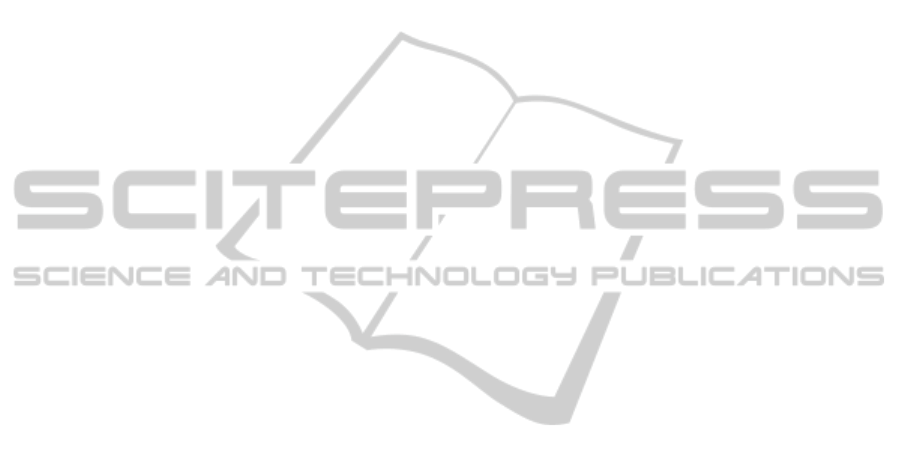
processing phase in every activity recognition system,
being completely independent from that.
As for the future work, we are currently setting-up
new experiments aimed at comparing the hierarchical
approach with a multi-class classifier and with an en-
semble (not hierarchical) of classifiers. Moreover, we
are improving the approach in order to be totally auto-
matic, by using data from Moves as feature instead of
to validate the initial dataset. We are also interested in
studying if we can generalize the proposed approach
to adopt it for all the user of the system, or if we have
to use a personalized approach for each different user
(or a small group of them).
ACKNOWLEDGEMENTS
The research leading to these results has received
funding from the European Community’s, Seventh
Framework Programme FP7/2007-2013, BackHome
project grant agreement n. 288566.
REFERENCES
Carneiro, D., Costa, R., Novais, P., Machado, J., and Neves,
J. (2008). Simulating and monitoring ambient assisted
living. In Proc. ESM.
Casals, E., Cordero, J. A., Dauwalder, S., Fern
´
andez, J. M.,
Sol
`
a, M., Vargiu, E., and Miralles, F. (2014). Ambient
intelligence by atml: Rules in backhome. In Emerging
ideas on Information Filtering and Retrieval. DART
2013: Revised and Invited Papers; C. Lai, A. Giuliani
and G. Semeraro (eds.).
Cook, D. J. (2010). Learning setting-generalized activity
models for smart spaces. IEEE intelligent systems,
2010(99):1.
Cook, D. J. and Das, S. K. (2007). How smart are our en-
vironments? an updated look at the state of the art.
Pervasive and mobile computing, 3(2):53–73.
Corchado, J., Bajo, J., Tapia, D., and Abraham, A. (2010).
Using heterogeneous wireless sensor networks in a
telemonitoring system for healthcare. IEEE Trans-
actions on Information Technology in Biomedicine,
14(2):234–240.
Datar, M., Gionis, A., Indyk, P., and Motwani, R. (2002).
Maintaining stream statistics over sliding windows.
SIAM Journal on Computing, 31(6):1794–1813.
Edlinger, G., Hintermller, C., Halder, S., Vargiu, E., Mi-
ralles, F., Lowish, H., Anderson, N., Martin, S., and
Daly, J. (2015). Brain neural computer interface for
everyday home usage. In HCI International 2015.
Fern
´
andez-Delgado, M., Cernadas, E., Barro, S., and
Amorim, D. (2014). Do we need hundreds of classi-
fiers to solve real world classification problems? The
Journal of Machine Learning Research, 15(1):3133–
3181.
Jafari, R., Encarnacao, A., Zahoory, A., Dabiri, F., Noshadi,
H., and Sarrafzadeh, M. (2005). Wireless sensor net-
works for health monitoring. In Mobile and Ubiqui-
tous Systems: Networking and Services, 2005. Mo-
biQuitous 2005. The Second Annual International
Conference on, pages 479–481. IEEE.
Krishnan, N. C. and Cook, D. J. (2014). Activity recogni-
tion on streaming sensor data. Pervasive and Mobile
Computing, 10:138–154.
Markou, M. and Singh, S. (2003). Novelty detection: a re-
view?part 1: statistical approaches. Signal processing,
83(12):2481–2497.
Meijer, G. A., Westerterp, K. R., Verhoeven, F. M., Koper,
H. B., and ten Hoor, F. (1991). Methods to assess
physical activity with special reference to motion sen-
sors and accelerometers. Biomedical Engineering,
IEEE Transactions on, 38(3):221–229.
Miralles, F., Vargiu, E., Dauwalder, S., Sol
`
a, M., Fern
´
andez,
J., Casals, E., and Cordero, J. (2014). Telemonitoring
and home support in backhome. In Proceedings of the
8th International Workshop on Information Filtering
and Retrieval co-located with XIII AI*IA Symposium
on Artificial Intelligence (AI*IA 2014).
Mitchell, M., Meyers, C., Wang, A., and Tyson, G.
(2011). Contextprovider: Context awareness for med-
ical monitoring applications. In Conf Proc IEEE Eng
Med Biol Soc.
Nait Aicha, A., Englebienne, G., and Kr
¨
ose, B. (2013).
How lonely is your grandma?: detecting the visits to
assisted living elderly from wireless sensor network
data. In Proceedings of the 2013 ACM conference on
Pervasive and ubiquitous computing adjunct publica-
tion, pages 1285–1294. ACM.
Nugent, C. D., Hong, X., Hallberg, J., Finlay, D., and
Synnes, K. (2008). Assessing the impact of indi-
vidual sensor reliability within smart living environ-
ments. In Automation Science and Engineering, 2008.
CASE 2008. IEEE International Conference on, pages
685–690. IEEE.
Ord
´
onez, F. J., de Toledo, P., and Sanchis, A. (2013). Activ-
ity recognition using hybrid generative/discriminative
models on home environments using binary sensors.
Sensors, 13(5):5460–5477.
Pitta, F., Troosters, T., Spruit, M. A., Decramer, M., and
Gosselink, R. (2005). Activity monitoring for as-
sessment of physical activities in daily life in patients
with chronic obstructive pulmonary disease. Archives
of physical medicine and rehabilitation, 86(10):1979–
1985.
Ranganathan, A., Al-Muhtadi, J., and Campbell, R. H.
(2004). Reasoning about uncertain contexts in perva-
sive computing environments. IEEE Pervasive Com-
puting, 3(2):62–70.
Scanaill, C. N., Carew, S., Barralon, P., Noury, N., Lyons,
D., and Lyons, G. M. (2006). A review of approaches
to mobility telemonitoring of the elderly in their liv-
ing environment. Annals of Biomedical Engineering,
34(4):547–563.
Sch
¨
olkopf, B., Platt, J. C., Shawe-Taylor, J., Smola, A. J.,
and Williamson, R. C. (2001). Estimating the support
ImprovingActivityMonitoringThroughaHierarchicalApproach
167