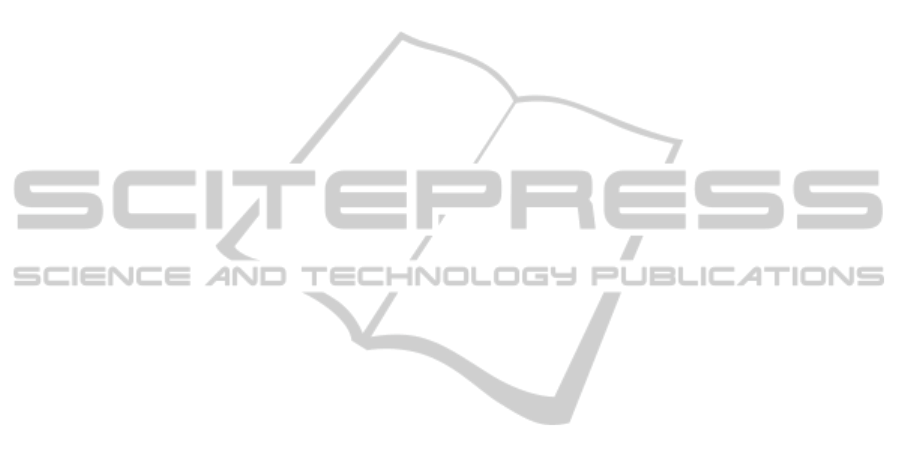
ogy is most likely to appear at the beginning of a ses-
sion. Similarly when a student starts working with
the linked list tutorial, the system displays a window
describing an analogy of people standing in a line.
Second approach is based on the fact that the tutor
frequently refers to analogy during a tutoring ses-
sion. Correspondingly, for every problem a step by
step analogy based example was fashioned that stu-
dent could refer to. We have recently run a controlled
experiment with three conditions: The First condition
provides an analogy at the beginning of the session.
The Second condition enables student access to anal-
ogy based step by step examples for every problem.
The Third condition enables student access to worked
out examples for every problem. We are currently
analyzing the results of these experiments to uncover
whether our implementation of analogy is effective.
ACKNOWLEDGEMENTS
This work is supported by award NPRS 5-939-1-155
from the Qatar National Research Fund.
REFERENCES
Carletta, J. (1996). Assessing agreement on classification
tasks: the kappa statistic. Computational linguistics,
22(2):249–254.
Chang, M. D. (2014). Analogy tutor: A tutoring system
for promoting conceptual learning via comparison. In
Twenty-Eighth AAAI Conference on Artificial Intelli-
gence.
Chen, L., Di Eugenio, B., Fossati, D., Ohlsson, S., and
Cosejo, D. (2011). Exploring effective dialogue act
sequences in one-on-one computer science tutoring
dialogues. In Proceedings of the 6th Workshop on In-
novative Use of NLP for Building Educational Appli-
cations, pages 65–75. Association for Computational
Linguistics.
Chi, M. T., Siler, S. A., Jeong, H., Yamauchi, T., and Haus-
mann, R. G. (2001). Learning from human tutoring.
Cognitive Science, 25(4):471–533.
Di Eugenio, B., Chen, L., Green, N., Fossati, D., and Al-
Zoubi, O. (2013). Worked out examples in computer
science tutoring. In Artificial Intelligence in Educa-
tion, pages 852–855. Springer.
Di Eugenio, B. and Glass, M. (2004). The kappa statistic:
A second look. Computational linguistics, 30(1):95–
101.
Ezen-Can, A. and Boyer, K. E. (2013). In-context evalua-
tion of unsupervised dialogue act models for tutorial
dialogue. In Proceedings of SIGDIAL, pages 324–
328.
Fossati, D. (2013). Chiqat: An intelligent tutoring system
for learning computer science. In Qatar Foundation
Annual Research Conference, number 2013.
Fossati, D., Di Eugenio, B., Ohlsson, S., and Brown, C.
(2015). Data driven automatic feedback generation in
the ilist intelligent tutoring system. Technology, In-
struction, Cognition and Learning, (To appear).
Fox, B. A. (1993). The Human Tutorial Dialogue
Project: Issues in the design of instructional systems.
Lawrence Erlbaum Associates Hillsdale, NJ.
Gadgil, S. and Nokes, T. (2009). Analogical scaffolding in
collaborative learning. In annual meeting of the Cog-
nitive Science Society, Amsterdam, The Netherlands.
Gentner, D. (1998). Analogy. A companion to cognitive
science, pages 107–113.
Gentner, D. and Colhoun, J. (2010). Analogical processes
in human thinking and learning. In Towards a theory
of thinking, pages 35–48. Springer.
Gentner, D., Loewenstein, J., and Thompson, L. (2003).
Learning and transfer: A general role for analogi-
cal encoding. Journal of Educational Psychology,
95(2):393.
Hofstadter, D. R. (2001). Analogy as the core of cognition.
The analogical mind: Perspectives from cognitive sci-
ence, pages 499–538.
Litman, D. J., Ros
´
e, C. P., Forbes-Riley, K., VanLehn, K.,
Bhembe, D., and Silliman, S. (2006). Spoken versus
typed human and computer dialogue tutoring. Interna-
tional Journal of Artificial Intelligence in Education,
16(2):145–170.
Lulis, E., Evens, M., and Michael, J. (2004). Implementing
analogies in an electronic tutoring system. In Intelli-
gent Tutoring Systems, pages 751–761. Springer.
Murray, T., Schultz, K., Brown, D., and Clement, J.
(1990). An analogy-based computer tutor for reme-
diating physics misconceptions. Interactive Learning
Environments, 1(2):79–101.
Nokes, T. J. and VanLehn, K. (2008). Bridging princi-
ples and examples through analogy and explanation.
In Proceedings of the 8th international conference
on International conference for the learning sciences-
Volume 3, pages 100–102. International Society of the
Learning Sciences.
Ohlsson, S., Di Eugenio, B., Chow, B., Fossati, D., Lu,
X., and Kershaw, T. C. (2007). Beyond the code-and-
count analysis of tutoring dialogues. Artificial intelli-
gence in education: Building technology rich learning
contexts that work, 158:349.
Passonneau, R. J. and Litman, D. J. (1997). Discourse seg-
mentation by human and automated means. Compu-
tational Linguistics, 23(1):103–139.
AStudyofAnalogyinComputerScienceTutorialDialogues
237