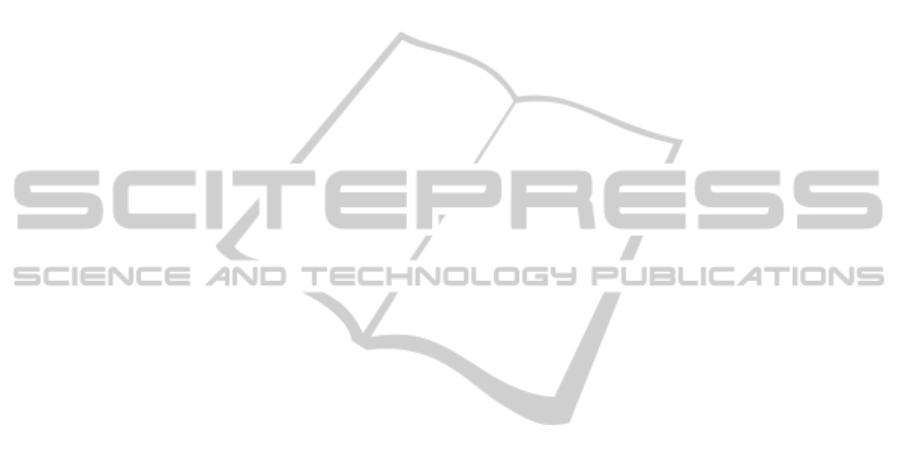
Comparison of the three methods based on sim-
ulation results has shown the following: 1) the QP
method allows to achieve the design objectives after a
small number of iterations, while both of the paramet-
ric and supervisory methods fail. 2) In the case when
a sharp decrease of pH occurs, the QP method is the
only one that ensures closed-loop systems stability. 3)
Application of the parametric and supervisory control
methods seem to produce important substrate concen-
tration fluctuations, in contrast to QP method. 4) Al-
though, due to the nature of the bioreactor, a certain
range of variations of the substrate concentration may
occur at the steady-state, the range of these variations
occurring by using the parametric and supervisory ap-
proaches is often not acceptable.
REFERENCES
A Cuzzola, F., C Geromel, J., and Morari, M. (2002). An
improved approach for constrained robust model pre-
dictive control. Automatica, 38(7):1183–1189.
Abate, A. and El Ghaoui, L. (2004). Robust model predic-
tive control through adjustable variables: an applica-
tion to path planning. In Decision and Control, 2004.
CDC. 43rd IEEE Conference on, volume 3, pages
2485–2490. IEEE.
Allwright, J. and Papavasiliou, G. (1992). On linear pro-
gramming and robust model-predictive control us-
ing impulse-responses. Systems & Control Letters,
18(2):159–164.
Ashoori, A., Moshiri, B., Khaki-Sedigh, A., and Bakhtiari,
M. R. (2009). Optimal control of a nonlinear fed-
batch fermentation process using model predictive ap-
proach. Journal of Process Control, 19(7):1162–1173.
Badgwell, T. A. (1997). A robust model predictive control
algorithm for stable linear plants. In American Control
Conference, 1997. Proceedings of the 1997, volume 3,
pages 1618–1622. IEEE.
Bemporad, A. (1998). A predictive controller with artificial
lyapunov function for linear systems with input/state
constraints. Automatica, 34(10):1255–1260.
Bemporad, A. and Garulli, A. (1997). Predictive control via
set-membership state estimation for constrained linear
systems with disturbances. In Proceedings of the 4th
European Control Conference.
Campo, P. J. and Morari, M. (1987). Robust model predic-
tive control. In American Control Conference, 1987,
pages 1021–1026. IEEE.
Freitas, C. and Teixeira, J. (1998). Hydrodynamic studies
in an airlift reactor with an enlarged degassing zone.
Bioprocess Engineering, 18(4):267–279.
Fukushima, H. and Bitmead, R. R. (2005). Robust con-
strained predictive control using comparison model.
Automatica, 41(1):97–106.
Gilbert, E. G. and Tan, K. T. (1991). Linear systems with
state and control constraints: The theory and appli-
cation of maximal output admissible sets. Automatic
Control, IEEE Transactions on, 36(9):1008–1020.
Grossmann, I. E., Halemane, K. P., and Swaney, R. E.
(1983). Optimization strategies for flexible chemi-
cal processes. Computers & Chemical Engineering,
7(4):439–462.
Keerthi, S. a. and Gilbert, E. G. (1988). Optimal infinite-
horizon feedback laws for a general class of con-
strained discrete-time systems: Stability and moving-
horizon approximations. Journal of optimization the-
ory and applications, 57(2):265–293.
Kerrigan, E. C. and Maciejowski, J. M. (2004). Feedback
min-max model predictive control using a single lin-
ear program: robust stability and the explicit solution.
International Journal of Robust and Nonlinear Con-
trol, 14(4):395–413.
Kothare, M. V., Balakrishnan, V., and Morari, M. (1996a).
Robust constrained model predictive control using lin-
ear matrix inequalities. Automatica, 32(10):1361–
1379.
Kothare, M. V., Balakrishnan, V., and Morari, M. (1996b).
Robust constrained model predictive control using lin-
ear matrix inequalities. Automatica, 32(10):1361–
1379.
Lee, J. a. and Yu, Z. (1997). Worst-case formulations of
model predictive control for systems with bounded pa-
rameters. Automatica, 33(5):763–781.
Lofberg, J. (2004). Yalmip: A toolbox for modeling and
optimization in matlab. In Computer Aided Control
Systems Design, 2004 IEEE International Symposium
on, pages 284–289. IEEE.
L
¨
ofberg, J. (2008). Modeling and solving uncertain opti-
mization problems in yalmip. In Proceedings of the
17th IFAC World Congress, pages 1337–1341.
Mailleret, L., Bernard, O., and Steyer, J.-P. (2004). Non-
linear adaptive control for bioreactors with unknown
kinetics. Automatica, 40(8):1379–1385.
Mayne, D. Q., Rakovi
´
c, S., Findeisen, R., and Allg
¨
ower,
F. (2006). Robust output feedback model predictive
control of constrained linear systems. Automatica,
42(7):1217–1222.
Mayne, D. Q. and Schroeder, W. (1997). Robust time-
optimal control of constrained linear systems. Auto-
matica, 33(12):2103–2118.
Parker, R. and Doyle, F. (1998). Nonlinear model predictive
control of a continuous bioreactor at near-optimum
conditions. In American Control Conference, 1998.
Proceedings of the 1998, volume 4, pages 2549–2553.
IEEE.
Rawlings, J. B. and Mayne, D. Q. (2009). Model predictive
control: Theory and design. Nob Hill Pub.
Rawlings, J. B. and Muske, K. R. (1993). The stability of
constrained receding horizon control. Automatic Con-
trol, IEEE Transactions on, 38(10):1512–1516.
Rubio, F. C., Garcia, J. L., Molina, E., and Chisti, Y. (2001).
Axial inhomogeneities in steady-state dissolved oxy-
gen in airlift bioreactors: predictive models. Chemical
Engineering Journal, 84(1):43–55.
AComparisonofRobustModelPredictiveControlTechniquesforaContinuousBioreactor
437