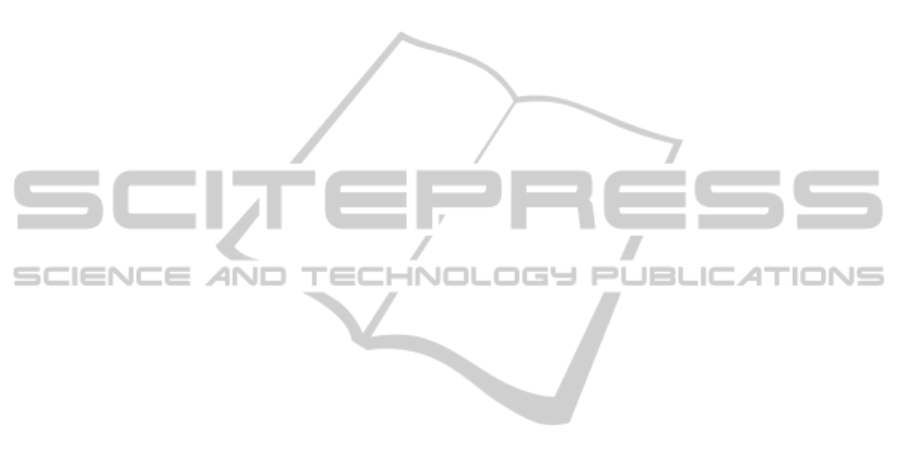
∀C(M) → O(E
S
) = M(qA) ⊂ (M(qB) ∪M(qC)) (1)
From this perspective of thoughts concerning a neural
network in the human brain, each neuron has the exact
same functions, attributes and components for reach-
ing mathematical and mereonymic operability. Addi-
tionally, the life-span (essential and separable cogni-
tive products of a thought as the result of operatios
on neural axon states of created motifs) of the rela-
tionship between first-thought, next-thought (thought
and SubQuantity of thoughts as individual wholes),
and a single motif (neural axon states which turn a
substantial neuron into a particular thought) all corre-
spond to Guizzardi’s description of inseparable parts.
Axon-states and thoughts are essential and seperable;
they are highly dependent on one another other due
to the fact that life-span of individual sub-quantities
of thoughts are wholes in their own right, and thus do
not loose their existence when they loose their whole.
A quantity of thoughts must be as infinitely diversible
(homeomerous) as the neural axon-states (motif) for
the creation of sub-quantities of further though (think-
ing fractals). This is exactly what could be key in the
production of the infinitely diverse intuitive cognitive
products of creativity, which could in turn be the most
important factor in measuring consciousness.
Transitivity Problem. All compositions need to be
transitive as to assure that the operations of the whole
have the correct impact on each of its parts. Transi-
tivity holds true between component functional com-
plexes when the parts share a similar essential part-
hood relationship which also applies to the whole
(cf.) (Guizzardi, 2005, pp. 187). If the relation-
ship between the parts is not the same as that be-
tween the wholes, the applicability of operations to
the whole needs to be validated in order to assure that
the operations between the parts of the whole have
the correct impact. A formalized solution to isolate
the scope of transitivity of functional part-whole re-
lations can be found in (Guizzardi, 2009). However,
all neurons must have the same kind of relationship
within their sub-collections, and all sub-collections
should also share the same type of relation as the
neuron cells share with one another. The ideal situa-
tion would be that of a subQuantityOf and subCollec-
tionOf relationships (corresponding to node-weight
which equals the quantity of connections in neural
networks) (Sporns, 2011, p. 8).
3 CONCLUSION
This paper describes the development of an ontology
with the aim to answer many questions for conscious-
ness in an automated way. This ontology has identi-
fied the most important factor in consciousness as be-
ing the creation of infinitely diversible cognitive prod-
ucts of creativity based on cognitive pattern-matching
between particular collections of thoughts. Further-
more, three mayor neural brain cell abilities (de-
scribed in section 6) were discovered as being of high
importance for further researches in the field of cog-
nitive product generation. Transitivity was introduced
to be essential in the correct application of function
impacts within particular thoughts. In conclusion to
the created theory, two hypothesis can be derived:
Hypothesis 1. With the simulation of pattern
matches between mapped thought collectives a learn-
ing environment is able to construct cognition au-
tomatically by observing learning behavior during
course accomplishment.
Hypothesis 2. By observing learning behavior, a
learning environment is able to adapt, create and
differ learning fractals individually to support the
learner with suggestions in order to understand
materials better and faster.
Further research is needed to sequentially map
and process patterns for cognition detection in
E-learning environments. This knowledge answer the
question how cognition relates to consciousness on a
fundamental ontological basis.
REFERENCES
Falbo, R. A. (2004). Experiences in using a method for
building domain ontologies. Proc. of International
Workshop on Ontology In Action, Banff, Alberta,
Canada.
Fishwick, P. and Miller, J. (Jan. 2004). Winter SImulation
Conference, Proceedings of The 2004. IEEE, Piscat-
away.
Grubb, M. S. and Burrone, J. (2010). Activity-dependent
relocation of the axon initial segment fine-tunes neu-
ronal excitability. 465(7301), pages 1070–1074. Na-
ture.
Guizzardi and Wagner (op. 2010). Towards an ontological
foundation of discrete event simulation. In Proceed-
ings of the 2010 Winter Simulation Conference, eds. B.
Johansson, S. Jain, J. Montoya-Torres, J. Hugan, and
E. Ycesan, 652-664. Piscataway, New Jersey: Institute
of Electrical and Electronics Engineers, Inc. IEEE,
Piscataway.
Guizzardi, G. (2005). Ontological foundations for struc-
tural conceptual models, volume no. 015 of Telemat-
ica Instituut fundamental research series. Centre for
CSEDU2015-7thInternationalConferenceonComputerSupportedEducation
414