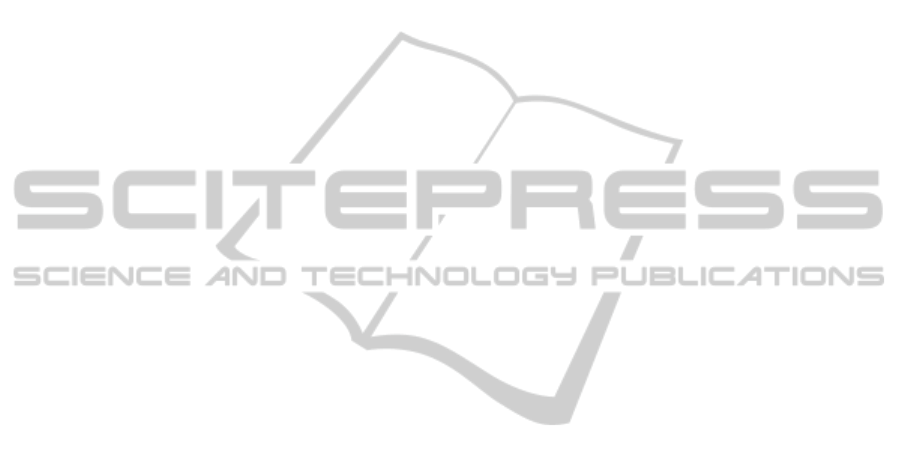
death decisions as well as ethical problems. If an
AI system makes a decision that we regret, then we
change their algorithms. If AI systems make
decisions that our society or our laws do not approve
of then we will modify the principles that govern
them or create better ones. Of course human beings
make mistakes and intelligent machines will make
mistakes too, even big mistakes. Like humans, we
need to keep watching over them, coaching and
improving them but a problem is that we don’t have
a agreement on what is acceptable.
There is a difference between intelligence and
decision-making. Intelligent machines can be very
useful but stupid machines can be scary. As for
human beings, Bishop has said that it is machine
stupidity that is dangerous and not machine
intelligence. A problem is that intelligent algorithms
can make many appropriate decisions and then
suddenly make a crazy one and flunk dramatically
because of an occurrence that did not appear in
training data. That is a problem with bounded
intelligence. But we should fear our own stupidity
more than the theoretical wisdom or foolishness of
algorithms yet to come. Ingham and Mollard have
said that AI machines have no emotions and never
will because they are not subject to the forces of
natural selection.
Kelly has said that there is no metric for
intelligence or benchmark for particular kinds of
learning and smartness and so it is difficult to know
if we are improving.
As AI systems make blunders then we can make
a decision about what is tolerable. Since AI is
taking on some tasks that humans do, we have a lot
to teach to them.
As humans, we only discern the real world
through a virtual model that we think of as reality.
Our memory is a neurological fabrication. Our
brains produce our stories and although they are
inaccurate, they are sufficient for us to stumble
along. We may be beaten on specific tasks but
overall, we tend to do admirably against machines.
Brockman has said that they are a long way from
replicating our flexibility, anger, fear, aggression,
and teamwork. While appreciating the limited,
chess playing talent of powerful computers, we
should not be unsettled by it. Intelligent machines
have helped us to become more skilful chess players.
As AI develops, we might have to engineer ways to
prevent consciousness in them just as we engineer
other systems to be safe. After all, even with Deep
Blue, anyone can pull its plug and beat it into rubble
with a sledgehammer (Provine, 2014).
REFERENCES
Bergasa-Suso, J., Sanders, d., Tewkesbury, G., 2005.
Intelligent browser-based systems to assist Internet
users. IEEE T EDUC 48 (4), pp. 580-585.
Brackenbury, I., Ravin, Y., 2002. Machine intelligence
and the Turing Test, IBM Syst Jrnl, Vol: 41 3, pp 524-
529.
Brooks, R., 2014. Artificial intelligence is a tool, not a
threat http://www.rethinkrobotics.com/artificial-
intelligence-tool-threat in rethinking robotics. Jan 15.
Berlinski, D., 2000. The Advent of the Algorithm,
Harcourt Books. ISBN 0-15-601391-6.Crevier, D.,
1993. AI: The Tumultuous Search for Artificial
Intelligence, New York, NY, USA: BasicBooks.
Chester, S., Tewkesbury, G., Sanders, D., et al 2006. New
electronic multi-media assessment system. 2nd Int
Conf on Web Info Sys and Tech, pp: 424 Year.
Chester, S., Tewkesbury, G., Sanders, D., et al 2007. New
electronic multi-media assessment system. Web Info
Systems and Technologies 1, pp 414-420.
Dreyfus, H., Dreyfus, S., 2008. From Socrates to Expert
Systems: The Limits and Dangers of Calculative
Rationality. WWW Pages of the Graduate School at
Berkeley 15 Jan 15. http://garnet.berkeley.edu.
Dyson, G., 2014. AI Brains Will be Analog Computers, Of
Course. Space Hippo. http://space-hippo.net/ai-
brains-analog-computers. http://space-hippo.net/ai-
brains-analog-computers. 15 Jan 15.
Gegov, A., Gobalakrishnan, N., Sanders, D., 2014b.
Filtration of non-monotonic rules for fuzzy rule base
compression. INT J COMPUT INT SYS 7 (2). pp.
382-400.
Gegov, A., Sanders, D., Vatchova, B., 2014a. Complexity
management methodology for fuzzy systems with
feedback rule bases. J INTELL FUZZY SYST 26 (1).
pp. 451-464.
Kandel, E., 2012 Principles of neural science ed: Kandel,
E., Schwartz, J., Jessell, T.,. Appleton and Lange:
McGraw Hill, pp. 338–343.
Kucera, V., 1997. Control Theory and Forty Years of
IFAC: A Personal View. IFAC Newsletter Special
Issue: 40th Anniversary of IFAC, Paper 5.
http://web.dit.upm.es. Accessed 15 Jan 15.
Kurzweil, R., 2005. The Singularity is Near, Penguin
Books. ISBN 0-670-03384-7.
Lanier, J., 2014. The Myth Of AI - A Conversation with J
Lanier. Edge. http://edge.org/conversation. Jan 15.
Masi, C., 2007. Fuzzy Neural Control Systems —
Explained. Control Engineering.
http://www.controleng.com. 15 Jan 15.
McCarthy, J., 2008. What Is Artificial Intelligence?"
Computer Science Department WWW Pages at
Stanford University. http://www-
formal.stanford.edu/jmc. Retrieved 14 Jan 15.
McCorduck, P., 2004, Machines Who Think: A Personal
Inquiry into the History and Prospects of Artificial
Intelligence, New York, AK Peters.
Muehlhauser, M., 2014. Three misconceptions in
Edge.org’s conversation on “The Myth of AI”.
ItIsArtificialIdiocyThatIsAlarming,NotArtificialIntelligence
349