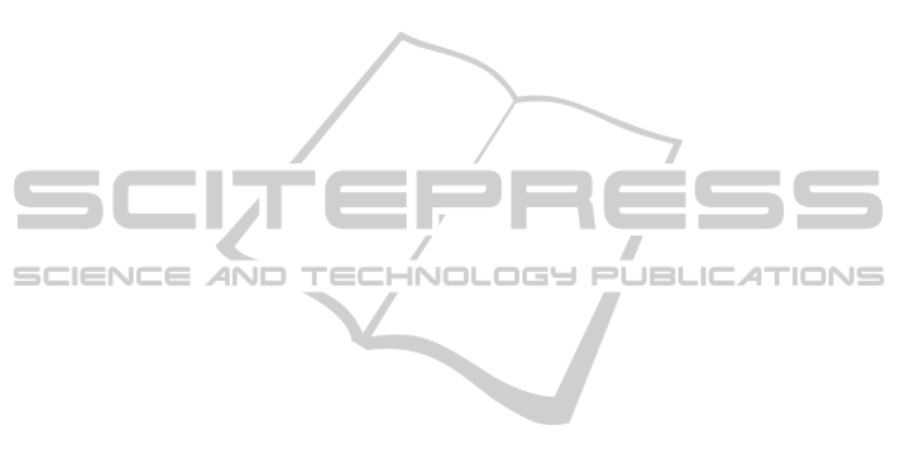
formance of our approach. For example, we will take
into account the correspondence between the learner’s
level and the resource’s prerequisites. We will also
pay attention on the similar behaviors of users in the
same communities (such as class, course, group of
discussion or social network).
ACKNOWLEDGEMENTS
This work has been fully supported by the French
General Commission for Investment (Commissariat
G
´
en
´
eral
`
a l’Investissement), the Deposits and Con-
signments Fund (Caisse des D
´
ep
ˆ
ots et Consigna-
tions) and the Ministry of Higher Education & Re-
search (Minist
`
ere de l’Enseignement Sup
´
erieur et de
la Recherche) within the context of the PERICLES
project (http://www.e-pericles.org).
REFERENCES
Avancini, H. and Straccia, U. (2005). User recommendation
for collaborative and personalised digital archives. Int.
J. Web Based Communities, 1(2):163–175.
Brin, S. and Page, L. (1998). The anatomy of a large-scale
hypertextual web search engine. In Proceedings of the
Seventh International Conference on World Wide Web
7, WWW7, pages 107–117, Amsterdam, The Nether-
lands, The Netherlands. Elsevier Science Publishers
B. V.
Chan, N., Roussanaly, A., and Boyer, A. (2014). Learn-
ing resource recommendation: An orchestration of
content-based filtering, word semantic similarity and
page ranking. In Rensing, C., de Freitas, S., Ley, T.,
and Mu
˜
noz-Merino, P., editors, Open Learning and
Teaching in Educational Communities, volume 8719
of Lecture Notes in Computer Science, pages 302–
316. Springer International Publishing.
Drachsler, H., Pecceu, D., Arts, T., Hutten, E., Rutledge,
L., Rosmalen, P., Hummel, H., and Koper, R. (2009).
Remashed — recommendations for mash-up personal
learning environments. In Proceedings of the 4th Eu-
ropean Conference on Technology Enhanced Learn-
ing: Learning in the Synergy of Multiple Disciplines,
EC-TEL ’09, pages 788–793, Berlin, Heidelberg.
Springer-Verlag.
Engelhardt, M., Hildebrand, A., Lange, D., and Schmidt,
T. C. (2006). Reasoning about elearning multimedia
objects. In First International Workshop on Seman-
tic Web Annotations for Multimedia (SWAMM), joint
with the 15th World Wide Web (WWW) Conference,
Edinburgh, Scotland.
Hendez, M. and Achour, H. (2014). Keywords extrac-
tion for automatic indexing of e-learning resources.
In Computer Applications Research (WSCAR), 2014
World Symposium on, pages 1–5.
Huang, Y.-M., Huang, T.-C., Wang, K.-T., and Hwang, W.-
Y. (2009). A markov-based recommendation model
for exploring the transfer of learning on the web. Ed-
ucational Technology & Society, 12(2):144–162.
Hummel, H. G. K., van den Berg, B., Berlanga, A. J.,
Drachsler, H., Janssen, J., Nadolski, R., and Koper,
R. (2007). Combining social-based and information-
based approaches for personalised recommendation
on sequencing learning activities. IJLT, 3(2):152–168.
Janssen, J., Tattersall, C., Waterink, W., van den Berg, B.,
van Es, R., Bolman, C., and Koper, R. (2007). Self-
organising navigational support in lifelong learning:
How predecessors can lead the way. Comput. Educ.,
49(3):781–793.
Khribi, M. K., Jemni, M., and Nasraoui, O. (2009). Au-
tomatic recommendations for e-learning personaliza-
tion based on web usage mining techniques and infor-
mation retrieval. Educational Technology & Society,
12(4):30–42.
Koutrika, G., Ikeda, R., Bercovitz, B., and Garcia-Molina,
H. (2008). Flexible recommendations over rich data.
In Proceedings of the 2008 ACM Conference on Rec-
ommender Systems, RecSys ’08, pages 203–210, New
York, NY, USA. ACM.
Lemire, D., Boley, H., McGrath, S., and Ball, M. (2005).
Collaborative filtering and inference rules for context-
aware learning object recommendation. International
Journal of Interactive Technology and Smart Educa-
tion, 2(3).
Manning, C. D., Raghavan, P., and Schutze, H. (2008). In-
troduction to Information Retrieval. Cambridge Uni-
versity Press, New York, NY, USA.
Manouselis, N., Drachsler, H., Vuorikari, R., Hummel, H.,
and Koper, R. (2011). Recommender systems in tech-
nology enhanced learning. In Ricci, F., Rokach, L.,
Shapira, B., and Kantor, P. B., editors, Recommender
Systems Handbook, pages 387–415. Springer US.
Manouselis, N., Vuorikari, R., and Assche, F. V. (2007).
Simulated analysis of maut collaborative filtering for
learning object recommendation. In In Workshop pro-
ceedings of the EC-TEL conference: SIRTEL07 (EC-
TEL ’07, pages 17–20.
Nadolski, R. J., van den Berg, B., Berlanga, A. J., Drach-
sler, H., Hummel, H. G., Koper, R., and Sloep, P. B.
(2009). Simulating light-weight personalised recom-
mender systems in learning networks: A case for
pedagogy-oriented and rating-based hybrid recom-
mendation strategies. Journal of Artificial Societies
and Social Simulation, 12(1):4.
Nilsson, M., Johnston, P., Naeve, A., and Powell, A. (2006).
The future of learning object metadata interoperabil-
ity. In Koohang, A., editor, Principles and Practices
of the Effective Use of Learning Objects. Informing
Science Press.
Page, L., Brin, S., Motwani, R., and Winograd, T. (1999).
The pagerank citation ranking: Bringing order to the
web. Technical Report 1999-66, Stanford InfoLab.
Previous number = SIDL-WP-1999-0120.
Saini, P., Ronchetti, M., and Sona, D. (2006). Automatic
generation of metadata for learning objects. In Ad-
CSEDU2015-7thInternationalConferenceonComputerSupportedEducation
128