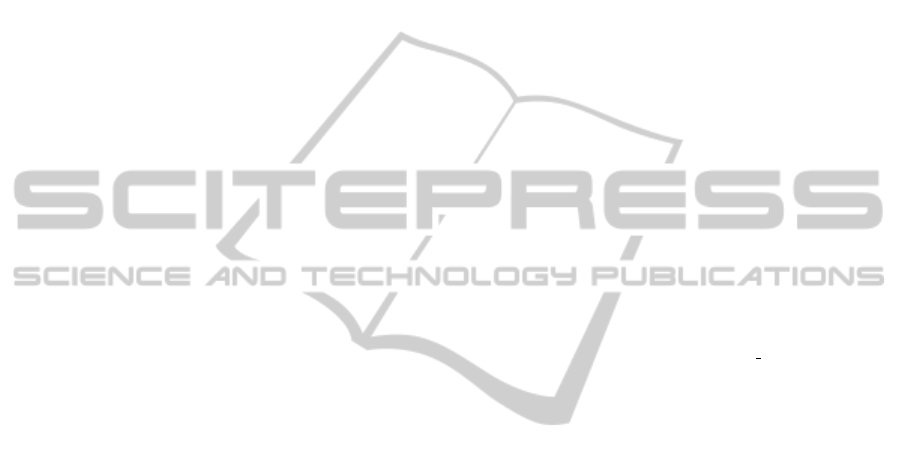
runs up to higher rotational speed meaning that cur-
rent demand decreases (whereby the efficiency factor
increases). A continuously variable load coincides
with continuous changes of the frequency spectrum.
This implies that the averaged values of FC cause a
high similarity of different compared combinations.
In section 4.3, we presented an appropriate feature
(ratios between FC) that is likely to solve this prob-
lem. Because we often switched more than one de-
vice at the same time during the measurement of the
dataset, we are not able to use this feature yet, because
it needs the delta-form (only one device may switch
at the same time).
In comparison with setup 1, we could observe the
same problem in device detection, when there is a
large difference between the load scale of present de-
vices. Variations of the power consumptions of high
load devices can exceed smaller devices power con-
sumption level. Here, the speakers load is as high as
the variation range of power of the mixing machine.
So far, we did not find any feature which could solve
this.
6 SUMMARY AND OUTLOOK
Our developed smart meter prototype, which is cur-
rently in testing phase, is able to provide information
about the electric signal without missing any event
occurring during time of measurement. We pointed
out that with Fourier descriptors, classification with-
out consideration of the voltage signal is possible for
observing state changes of finite state machines. For
specific machines which have a finite state load be-
havior and whose states are of a similar load scale,
this could be a low cost and safe method of supervis-
ing. Like previous research, we observed the problem
of variable loads for the disaggregation algorithms at
setup 2. Therefore, additional extracted features like
ratios between FC could help to identify the existence
of variable loads and will be tested in further setups.
Another approach is to improve transient signal de-
tection, where envelopes of FC during device start-
up and shut-down could be allocated with specific de-
vices. The goal is to make all information contained
in the FC available and combining features extracted
from short time windows and long time windows in
detection algorithms.
ACKNOWLEDGEMENTS
This paper evolved from the research and develop-
ment project “Process Monitoring and Improved En-
ergy Efficiency of Technical Workplaces via Smart
Meters” (PEBiS), which is sponsored by the founda-
tion “Stiftung Rheinland-Pfalz f
¨
ur Innovation” grant
number 961-386261/1048. The contents of this docu-
ment are the sole responsibility of the authors and can
under no circumstances be regarded as reflecting the
position of the foundation “Stiftung Rheinland-Pfalz
f
¨
ur Innovation”.
REFERENCES
Guldner, A., Arns, S., Schunk, T., Gollmer, K.-U., Michels,
R., and Naumann, S. (2013). Detecting consumer de-
vices by applying pattern recognition to smart me-
ter signals. In Page, B., Fleischer, A. G., G
¨
obel, J.,
and Wohlgemuth, V., editors, EnviroInfo2013 - Envi-
ronmental Informatics and Renewable Energies. 27th
International Conference on Informatics for Environ-
mental Protection, Hamburg, September 2-4, 2013,
pages 198–204.
Hart, G. W. (1992). Nonintrusive appliance load monitor-
ing. In Proceedings of the IEEE, Vol. 80, pp. 1870-
1891. IEEE.
H
¨
ock, G. (2009). Dirty Power Oberschwingungen
durch nichtlineare Verbraucher. http://www.gmc-
instruments.ch/src/download/dDirty Power.pdf. [On-
line; accessed 01-December-2014].
Lee, K. D., Norford, L. K., Armstrong, P. R., Holloway, J.,
and Shaw, S. R. (2005). Estimation of variable-speed-
drive power consumption from harmonic content. In
IEEE Transactions on Energy Conversion, Vol. 20, pp.
566-574. IEEE.
Leeb, S. B., Shaw, S. R., and Kirtley, J. L. (1995). Transient
event detection in spectral envelope estimates for non-
intrusive load monitoring. In IEEE Transactions on
Power Delivery, p. 1200, 1995. IEEE.
Liang, J., Ng, S. K. K., Kendall, G., and Cheng, J. W. M.
(2010). Load signature studypart i: Basic concept,
structure, and methodology. In IEEE Transactions on
Power Delivery, Vol. 25, pp. 551-560. IEEE.
Srinivasan, D. and Liew, A. (2006). Neural-network-based
signature recognition for harmonic source identifica-
tion. In IEEE Transactions on Power Delivery, vol.
21, pp. 398-405. IEEE.
Zeifman, M. and Roth, K. (2011). Nonintrusive appliance
load monitoring: Review and outlook. In IEEE Trans-
actions on Consumer Electronics, Vol. 57, pp. 76-84.
IEEE.
Zoha, A., Gluhak, A., Imran, M. A., and Rajasegarar, S.
(2012). Non-intrusive load monitoring approaches for
disaggregated energy sensing: A survey. In Sensors
2012, 12. Sensors - Open Access Journal.
SMARTGREENS2015-4thInternationalConferenceonSmartCitiesandGreenICTSystems
216