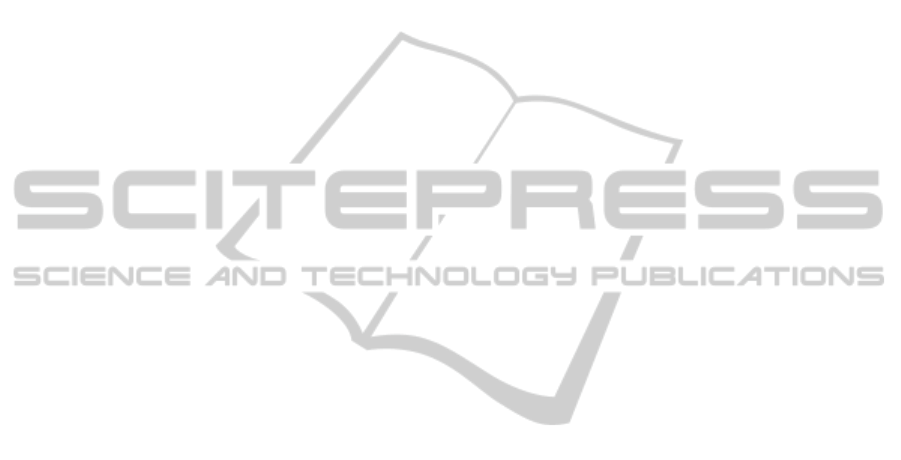
and Rackspace). The selection of the IaaS offerings
consisted of evaluating the different providers and their
corresponding optimized VM instances (Micro, Gen-
eral Purpose, Compute Optimized, and Memory Opti-
mized). The simulation environment was migrated and
its performance evaluated using an artificial workload.
A second step in our analysis consisted on extrapolat-
ing the obtained results towards estimating the incurred
costs for running the simulation environment on- and
off-premise. The analyses showed a beneficial impact
in the performance and a significant reduction of mon-
etary costs when migrating the simulation environment
to the majority of off-premise Cloud offerings.
Despite our efforts towards analyzing and finding
the most efficient IaaS Cloud service to deploy and run
our simulation environment, our experiments solely fo-
cused on IaaS offerings. Future works focus on analyz-
ing further service models, i.e. Platform-as-a-Service
(PaaS) or Database-as-a-Service (DBaaS), as well as
evaluating the distribution of the different components
constituting the simulation environment among multi-
ple Cloud offerings. Investigating different autoscaling
techniques and resources configuration possibilities is
also part of future work, e.g. feeding the application
distribution system proposed in (G
´
omez S
´
aez et al.,
2014b) with such empirical observations.
ACKNOWLEDGEMENTS
The research leading to these results has received fund-
ing from the FP7 EU project ALLOW Ensembles
(600792), the German Research Foundation (DFG)
within the Cluster of Excellence in Simulation Technol-
ogy (EXC310), and the German DFG project Bench-
Flow (DACH Grant Nr. 200021E-145062/1).
REFERENCES
Andrikopoulos, V., Song, Z., and Leymann, F. (2013). Sup-
porting the migration of applications to the cloud
through a decision support system. In Cloud Com-
puting (CLOUD), 2013 IEEE Sixth International Con-
ference on, pages 565–572. IEEE.
Binkele, P. and Schmauder, S. (2003). An atomistic Monte
Carlo Simulation of Precipitation in a Binary System.
Zeitschrift f
¨
ur Metallkunde, 94(8):858–863.
de Oliveira, D., Oca
˜
na, K. A. C. S., Ogasawara, E. S., Dias,
J., Bai
˜
ao, F. A., and Mattoso, M. (2011). A Perfor-
mance Evaluation of X-Ray Crystallography Scientific
Workflow Using SciCumulus. In Liu, L. and Parashar,
M., editors, IEEE CLOUD, pages 708–715. IEEE.
G
´
omez S
´
aez, S., Andrikopoulos, V., Leymann, F., and
Strauch, S. (2014a). Design Support for Performance
Aware Dynamic Application (Re-)Distribution in the
Cloud. IEEE Transactions on Services Computing (to
appear).
G
´
omez S
´
aez, S., Andrikopoulos, V., Wessling, F., and Mar-
quezan, C. C. (2014b). Cloud Adaptation & Applica-
tion (Re-)Distribution: Bridging the two Perspectives.
In Proceedings EnCASE’14, pages 1–10. IEEE Com-
puter Society Press.
G
¨
orlach, K., Sonntag, M., Karastoyanova, D., Leymann,
F., and Reiter, M. (2011). Conventional Workflow
Technology for Scientific Simulation, pages 323–352.
Guide to e-Science. Springer-Verlag.
Juve, G., Chervenak, A., Deelman, E., Bharathi, S., Mehta,
G., and Vahi, K. (2013). Characterizing and Profiling
Scientific Workflows. Future Gener. Comput. Syst.,
29(3):682–692.
Juve, G., Deelman, E., Vahi, K., Mehta, G., Berriman, B.,
Berman, B., and Maechling, P. (2009). Scientific Work-
flow Applications on Amazon EC2. In E-Science Work-
shops, 2009 5th IEEE International Conference on,
pages 59–66.
Molnar, D., Binkele, P., Hocker, S., and Schmauder, S.
(2010). Multiscale Modelling of Nano Tensile Tests
for different Cu-precipitation States in
α
-Fe. In Proc.
of the 5th Int. Conf. on Multiscale Materials Modelling,
pages 235–239.
Ostermann, S., Iosup, A., Yigitbasi, N., Prodan, R.,
Fahringer, T., and Epema, D. (2010). A Performance
Analysis of EC2 Cloud Computing Services for Scien-
tific Computing. In Cloud Computing, pages 115–131.
Springer.
Pathirage, M., Perera, S., Kumara, I., and Weerawarana,
S. (2011). A Multi-tenant Architecture for Business
Process Executions. In Proceedings of the 2011 IEEE
International Conference on Web Services, ICWS ’11,
pages 121–128, Washington, DC, USA. IEEE Com-
puter Society.
R
¨
ock, C., Harrer, S., and Wirtz, G. (2014). Performance
Benchmarking of BPEL Engines: A Comparison
Framework, Status Quo Evaluation and Challenges.
In 26th International Conference on Software Engi-
neering and Knowledge Engineering (SEKE), pages
31–34, Vancouver, Canada.
Skouradaki, M., Roller, D. H., Frank, L., Ferme, V., and
Pautasso, C. (2015). On the Road to Benchmarking
BPMN 2.0 Workflow Engines. In Proceedings of the
6th ACM/SPEC International Conference on Perfor-
mance Engineering ICPE 2015, pages 1–4. ACM.
Sonntag, M., Hahn, M., and Karastoyanova, D. (2012).
Mayflower - Explorative Modeling of Scientific Work-
flows with BPEL. In Proceedings of the Demo Track of
the 10th International Conference on Business Process
Management (BPM 2012), CEUR Workshop Proceed-
ings, 2012, pages 1–5. CEUR Workshop Proceedings.
Sonntag, M., Hotta, S., Karastoyanova, D., Molnar, D., and
Schmauder, S. (2011a). Using Services and Service
Compositions to Enable the Distributed Execution of
Legacy Simulation Applications. In Abramowicz, W.,
Llorente, I., Surridge, M., Zisman, A., and Vayssi
`
ere,
J., editors, Towards a Service-Based Internet, Proceed-
CLOSER2015-5thInternationalConferenceonCloudComputingandServicesScience
360