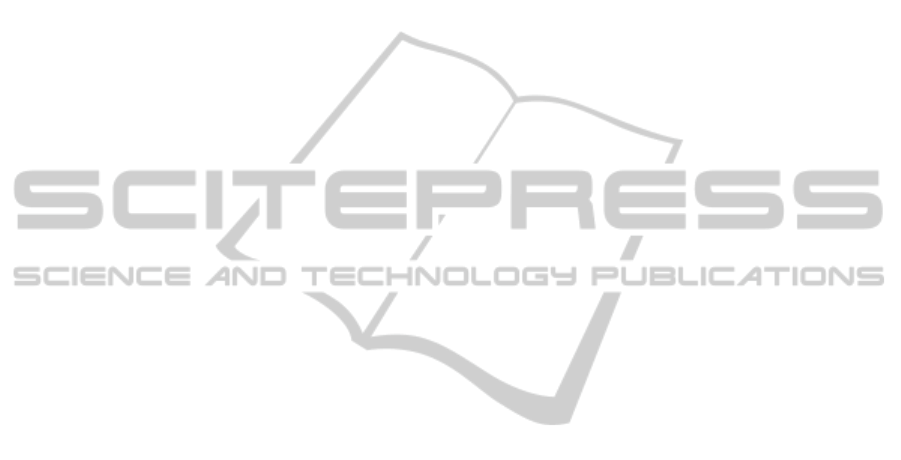
shopping: perspectives of fairness and trust. Online
Information Review, 36(1), pp.104–125.
Choi, B. & Choi, B.-J., 2014. The effects of perceived
service recovery justice on customer affection, loyalty,
and word-of-mouth. European Journal of Marketing,
48(1/2), pp.108–131.
Colquitt, J. A. et al., 2001. Justice at the millennium: a
meta-analytic review of 25 years of organizational
justice research. Journal of Applied Psychology, 86(3),
pp.425–445.
Cropanzano, R., 1993. Justice in the workplace:
approaching fairness in human resource management,
Mahwah, NJ: Lawrence Erlbaum Associates.
Dhar, S., 2012. From outsourcing to cloud computing:
evolution of IT services. Management Research
Review, 35(8), pp.664–675.
Dichter, E., 1966. How word-of-mouth advertising works.
Harvard Business Review, 44(6), pp.147–166.
Eberlin, R. & Tatum, B. C., 2005. Organizational justice
and decision making: when good intentions are not
enough. Management Decision, 43(7/8), p.1040.
Fishbein, M. & Ajzen, I., 1975. Belief, attitude, intention,
and behavior: an introduction to theory and research,
MA: Addison-Wesley.
Folger, R. & Greenberg, J., 1985. Procedural justice: an
interpretive analysis of personnel systems. Research in
Personnel and Human Resources Management, 3,
pp.141–183.
Greenberg, J., 1990. Organizational justice: yesterday,
today, and tomorrow. Journal of Management, 16(2),
pp.399–432.
Greenberg, J., 1993. The social side of fairness:
interpersonal and informational classes of
organizational justice. In R. Cropanzano, ed. Justice in
the Workplace: Approaching Fairness in Human
Resource Management. Hillsdale, NJ: Lawrence
Erlbaum Associates, pp. 79–103.
Hair, J. F. et al., 2009. Multivariate data analysis
Seventh., Englewood Cliffs: Prentice Prentice Hall.
Hocutt, M., Browers, M. & Donavan, D., 2006. The art of
service recovery: fact or fiction. Journal of Service
Marketing, 20, pp.199–207.
Johnston, R., 1995. Service failure and recovery: impact,
attributes and processes. Advance in Services
Marketing and Management: Research and Practice,
4, pp.211–28.
Kassim, E. S. et al., 2012. Information System Acceptance
and User Satisfaction: The Mediating Role of Trust.
International Conference on Asia Pacific Business
Innovation and Technology Management, 57(0),
pp.412–418.
Kau, A.-K. & Loh, E.W.-Y., 2006. The effects of service
recovery on consumer satisfaction: a comparison
between complainants and non-complainants. Journal
of Services Marketing, 20(2), pp.101–111.
Kelly, S. W. & Davis, M. A., 1994. Antecedents to
customer expectations for service recovery. Journal of
the Academy of Marketing Science
, 22(11), pp.52–61.
Kim, T., Yoo, J.-E. & Lee, G., 2012. Post-recovery
customer relationships and customer partnerships in a
restaurant setting. International Journal of
Contemporary Hospitality Management, 24(3),
pp.381–401.
Kuenzel, S. & Katsaris, N., 2009. A critical analysis of
service recovery processes in the hotel industry. TMC
Academic Journal, 4(1), pp.14–24.
Kuo, Y.-F., Yen, S.-T. & Chen, L.-H., 2011. Online
auction service failures in Taiwan: typologies and
recovery strategies. Electronic Commerce Research
and Applications, 10(2), pp.183–193.
Kwok, D., Land, L. & Stephens, G., 2009. Multi-
dimensionality of overall consumer satisfaction –
socio-technical perspective. In Proceedings of the
Fifteenth Americas Conference on Information
Systems. p. 310.
La, K. V. & Kandampully, J., 2004. Market oriented
learning and customer value enhancement through
service recovery management. Managing Service
Quality, 14(5), pp.390–401.
Lawkobkit, M. & Kohsuwan, P., 2012. Focal determinants
of service fairness and service recovery satisfaction in
cloud computing. In Chiang Mai: 17th Asia Pacific
Decision Sciences Institute (APDSI) International
Conference, p. 81.
Lawkobkit, M. & Larpsiri, R., 2014. The focal
determinants of service fairness, satisfaction and
behavioral intentions in service management. APHEIT
Humanities-Social Sciences Journal, 21(1), pp.22–36.
Leventhal, G. S., 1980. What should be done with equity
theory? In K. Gergen, M. Greenberg, & R. Willis, eds.
Social exchange: advances in theory and research.
New York: Plenum Press, pp. 27–55.
Levesque, T. & McDougall, G. H. G., 1996. Determinants
of customer satisfaction in retail banking.
International Journal of Bank Marketing, 14(7),
pp.12–20.
Liu, R. R. & Zhang, W., 2010. Informational influence of
online customer feedback: an empirical study. Journal
of Database Marketing & Customer Strategy
Management, 17(2), pp.120–131.
Masterson, S. S. et al., 2000. Integrating justice and social
exchange: The differing effects of fair procedures and
treatment on work relationships. Academy of
Management Journal, 43(4), pp.738–748.
Maxham, J. G. & Netemeyer, R., 2003. Firms reap what
they sow: the effects of shared values and perceived
organizational justice on customers’ evaluations of
complaint handling. Journal of Marketing, 67(1),
pp.46–62.
Maxham, J. G. & Netemeyer, R., 2002. Modeling
customer perceptions of complaint handling over time:
the effects of perceived justice on satisfaction and
intent. Journal of Retailing, 78, pp.239–252.
McCollough, M. A. & Bhardwaj, S. G., 1992. The
recovery paradox: an examination of consumer
satisfaction in relation to disconfirmation, service
quality and attribution based theory. In Marketing
Theory and Applications. American Marketing
Association, p. 119.
CLOSER2015-5thInternationalConferenceonCloudComputingandServicesScience
274