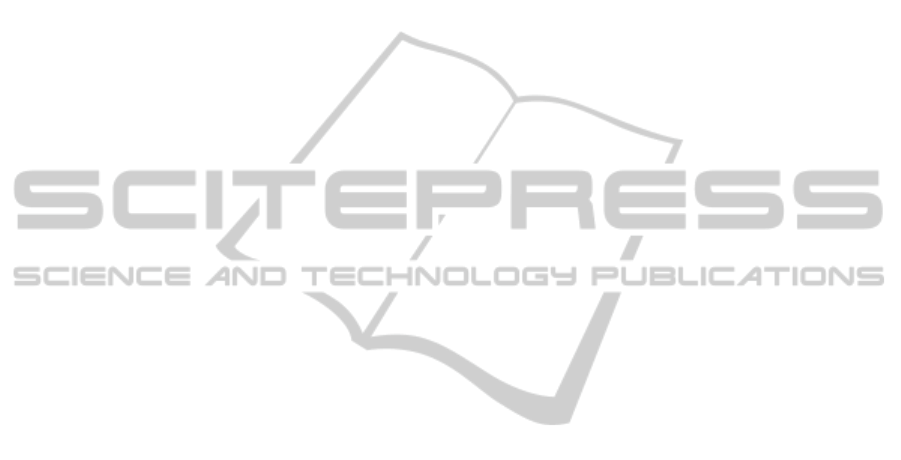
lighting and the slack for energy optimization, so
automated lighting control can produce lower
(absolute and relative) efficiency gains compared to
“darker” days when artificial light is used more.
Results indicate that subject occupants, when
manually adjusting dimming levels, consistently keep
the lights at higher luminance levels compared to
their comfort zone boundary. This slack is used by the
automated control to produce energy savings. This is
a natural human reaction and has been consistently
observed in all collected measurements so far.
A related side-effect is that the “comfort”
automated strategy performs consistently better than
the manual control. Users are likely to tolerate some
discomfort to avoid the inconvenience of going to the
lighting switch to dim the lights (Figure 2). The area
between the two step-wise lines is a proxy of the
possible energy savings by automated control. As
shown in Table 1, 29.2% less energy is used by the
“Wise” strategy for a 6.22% sacrifice in comfort of
the occupant (from 88.78% to 82.56%).
Also, it is possible and practical to implement
several control strategies which span the entire energy
efficiency vs. occupancy comfort continuum. Tight
comfort control removes the main entry barrier for the
widespread uptake of automated lighting control
solutions. Controlling user (dis)comfort allows the
facility manager to gain energy efficiency from day
one without hampering occupant comfort – and
potentially progressively further enhancing energy
efficiency by trading off some comfort.
THOR allows automated lighting control systems
to consistently improve occupant visual comfort and
reduce energy consumption compared to manual
control. The two key enablers are: i) the learning
algorithm that unambiguously quantifies personal
visual comfort preferences and improving acceptance
levels for automated lighting control strategies and,
ii) the continuous monitoring of ambient conditions
that provide the necessary stimuli to the automated
lighting control.
5 RESIDENTIAL LIGHTING
CONTROL APP
A residential version of THOR has been developed
for mobile devices. It uses available gateways to dim
the lights and uses sensors (cameras, luminance
sensors, movement sensors) existing on devices to
offer enhanced functionality for personalized
(comfort based) light control. A free version, called
Hue Mate, offering automated personalized light
control of Philips Hue lights is available in Google
Play and App Store.
6 CONCLUSIONS AND FUTURE
WORK
This paper introduces THOR, an innovative
framework for automated, personalized lighting
control in commercial buildings. It is based on a
dynamic occupant profiling mechanism constantly
adapting to real-time events and ambient information.
The core behavioural profiling engine is transparent
and entirely implicit, requiring no direct occupant
feedback. Integrated but flexible control strategies
can reach high levels of savings and comfort. Pilot
assessment indicated more than 10% energy savings
retaining comfort levels above 90% or more than
35% savings retaining comfort levels above 75%.
REFERENCES
US Department of Energy, 2010. Buildings Energy Data
Book.
El-TERTIARY Project, 2008. Monitoring Electricity
Consumption in the Tertiary Sector.
US Department of Energy, 2013. Analysis and
Representation of Miscellaneous Electric Loads in
NEMS.
Zimmermann, G., 2003. Modeling the building as a
system”, In 8th International IBPSA Conference. p.
1483 – 1490.
Zimmermann, G., 2006. Modeling and simulation of
dynamic user behavior in buildings - a lighting control
case study”, In eWork and eBusiness in architecture,
engineering and construction: Proceedings of the 6th
European conference on product and process
modelling. p. 309-316, Taylor & Francis Group.
Robinson, R., 2006, Some trends and research needs in
energy and comfort prediction. In Proceedings of
comfort and energy use in building, Windsor, UK.
V. Singhvi, A. Krause, C. Guestrin, J.H. Garrett Jr., H.S.
Matthews, 2005. Intelligent light control using sensor
networks. In Proceedings 3rd International Conference
on Embedded Networked Sensor Systems. pp. 218–229.
Y.-J. Wen, A. Agogino, 2008. Wireless networked lighting
systems for optimizing energy savings and user
satisfaction, In Wireless Hive Networks Conference, pp.
1–7.
H. Chen, P. Chou, S. Duri, H. Lei, J. Reason, 2009. The
design and implementation of a smart building control
system. In IEEE International Conference on e-
Business Engineering, pp. 255–262.
B. Dong, B. Andrew, 2009. Sensor-based occupancy
behavioral pattern recognition for energy and comfort
CombinedVisualComfortandEnergyEfficiencythroughTruePersonalizationofAutomatedLightingControl
269