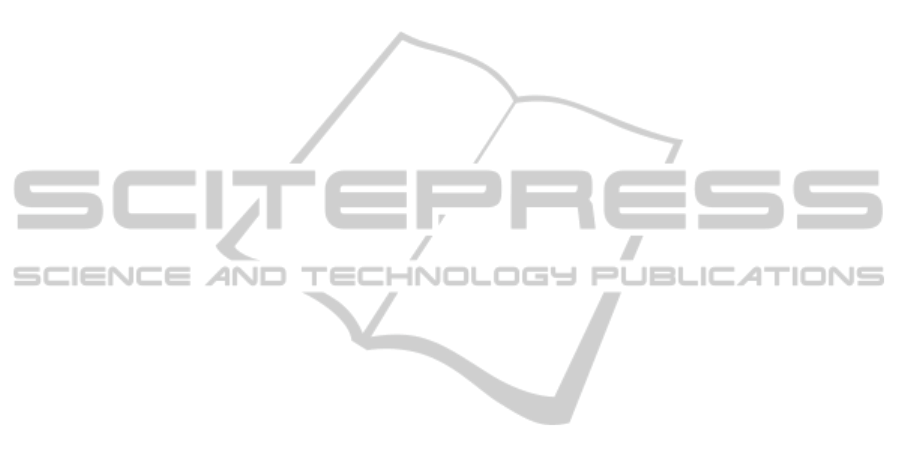
mal project managers (PM) in the day-to-day activi-
ties. Although the analysis of these project team dy-
namics have not been the main goal of the present
work, the authors are considering the idea of de-
termining the social behavioural profiles of project
members beyond their formal given roles.
For the future, the authors plan to validate the ob-
tained results using different datasets. They also in-
tend to use the communication data of the projects in
order to try to predict the final marks of students. Fi-
nally, it would be interesting to analyse message con-
tent as a way to improve the prediction of team mem-
ber roles.
ACKNOWLEDGEMENTS
The authors wish to recognise the financial support
of the “Vicerrectorado de Profesorado, Planificaci
´
on
e Innovaci
´
on Docente” of the University of La Rioja,
through the “Direcci
´
on Acadmica de Formaci
´
on e In-
novaci
´
on Docente” (APIDUR 2014).
REFERENCES
Alba-El
´
ıas, F., Gonz
´
alez-Marcos, A., and Ordieres-Mer
´
e,
J. (2013). An ict based project management learning
framework. In EUROCON, 2013 IEEE, pages 300–
306.
Alba-El
´
ıas, F., Gonz
´
alez-Marcos, A., and Ordieres-Mer
´
e, J.
(2014). An active project management framework for
professional skills development. International Jour-
nal of Engineering Education, 30(5):1242–1253.
Bouckaert, R. (2007). Bayesian Network Classifiers in
Weka for Version 3-5-6. The University of Waikato.
Breiman, L. (2001). Random forests. Machine Learning,
45(1):5–32.
Chen, I.-X. and Yang, C.-Z. (2010). Handbook of So-
cial Network Technologies and Applications, chap-
ter Visualization of Social Networks, pages 585–610.
Springer, Florida, USA.
Choa, H., Gayb, G., Davidsonc, B., and Ingraffe, A. (2007).
Social networks, communication styles, and learning
performance in a cscl community. Computers & Edu-
cation, 49(2):309–329.
Cohen, W. W. (1995). Fast effective rule induction. In
Twelfth International Conference on Machine Learn-
ing, pages 115–123. Morgan Kaufmann.
Duda, R. O., Hart, P. E., and Stork, D. G. (2000). Pattern
Classification. Wiley Interscience, 2 edition.
Friedman, J., Hastie, T., and Tibshirani, R. (2000). Addi-
tive logistic regression : A statistical view of boosting.
Annals of statistics, 28(2):337–407.
Gaines, B. and Compton, P. (1995). Induction of ripple-
down rules applied to modeling large databases. Jour-
nal of Intelligent Information Systems, 5(3):211–228.
George, S. and Leroux, P. (2002). An approach to automatic
analysis of learners’ social behavior during computer-
mediated synchronous conversations. In Cerri, S.,
Gouard
`
eres, G., and Paraguau, F., editors, Intelligent
Tutoring Systems, volume 2363 of Lecture Notes in
Computer Science, pages 630–640. Springer Berlin
Heidelberg.
Girasoli, A. J. and Hannafin, R. D. (2008). Using
asynchronous av communication tools to increase
academic self-efficacy. Computers & Education,
51(4):1676–1682.
Hall, M. and Frank, E. (2008). Combining naive bayes and
decision tables. In Proceedings of the 21st Florida
Artificial Intelligence Society Conference (FLAIRS),
pages 318–319. AAAI press.
Holmes, G., Pfahringer, B., Kirkby, R., Frank, E., and Hall,
M. (2001). Multiclass alternating decision trees. In
ECML, pages 161–172. Springer.
Hrastinski, S. (2008). The potential of synchronous com-
munication to enhance participation in online discus-
sions: A case study of two e-learning courses. Infor-
mation & Management, 45(7):499–506.
Kleinberg, J. M. (1999). Authoritative sources in a hyper-
linked environment. Journal of the ACM, 46(5):604–
632.
le Cessie, S. and van Houwelingen, J. (1992). Ridge es-
timators in logistic regression. Applied Statistics,
41(1):191–201.
Lopez, M. I., Romero, C., Ventura, S., and Luna, J. M.
(2012). Classification via clustering for predicting fi-
nal marks starting from the student participation in fo-
rums. In EDM’12, pages 148–151.
Martin, B. (1995). Instance-based learning : Nearest neigh-
bor with generalization. Technical report, University
of Waikato.
OGC (2009). Managing Successful Projects with
PRINCE2
TM
. Office Of Government Commerce.
Page, L., Brin, S., Motwani, R., and Winograd, T. (1999).
The pagerank citation ranking: Bringing order to the
web. Technical Report 1999-66, Stanford InfoLab.
Park, J. and Sandberg, I. W. (1991). Universal approxi-
mation using radial-basis-function networks. Neural
Comput., 3(2):246–257.
Pinheiro, C. A. R. (2011). Social Network Analysis in
Telecommunications. John Wiley & Sons, Hoboken,
New Jersey.
Platt, J. C. (1998). Fast training of support vector machines
using sequential minimal optimization. In Advances
in Kernel Methods - Support Vector Learning. MIT
Press.
PMI (2008). A Guide to the Project Management Body
of Knowledge (PMBOK Guide). Project Management
Institute, Newtown Square, PA, USA, 4th edition.
Quinlan, R. (1993). C4.5: Programs for Machine Learning.
Morgan Kaufmann Publishers, San Mateo, CA.
Ruck, D. W., Rogers, S. K., Kabrisky, M., Oxley, M. E.,
and Suter, B. W. (1990). The multilayer perceptron
as an approximation to a Bayes optimal discriminant
function. IEEE Transactions on Neural Networks,
1(4):296–298.
AnalysingOnlineEducation-basedAsynchronousCommunicationToolstoDetectStudents'Roles
423