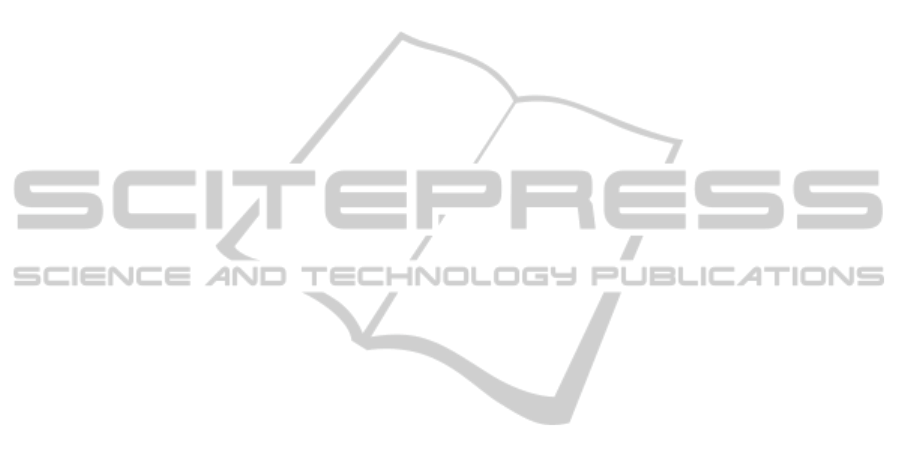
Things Better: Doing Better Things, pp. 161-171.
Budapest, Hungary: EDEN. Oxford, United Kingdom.
Brouns, F., Tammets, K., and Padrón-Nápoles, C. L.
(2014b). How can the EMMA approach to learning
analytics improve employability?. Retrieved from
http://dspace.learningnetworks.org/bitstream/1820/554
2/1/submission9_M4WS.pdf.
Burgos, D., and Corbí, A. (2014). A recommendation
model on personalised learning to improve the user’s
performance and interaction in MOOCs and OERs.
UNESCO Institute for Information Technologies in
Education. IITE 2014 International Conference. Oct
14th-15th, 2014. Moscow, Russia.
Cavanaugh, J. (2013). The Coming Personalization of
Postsecondary Education Competencies. CAEL 2013
Forum & News: Competency-Based Education, pp. 2-
5.
Collet, P. (2013). POEM (Personalised Open Education
for the Masses). Retrieved from http://
blog.educpros.fr/pierredubois/files/2013/07/Financem
ent.pdf.
Daniel, J. (2012). Making sense of MOOCs: Musings in a
maze of myth, paradox and possibility. Journal of
Interactive Media in Education, 3.
Daradoumis, T., Bassi, R., Xhafa, F., and Caballé, S.
(2013, October). A review on massive elearning
(MOOC) design, delivery and assessment. In P2P,
Parallel, Grid, Cloud and Internet Computing
(3PGCIC), pp. 208-213. IEEE.
De Maio, C., Loia, V., Mangione, G. R., and Orciuoli, F.
(2014). Automatic Generation of SKOS Taxonomies
for Generating Topic-Based User Interfaces in
MOOCs. In Open Learning and Teaching in
Educational Communities, pp. 398-403, Springer
International Publishing.
Elkherj, M. and Freund, Y. (2014, March). A system for
sending the right hint at the right time. In Proceedings
of the first ACM conference on Learning@ scale
conference: pp. 219-220. ACM.
Fasihuddin, H. A., Skinner, G. D., and Athauda, R. I.
(2014) Personalizing Open Learning Environments
through the adaptation to Learning Styles. ICITA
2014, 9
th
International Conference on Information
Technology and Applications, Sydney, Australia, July
2014. ISBN: 978-0-9803267-6-5.
Fasimpaur, K. (2013). Massive and Open. Learning and
Leading with Technology March/April 2013, pp. 12-
17.
Freeman, M. A. R. K., and Hancock, P. H. I. L. (2013).
Milking MOOCs: Towards the right blend in
accounting education. Academic Leadership Series, 4,
86-100.
Godwin-Jones, R. (2014). Emerging Technologies Global
Reach and Local Practice: the Promise of MOOCs.
Announcements & Call for Papers, 5.
Gutiérrez-Rojas, I., Alario-Hoyos, C., Pérez-Sanagustín,
M., Leony, D., and Delgado-Kloos, C. (2014a).
Scaffolding Self-learning in MOOCs. Proceedings of
the Second MOOC European Stakeholders Summit,
EMOOCs, 43-49.
Gutiérrez-Rojas, I., Leony, D., Alario-Hoyos, C., Pérez-
Sanagustín, M., and Delgado-Kloos, C. (2014b)
Towards an Outcome-based Discovery and Filtering of
MOOCs using moocrank.
Harman, K., and Koohang, A. (2013) MOOC 2050: A
Futuristic Tour. Issues in Information Systems, 14 (2),
pp. 346-352.
Henning, P. A., Heberle, F., Streicher, A., Zielinski, A.,
Swertz, C., Bock, J., and Zander, S. (2014).
Personalized Web Learning: Merging Open
Educational Resources into Adaptive Courses for
Higher Education. Personalization Approaches in
Learning Environments, 55.
Hollands, F. M. and Tirthali, D. (2014, May). MOOCs:
Expectations and reality. Full report. Center for
Benefit-Cost Studies of Education, Teachers College
Columbia University. Retrieved from http://
cbcse.org/wordpress/wp- content/uploads/2014/05/
MOOCs_Expectations_and_Reality.pdf.
Jona, K., and Naidu, S. (2014). MOOCs: Emerging
Research. Distance Education, 35(2), pp.141-144.
Kalz, M. (2014) (in press). Lifelong Learning and its
support with new technologies. In Smelser, N. J. and
Baltes P. B. (Eds.). International Encyclopaedia of the
Social and Behavioral Sciences. Pergamon: Oxford.
Kalz, M., and Specht, M. (2013). If MOOCs are the
answer-did we ask the right questions. Implications for
the design of large-scale open online courses.
Maastricht School of Management in its series
Working Papers, 2013/25.
Kay, J., Reimann, P., Diebold, E., and Kummerfeld, B.
(2013). MOOCs: So Many Learners, So Much
Potential... IEEE Intelligent Systems, 28(3), pp. 70-77.
Ketamo, H. (2014, June). Learning Fingerprint: Adaptive
Tutoring for MOOCs. In World Conference on
Educational Multimedia, Hypermedia and
Telecommunications, Vol. 2014, No. 1, pp. 2458-
2467.
Kizilcec, R. F., Piech, C., and Schneider, E. (2013, April).
Deconstructing Disengagement: Analyzing Learner
Subpopulations In Massive Open Online Courses. In
Proceedings of the third international conference on
learning analytics and knowledge: pp. 170-179. ACM.
Knox, J. (2014). From MOOCs to Learning Analytics:
Scratching the surface of the 'visual'. eLearn,
2014(11), 3.
Knox, J., Ross, J., Sinclair, C., Macleod, H., and Bayne, S.
(2014). MOOC Feedback: Pleasing All the People?.
Invasion of the MOOCs, 98.
Liyanagunawardena, T. R., Adams, A. A., and Williams,
S. A. (2013) MOOCs: A systematic study of the
published literature 2008-2012. The International
Review of Research in Open and Distance Learning,
14(3), pp. 202-227.
Martín, S., Peire, J., and Castro, M. (2013) Proyecto
WePrendo. Retrieved from http://www.ieec.uned.es/
CSEDU2015-7thInternationalConferenceonComputerSupportedEducation
96