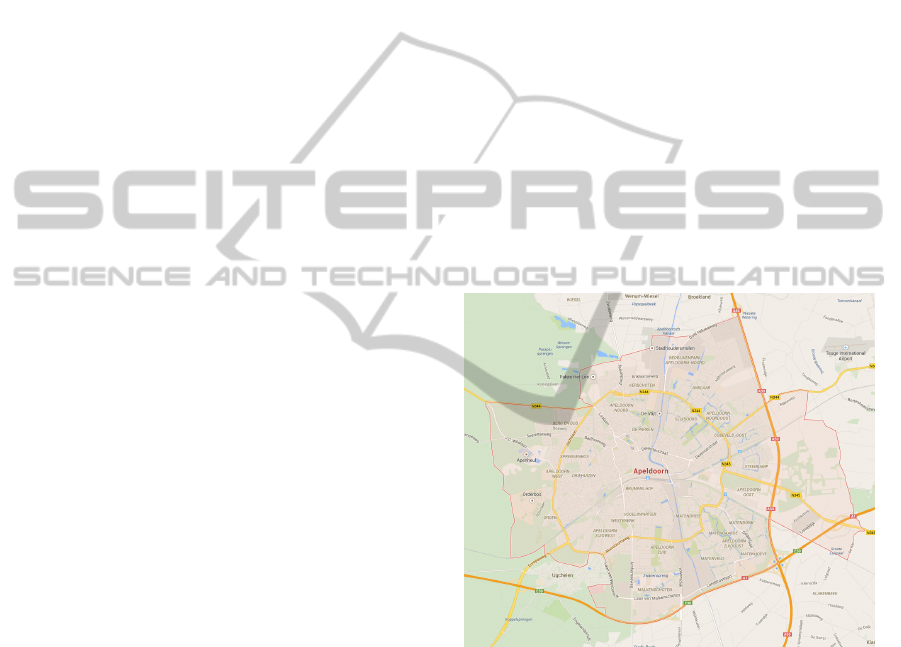
bottom-up assessments that now are possible, using
tools such as “Solar Atlas”, in Dutch Zonatlas
(Zonatlas), which are based on aerial photographs
and solar irradiation. But determining the actual
solar potential of BIPV using high-resolution data
can be very challenging due to the complexity of the
urban areas.
High resolution rooftop potential studies are
relatively new and not much has been done in this
area at rooftop level for estimating the technical and
geographical potential for PV deployment.
(Izquierdo et al. 2008) estimated the technical
potential of roof integrated PV systems using easily
available data and stratified-samples of
Geographical Information Systems (GIS) maps at a
regional level. Based on this work, PV solar energy
potential estimations at municipal to regional level
was conducted in Italy with the help of global solar
radiation maps taken from the Joint Research Centre
of the European Commission (Bergamasco and
Asinari 2011). (Hofierka and Kaňuk 2009) proposed
a methodology for PV potential estimation in urban
areas based on the open-source solar radiation tool
r.sun (developed by (Šúri and Hofierka 2004)) and
3-D city model in GIS. Furthermore, models to
estimate solar potential on building rooftops using
GIS and statistical approaches to create roof-top
solar radiation maps were explored by (Karteris et
al. 2013; Kodysh et al. 2013). (Redweik et al.
2013)developed a model to calculate the solar
energy potential of the buildings taking into account
both the roofs and the facades using high resolution
LiDAR (Laser Imaging Detection And Ranging)
data and applied the model to the campus of
university of Lisbon. However, all the mentioned
studies fall short in estimating the potential at
individual rooftop level.
In the present study, we estimate the rooftop PV
potential in Apeldoorn, a city in the Netherlands
using high resolution LiDAR data and GIS
techniques. Only roof integrated PV is addressed
here. With the use of Solar Analyst (Fu and Paul M
Rich 1999) of ArcGIS solar irradiation over large
geographic areas is computed accounting for
atmospheric effects, sun angle, elevation and effects
of shadows by buildings, elevation and orientation.
Classification of the solar irradiation map was done
to differentiate between optimum and less optimum
suitable sites. These were the basis of potential
estimation, where further energy potential
calculations are made taking into account the slope
and orientation information. These estimations
would help in looking at the trend of PV diffusion,
create business opportunities and additionally
provide an insight for policy implementations.
2 METHODOLOGY
The area chosen for the study was the city of
Apeldoorn (52° 13′ N, 5° 57′ E), in the Gelderland
province of the Netherlands. For locating the
potential PV sites and for calculating the PV
potential a digital elevation model (DEM) derived
from LiDAR data was used. This was obtained from
Actueel Hoogtebestand Nederlands (AHN)
(Nederland 2013). This key input has a resolution of
50 cm (point spacing of 9 points per m
2
, which is
well suited for estimation of solar radiation at
roof-tops. The study area chosen in shown in Figure
1. The city itself is at low elevation, while in the
West one recognizes a hilly region called De
Veluwe. Another important dataset was a vector file
of the footprints of residential buildings in the study
area. In this paper we focus on the residential sector.
The recent building footprint layer was obtained
from Basisregistratie Adressen en Gebouwen
(BAG), which is a part of the government cadaster
system.
Figure 1: City of Apeldoorn which is taken as the study
area in this research.
The estimation of solar potential in this study
was calculated in two steps. First, suitable locations
for roof-top PV were singled out, and then potential
estimation calculations were performed based on
GIS data analysis. We specified some requirements
in order to characterize suitable locations; and
performed all the calculations using ArcGIS.
The criteria chosen for locating suitable PV sites
were solar irradiation, slope and orientation. This
has been adopted from the work of (Chaves and
Bahill 2010). The Area Solar Radiation Tool of the
ArcGIS Spatial Analyst automatically performs the
solar irradiation calculation based on the model by
SMARTGREENS2015-4thInternationalConferenceonSmartCitiesandGreenICTSystems
130