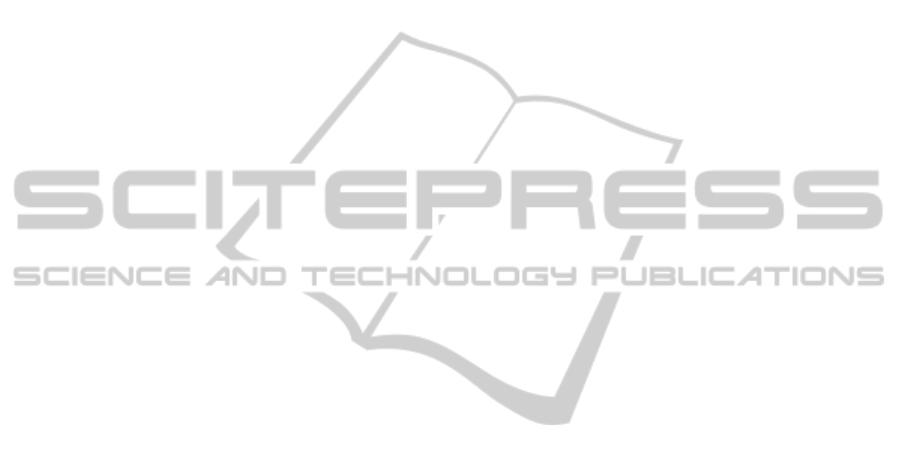
2.4 Irrigation
Drip irrigation was used in the study. Ten drip lines
were crossing the plots with 20cm spacing between
the emitters. Water required was calculated based on
crop evapotranspiration for each stage of wheat
development. The historical data like daily
maximum and minimum soil temperature and
humidity, wind speed and the meteorological data
for Seeb weather station were used. Visual basic in
Excel sheet was used to create sheet for reference
water requirement (ETo). The sheet is based on
Penman – Monteith method. To determine the time
of irrigation, the discharge of water from meter was
recorded. Hence, the time of irrigation was
calculated by dividing volume of water required by
the discharge.
2.5 Sensors
The inputs parameters including water potential and
soil temperature, for wheat simulation model can be
obtained from the sensors installed in the field. A
229 heat dissipation sensor from Campbell Scientific
Company, USA, is used to measure water potential
indirectly using principle of heat dissipation. The
principle of heat dissipation is whenever there is
water potential gradient between sensors and the
surrounding soil, water movement between sensor
and soil take certain time to reach equilibrium.
Hydraulic Equilibration time depend on magnitude
of water potential gradient and hydraulic
conductivity. The changes in water content of sensor
ceramic matric lead to change in the thermal
conductivity of sensor/ soil complex. There is
exponential relationship between water content and
thermal conductivity. As water content in the
ceramic sensor increase, the thermal conductivity
increase. A 229 heat dissipation sensor is porous
ceramic cylindrical shaped with thermocouple and
heating element at the middle of the cylinder. It has
the ability to measure a wide range of matric
potential from -10 to -2500KPa and it is compatible
with most Campbell Scientific data logger and
multiplexer. Also, it is known by long lasting
without need for maintenance. The 229 should be
installed horizontally at the desired depth and good
contact between the ceramic cylinder and soil must
be exist (Instruction manual of model 229, Campbell
Scientific Inc.). Soil water potential can also be
measured by using MPS2 from Decagon Devices
Inc., USA. MPS2 is ideal sensor for a range of water
potential measurement between -0.01 and -0.5 MPa
and soil temperature between -40 and 60C (Decagon
Devices Inc.). A 5TE sensor from Decagon devices,
USA, is used to measure volumetric water content,
soil temperature and electrical conductivity. It
measures the three parameters independently. The
volumetric water content is obtained by measuring
the dielectric constant of the media using
electromagnetic field supplied from the sensor with
70 MHz while the soil temperature is obtained from
thermistor which is installed at surface of the sensor
with a range of readings of -40 to 50°C. Electrical
conductivity is obtained by using stainless steel
electrode array and the reading is taking within the
range of 0 to 23 ds/m. The sensor is easily installed
in the field through pushing it directly to
undisturbed. EC is measured by applying alternating
current to two electrodes and measuring the
resistance between them soil (Decagon Devices
Inc.).
2.6 Linking WSM to GIS
To link the wheat simulation model in GIS, soil
temperature and volumetric water content raster
layers were required as input for the model and they
were extracted from the European Centre for
Medium-Range Weather Forecasts (ECMWF)
website which is an independent intergovernmental
organization established in 1975. The Centre
provides a catalogue of forecast data worldwide that
can be purchased by businesses and other
commercial customers for national community.
Different spatial analysis tools in GIS were used to
create the final emergence map mainly rater
calculator and reclassifying tools (Figure 2).
2.7 Statistical Analysis of Physical
Characteristics of Wheat
Physical characteristics of wheat like length of plant,
number of spikes per plant, number of seeds per
spike and weight of 100 seeds of each variety were
examined at the late season of each planting time.
Plant height was measured by taking average length
of random selection of plants using meter tab from
the soil surface to the begging of the spike. The
average plant length in each plot was recorded.
Random plants were also selected to find out
number of spikes in it and average numbers of seed
in each spike were counted. After the seeds removed
from its spike, 100 seeds from each plot was
weighed using digital scale. For statistical analysis,
ANOVA for single factor was used to examine the
differences among each factor.
GISTAM2015-1stInternationalConferenceonGeographicalInformationSystemsTheory,ApplicationsandManagement
144