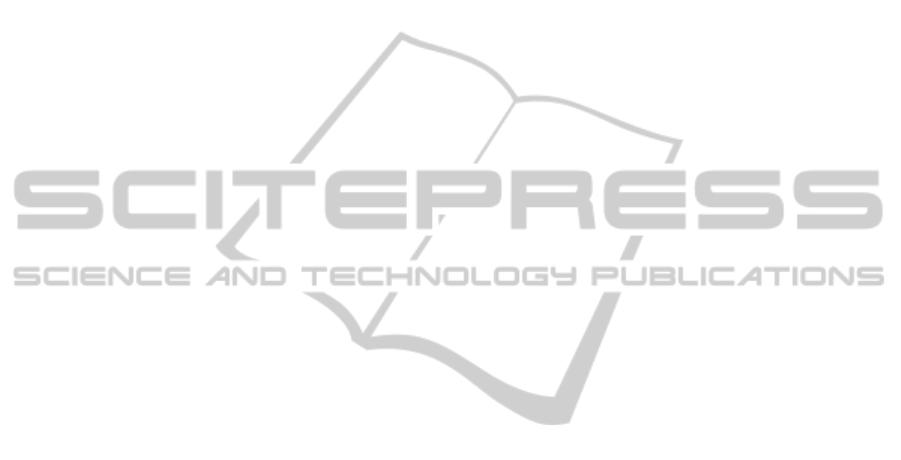
nonlinearity, emergency and surprise, and even, as
support to narrow, guide, verify and/or correct the
results of other Spatial Analysis alternatives.
Of course, questions remain unresolved, the fact
is that the spatial analysis currently done with GIS is
weak to support decision-making in situations like
those presented here, specially because they are not
designed for a group to develop in an interdisciplinary
way, a spatial analysis on complex spatial scenarios.
Moreover, it is not intended to imply, nor intended
this work to discredit the current way of doing spatial
analysis. But it does to raise awareness regarding the
necessity to consider new research lines in spatial
analysis that take into account the participation of
multidisciplinary groups to develop knowledge of
geographic space in an interdisciplinary way, with the
aim to refine what until now has been done; because
as Albert Einstein hinted: in order to obtain different
results, is imperative to do different things.
ACKNOWLEDGEMENTS
We specially thank our PhD colleague José J. Diez-
Rodríguez for his valuable collaboration in the
development of this work, as well as the National
Council of Science and Technology of Mexico
(CONACYT) for its support.
REFERENCES
Balram, S. & Dragićević, S., 2006. Collaborative
geographic information systems, United Kingdom: Idea
Group Publishing.
Blaschke, T. & Merschdorf, H., 2014. Geographic
information science as a multidisciplinary and
multiparadigmatic field. Cartography and Geographic
Information Science, 41(3), pp.196–213. Available at:
http://www.tandfonline.com/doi/abs/10.1080/1523040
6.2014.905755 [Accessed April 29, 2014].
Buzai, G.D., 2011. Modelos de localización-asignación
aplicados a servicios públicos urbanos: Análisis
espacial de Centros de Atención Primaria de Salud en
la ciudad de Luján, Argentina. Cuadernos de Geografía
- Revista Colombiana de Geografía, 20(2), pp.111–123.
Chang, Z.E. & Li, S., 2013. Geo-Social Model: A
Conceptual Framework for Real-time
Geocollaboration. Transactions in GIS, 17(2), pp.182–
205. Available at: http://doi.wiley.com/10.1111/j.1467-
9671.2012.01352.x [Accessed May 16, 2013].
Densham, P.J. & Goodchild, M.F., 1994. Spatial Decision
Support Systems, Santa Barbara, California, E.U.A.
Emsellem, K., Liziard, S. & Scarella, F., 2012. La
géoprospective: l’émergence d’un nouveau champ de
recherche? L’Espace géographique, 2(41), pp.154–168.
Available at: http://www.cairn.info/revue-espace-
geographique-2012-2-page-154.htm.
Fischer, M. M., 2006a. Spatial Analysis and
GeoComputation, Viena, Austria: Springer Berlin
Heidelberg.
Fischer, M. M., 2006b. Spatial Analysis in Geography. In
Spatial Analysis and GeoComputation.
Fischer, M. M. & Getis, A. eds., 2010. Handbook of
Applied Spatial Analysis. Software Tools, Methods and
Applications, Springer-Verlag Berlin Heidelberg.
Available at: http://www.springerlink.com/index/10.
1007/978-3-642-03647-7.
Flanagin, A.J. & Metzger, M.J., 2008. The credibility of
volunteered geographic information. GeoJournal, 72(3-
4), pp.137–148. Available at: http://link.springer.com/
10.1007/s10708-008-9188-y [Accessed May 21, 2013].
Franks, N.R. et al., 2002. Information flow, opinion polling
and collective intelligence in house-hunting social
insects. Philosophical transactions of the Royal Society
of London. Series B, Biological sciences,
357(October), pp.1567–1583.
Godet, M., Durance, P. & Gerber, A., 2008. Strategic
Foresight La Prospective Use and Misuse of Scenario
Building, Paris, France. Available at:
http://scholar.google.com/scholar?hl=en&btnG=Searc
h&q=intitle:Strategic+Foresight+La+Prospective+Use
+and+Misuse+of+Scenario+Building#2.
Gomez, B. & Jones, J.P., 2010. Research methods in
geography, United Kingdom: A John Wiley & Sons,
Ltd., Publication.
Goodchild, M.F., 2007. Citizens as sensors: the world of
volunteered geography. GeoJournal, 69, pp.211–221.
Goodchild, M.F. & Haining, R.P., 2004. GIS and spatial
data analysis: Converging perspectives. Papers in
Regional Science, 83(1), pp.363–385. Available at:
http://www.redalyc.org/articulo.oa?id=28900609.
Guzmán Peña, A. R., 2013. Proposal of a Model of
Territorial Intelligence. Journal of Technology
Management & Innovation, 8(ALTEC), pp.76–83.
Haller, E.A., 2007. Geospatial analysis framework. Brain:
Broad Research in Artificial Intelligence and
Neuroscience, 1(2), pp.166–171.
Houet, T. & Gourmelon, F., 2014. La géoprospective -
apport de la dimension spatiale aux démarches
prospectives. Cybergeo: European Journal of
Geography [En ligne], pp.1–9. Available at: http://
cybergeo.revues.org/26194.
Hudson-Smith, A. et al., 2009. NeoGeography and Web
2.0: concepts, tools and applications. Journal of
Location Based Services, 3(2), pp.118–145. Available
at: http://www.tandfonline.com/doi/abs/10.1080/174
89720902950366 [Accessed March 1, 2013].
Jankowski, P. et al., 1997. Spatial group choice: a SDSS
tool for collaborative spatial decision-making.
International Journal of Geographical Information
Science, 11(6), pp.577–602.
Jankowski, P., Fraley, G. & Pebesma, E., 2014. An
exploratory approach to spatial decision support.
Computers, Environment and Urban Systems, 45,
pp.101–113. Available at: http://linkinghub.elsevier.