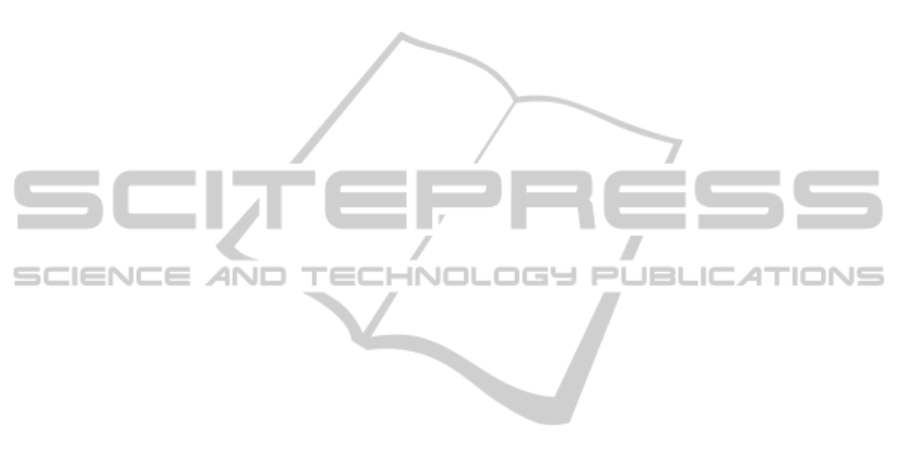
The value of Eps is determined as the distance
between the researchers’ profiles. A researcher's
profile consists of all its relevant rubrics and is
presented as a vector. VINITI rubricator of
knowledge areas is used as a rubricator. The set of
vectors forms a matrix of researchers's profiles . To
calculate the distance a cosine measure of adjacency
is used. The value MinPt is the minimum number of
the subjects of scientific school, i.e. the subjects of
"the communities of interest" in the model of a
scientific community.
For approbation of the proposed approach we
chose scientific communities of D. Serikbayev
EKSTU and Ioffe Physical-Technical Institute of the
Russian Academy of Science (Ioffe Institute). Papers
and research adirections of their scientifc
communities were examined. The results of
numerical experiments confirmed the efficiency of
the clustering algorithm used.
6 CONCLUSIONS
This paper describes the realization of monitoring
the development of university scientific schools,
which is one of functional components of the
technological approach to university scientific
knowledge management. Some models, methods,
and technologies of university scientific knowledge
life cycle support processes are considered.
The paper describes the developed model of a
specialist which reflects the level of scientific
activity productivity based on the calculation of
entropy and overall scientific activity evaluation.
The approach to identification of university
scientific schools based on the clustering of
university scientific community by common
interests is proposed.
The next stage of this work is to address the
problem of assessment of university scientific
activities and the degree of its integration with
educational process.
The work was performed under grant "The
development of an e-university's ontological
knowledge base”, state registration number
0213RK00305
REFERENCES
Klimov, S. M., 2002. Intellectual resources of society.
SPb: IFEREL, Znanie.
Ackoff, R.L., 1989. From Data to Wisdom. In Journal of
Applied Systems Analysis, vol. 16. pp. 3-9.
Zaim H., 2007. Performance of Knowledge Management
Practices: a causal analysis. Knowledge Management,
vol. 11(6), pp. 54-67. url:http://dx.doi.org/
10.1108/13673270710832163.
Miles, I., 2005. Knowledge intensive business services:
Prospects and policies. Emerald Group Publishing
Limited, vol. 7(6). pp. 39-63.
DOI 10.1108/14636680510630939.
Scarso, E., Bolisani, E., 2010. Knowledge-based strategies
for knowledge intensive business services: A multiple
case-study of computer service companies . Electronic
Journal of Knowledge Management. url: www.ejkm
com, vol. 8(1). pp. 151-160.
Tuzovskiy, A. F., 2007. The development of knowledge
management systems based on a single ontological
knowledge base. In Bulletin of the Tomsk Polytechnic
University, vol2(310), pp. 182-185.
Sveiby, Karl-Erik., 1989. The Invisible Balance Sheet.
Stockholm, 138 p.
Staab S., Schunurr H-P., Studer R., Sure Y., 2001.
Knowledge processes and ontologies. IEEE Intelligent
Systems, Special Issue on Knowledge Management,
vol. 16(1), pp. 26-34.
NC STI RK. Scientific schools and priorities for the
development of the country. URL:
http://exclusive.kz/bez-rubriki/22068.
Trubina, I. O., Zabelina, I.N., 2011. Creating personnel's
positive motivation in higher education institutions in
the process of education and development of scientific
schools. In Creative Economy, vol. 1(49), pp. 30-36.
Tuzovskiy, A. F., 2007. Creating and using a knowledge
base of specialists' competence profiles at
organizations. In Bulletin of the Tomsk Polytechnic
University, vol. 310(2), pp. 186-189.
Zhomartkyzy, G., Balova, T., Milosz, M., 2014.
Information Models and Methods of the University's
Scientific Knowledge Life Cycle Support. Proceedings
of the 2014 Federated Conference on Computer
Science and Information Systems, pp. 279 – 285.
All-Russian Institute of Scientific and Technical
Information, url:
http://scs.viniti.ru/rubtree/main.aspx?tree=RV.
Adamic, L., Zhang, J., Bakshy, E., Ackerman, M.S., 2008.
Knowledge sharing and yahoo answers: everyone
knows something. Proceedings of the 17th
international conference on World Wide Web. pp. 21-
25.
Baesso, P. T.,Wolfgand, Matsui Siqueira S. and Cristina
Vasconcelos de Andrade L., 2014. Finding Reliable
People in Online Communities of Questions and
Answers.-AnalysisofMetricsandScopeReduction. In
Proceedings of the 16th International Conference on
Enterprise Information Systems, pp. 526-535, DOI:
10.5220/0004954005260535.
Cantador, I., Castells P., 2011. Extracting multilayered
Communities of Interest from semantic user profiles:
Application to group modeling and hybrid
recommendations . Computers in Human Behavior,
vol. 27(4), pp. 1321-1336.
Bolshakova, E. I., Klyshinsky, E. S., Lande, D. V.,
MonitoringtheDevelopmentofUniversityScientificSchoolsinUniversityKnowledgeManagement
229