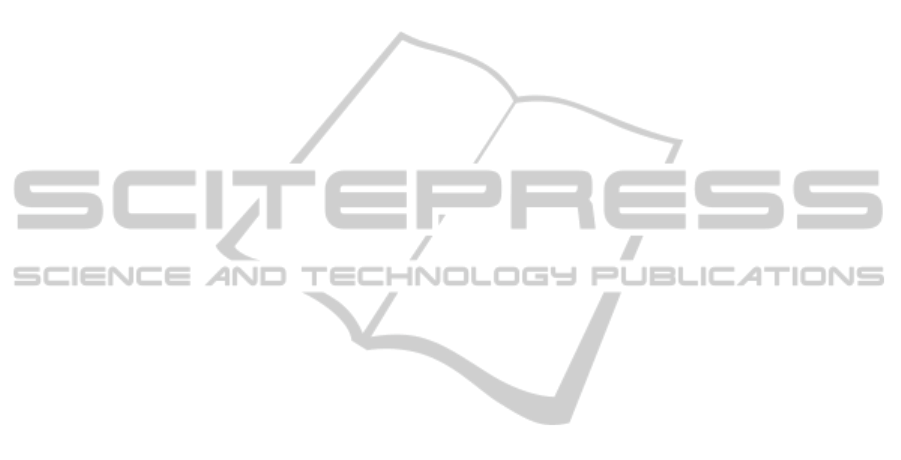
5 CONCLUSIONS
One possibility to launch this intelligent mobility rec-
ommendation system would be in combination with
a cloud backend and a mobile app. The system
logic and the algorithms would reside in the back-
end. Therefore it is possible to create thin mobile apps
for different mobile OS’ with no need for expensive
high-power CPUs in mobile phones. The mobile app
should be only a client for the cloud backend, which
is more like a GUI. The main task is to transmit infor-
mation for computation, receive the results as well as
notifications and visualize these information. Due to
this approach no additional devices are needed and the
acceptance barrier would be lowered. ”Yet another
app!” could be a barrier for the user, if this idea is not
going to be integrated into existing navigation apps.
Another app must be installed and configured (regis-
tration, settings etc.) before usage. Moreover privacy
is also a valid reason to be concerned to start using
this system. The user has to let the system record the
tracking information, compute mobility patterns and
store personal preferences and relations to other peo-
ple, who are connected with the user.
If the user is willing to let this happen the system
can support him to get a new experience about spare
time usage and socializing while traveling. Even in
reducing costs and saving the environment in case of
delayed or cancelled public transports by sharing non
public transport vehicle costs.
We also think about to use the described subsys-
tems in an inter modal navigation system. The focus
would be on detecting anomalies of different trans-
portation types, which should be used to arrive at the
destination point. The current time and the schedules
of the different transportation vehicles must be con-
tinuously observed and deviations have to be detected
in real time to warn the traveler or do a recalculation,
if there is a high risk to miss a connection. This pro-
vides a high potential for efficient route planning and
time saving especially in todays fast progression of
urbanization.
REFERENCES
AFP/woz (2010). Im winter durchschnittlich 114 zugaus-
flle pro tag bei der bahn. Hannoversche Allgemeine
Zeitung.
Bahn, D. (2014). Pnktlichkeitsentwicklung 2014.
Bilton, N. (2014). Tinder taps an age-old truth. The New
York Times, page E1.
BlaBlaCar (2012). Ridesharing statistics in europe.
Chan, N. D. and Shaheen, S. A. (2012). Ridesharing in
north america: Past, present, and future. Transport
Reviews, 32(1):93–112.
Froehlich, J. and Krumm, J. (2008). Route prediction from
trip observations. Technical report, SAE Technical Pa-
per.
Gonzalez, M. C., Hidalgo, C. A., and Barabasi, A.-L.
(2008). Understanding individual human mobility
patterns. Nature, 453(7196):779–782.
Herder, E., Siehndel, P., and Kawase, R. (2014). Predict-
ing user locations and trajectories. In User Model-
ing, Adaptation, and Personalization, pages 86–97.
Springer.
Lenhart, A. (2012). Teens, smartphones & texting. Pew
Internet & American Life Project.
Partzsch, Ina; Duerrschmidt, G. M. O. and Foerster, G.
(2012). Comparison of vehicle-based and smart-
phone generated acceleration data to determine mo-
tion states of passengers.
Patterson, D., Liao, L., Fox, D., and Kautz, H. (2003).
Inferring high-level behavior from low-level sensors.
In Dey, A., Schmidt, A., and McCarthy, J., editors,
UbiComp 2003: Ubiquitous Computing, volume 2864
of Lecture Notes in Computer Science, pages 73–89.
Springer Berlin Heidelberg.
Reddy, S., Burke, J., Estrin, D., Hansen, M., and Srivas-
tava, M. (2008). Determining transportation mode on
mobile phones. In Wearable Computers, 2008. ISWC
2008. 12th IEEE International Symposium on, pages
25–28.
Simmons, R., Browning, B., Zhang, Y., and Sadekar, V.
(2006). Learning to predict driver route and destina-
tion intent. In Intelligent Transportation Systems Con-
ference, 2006. ITSC’06. IEEE, pages 127–132. IEEE.
Song, C., Qu, Z., Blumm, N., and Barab
´
asi, A.-L. (2010).
Limits of predictability in human mobility. Science,
327(5968):1018–1021.
Statista (2014). Anzahl der smartphone-nutzer in deutsch-
land in den jahren 2009 bis 2014 (in millionen).
Townsend, D. J. and Bever, T. G. (2001). Sentence compre-
hension: The integration of habits and rules, volume
1950. MIT Press.
VEHITS2015-InternationalConferenceonVehicleTechnologyandIntelligentTransportSystems
118