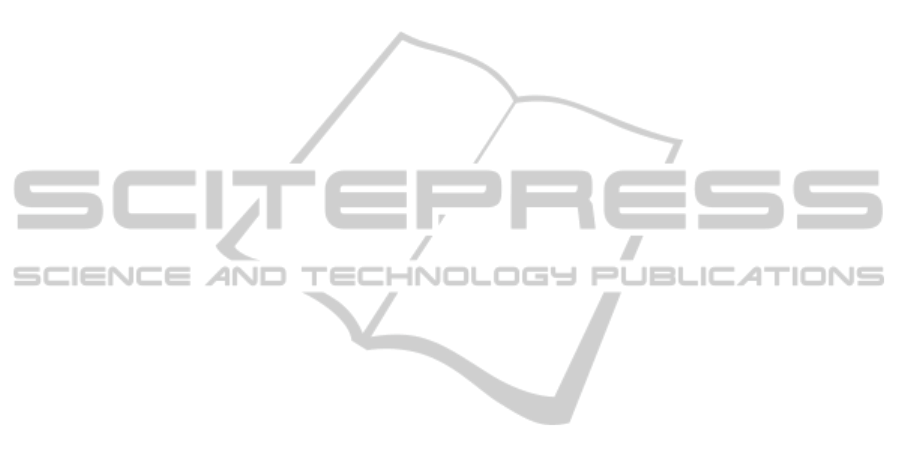
in terms of accurate and reliable documentation.
The validation results let us to conclude that it
is worth to use the entire infrastructure of Big Data
when the analysis include large files or complex tasks,
because it was found that only in those situations the
addition of nodes into the cluster markedly improves
performance.
As future work it is proposed to use cloud comput-
ing to address the problems encountered in the present
project, to evaluate the changes in development ef-
fort, and to promote scalability of BigTexts. Addi-
tionally, another field of work is the development of
more UDFs to increase the catalogue of BigTexts pre-
processing tasks.
REFERENCES
Brinkmann, B. H., Bower, M. R., Stengel, K. A., Wor-
rell, G. A., and Stead, M. (2009). Large-scale elec-
trophysiology: Acquisition, compression, encryption,
and storage of big data. Journal of Neuroscience
Methods, 180(1):185–192.
Capriolo, E., Wampler, D., and Rutherglen, J. (2012). Pro-
gramming Hive. O’Reilly Media, 1 edition edition.
Chawla, N. V. and Davis, D. A. (2013). Bringing big
data to personalized healthcare: A patient-centered
framework. Journal of General Internal Medicine,
28(S3):660–665.
Das, T. and Mohan Kumar, P. (2013). Big data analytics:
A framework for unstructured data analysis. School
of Information Technology and Engineering, VIT Uni-
versity.
Fox, B. (2011). Using big data for big impact. leveraging
data and analytics provides the foundation for rethink-
ing how to impact patient behavior. Health manage-
ment technology, 32(11):16.
Gates, A. (2011). Programming Pig. O’Reilly Media, 1
edition edition.
Lancaster University (2014). What
is stemming? Retrieved from
http://www.comp.lancs.ac.uk/computing/research
stemming/general/.
Liyanage, H., Liaw, S.-T., and de Lusignan, S. (2012). Ac-
celerating the development of an information ecosys-
tem in health care, by stimulating the growth of safe
intermediate processing of health information (IPHI).
Informatics in primary care, 20(2):81–86.
Maheshwari, A. (2015). Data Analytics Made Accessible.
Mayer-Schonberger, V. and Cukier, K. (2013). Big Data: A
Revolution That Will Transform How We Live, Work,
and Think. Houghton Mifflin Harcourt, Boston.
McAfee, A. and Brynjolfsson, E. (2012). Big data: The
management revolution. Harvard business review,
90(10):p60–68.
Meeker, W. Q. and Hong, Y. (2014). Reliability meets big
data: Opportunities and challenges. Quality Engineer-
ing, 26(1):102–116.
Moore, K. D., Eyestone, K., and Coddington, D. C. (2013).
The big deal about big data. Healthcare financial
management: journal of the Healthcare Financial
Management Association, 67(8):60–66, 68. PMID:
23957187.
Purkayastha, S. and Braa, J. (2013). Big data analytics for
developing countries – using the cloud for operational
BI in health. The Electronic Journal of Information
Systems in Developing Countries, 59(0).
Rajaraman, A. and Ullman, J. D. (2012). Mining of mas-
sive datasets. Cambridge University Press, New York,
N.Y.; Cambridge.
Sadalage, P. J. and Fowler, M. (2012). NoSQL Distilled:
A Brief Guide to the Emerging World of Polyglot Per-
sistence. Addison-Wesley Professional, Upper Saddle
River, NJ, 1 edition edition.
Sengupta, P. P. (2013). Intelligent platforms for dis-
ease assessment. JACC: Cardiovascular Imaging,
6(11):1206–1211.
Tablan, V., Roberts, I., Cunningham, H., and Bontcheva,
K. (2012). GATECloud.net: a platform for large-
scale, open-source text processing on the cloud.
Philosophical Transactions of the Royal Society A:
Mathematical, Physical and Engineering Sciences,
371(1983):20120071–20120071.
The Stanford NLP Group (2014a). Coref-
erence resolution. Retrieved from
http://nlp.stanford.edu/projects/coref.shtml.
The Stanford NLP Group (2014b). Stan-
ford CoreNLP. Retrieved from
http://nlp.stanford.edu/software/corenlp.shtml.
The Stanford NLP Group (2014c). Stanford log-
linear part-of-speech tagger. Retrieved from
http://nlp.stanford.edu/software/tagger.shtml.
The Stanford NLP Group (2014d). Stanford named
entity recognizer (NER). Retrieved from
http://nlp.stanford.edu/software/CRF-NER.shtml.
The University of Waikato (2014). Weka 3
- data mining with open source machine
learning software in java. Retrieved from
http://www.cs.waikato.ac.nz/ml/weka/.
White, T. (2012). Hadoop: The Definitive Guide. Yahoo
Press, Beijing, third edition edition edition.
ICT4AgeingWell2015-InternationalConferenceonInformationandCommunicationTechnologiesforAgeingWelland
e-Health
136