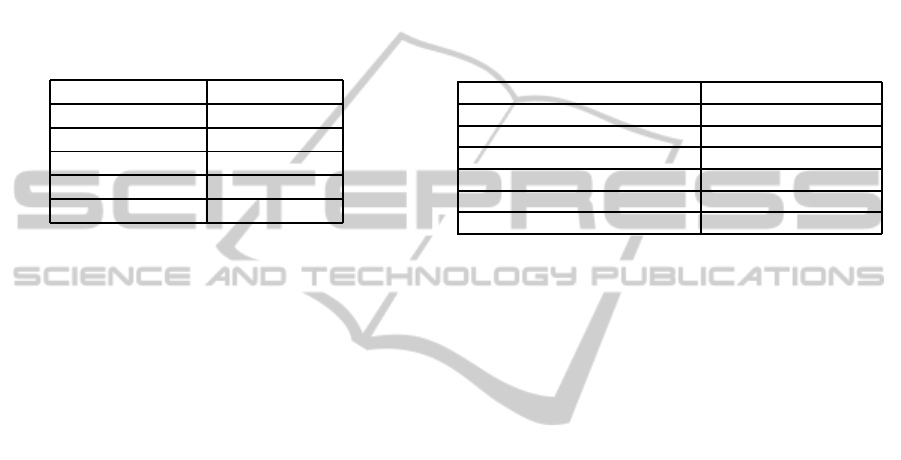
have to buy clothing and shoes anyway. Recent
reports (Fundac¸˜ao Calouste Gulbenkian, 2014) have
shown there are even potential savings in terms of
healthcare costs, as walking regularly does reduce
the risk of cardiovascular diseases and diabetes, for
instance. However, it is difficult to quantify direct
savings and for the purpose of this study the authors
decided not to consider them.
Overall the obtained estimated cost values for
each mode of transport were:
Table 2: Cost estimation per mode of transport.
Transport Cost
Car 0.36AC per km
Motorcycle 0.14AC per km
Public Transport 0.64AC per trip
Bicycle 0.03AC per km
Pedestrian 0AC per km
3.4.2 Emissions
For this project emissions were not measured due to
the granularity of data required and the project times-
pan. To ensure that the model represented the actual
Portuguese car fleet (Balsa, 2013) extensive measure-
ments had to be made and those would produce results
highly dependent on the driving style (Panis et al.,
2006).
More comprehensive models like the COPERT
and MOVES were not used due to some problems,
the MOVES model does not represent the Portuguese
car fleet (Duarte and Costa, 2010) while the COPERT
model does not have a data set representing Portugal
individually, only as part of the EU (Emisia, 2014).
Furthermore emission estimation can introduce a
great overhead if not properly evaluated, therefore,
within the scope of the work only the equivalent CO2
emissions for each mode of transport were consid-
ered.
Car emissions were obtained from the Carbon
Footprint Calculator (Carbon Footprint, 2012) for a
EU average petrol car with an engine up to 1.4L. The
value obtained was of 160.61 grams of CO
2
per km.
It is considered that this value reflects the average car
from the Portuguese fleet and therefore is adequate.
Motorcycle emissions were obtained from the
same source as the car ones (Carbon Footprint, 2012)
and the value obtained is 106.21 grams of CO
2
per
km.
Public transport emissions need to consider both
road based and rail based public transport. A previ-
ous work done by Quercus (Portuguese Environmen-
tal Agency), with results available online (Ecocasa,
2012). For road based public transport the value is
82 grams of CO
2
per km while for Portos rail based
transport system is 63 grams of CO
2
per km.
The European Cyclist Federation (European Cy-
clist Federation, 2012) states that cycling emissions
are around 21 grams of CO
2
per km.
For this paper it was considered that walking pro-
duced no emissions.
Overall the obtained estimated emission values for
each mode of transport were:
Table 3: Emission estimation per mode of transport.
Transport Emissions
Car 160.61 gCO
2
per km
Motorcycle 106.21 gCO
2
per km
Road-based Public Transport 82 gCO
2
per km
Rail-based Public Transport 63 gCO
2
per km
Bicycle 21 gCO
2
per km
Pedestrian 0 gCO
2
per km
4 DATA ANALYSIS
Using the collected and estimated values for each
mode of transport and pair of source and destination
the data was analysed to perceive patterns that would
allow to generate a model that could be extended to
other routes with different characteristics. The col-
lected GPS data was converted from CVS to GPX
format to be easier to interpret with mapping appli-
cations.
Due to errors induced by the measurements the
data points were fitted to the road to more correctly
represent reality. For each pair of source and destina-
tion the time taken and estimated cost and emissions
were evaluated for each mode of transportation. The
results were then averaged for all routes to give an
overall vision of the city along those 3 vectors.
The obtained results were extended to a model
where the cost, emission and time can by evaluated
for each mode of transport over distance (cf Figures
4,5, and 6)
Using the results obtained and the analysis along
each individual vector, an index that comprehends the
three parameters was developed. This index considers
different weights for time, emissions and cost that can
be tuned for different values (cf. Equation 1).
Index = Average(Time × Weight,
Distance× Emission× Weight,
Distance× Cost× Weight)
(1)
SMARTGREENS2015-4thInternationalConferenceonSmartCitiesandGreenICTSystems
46