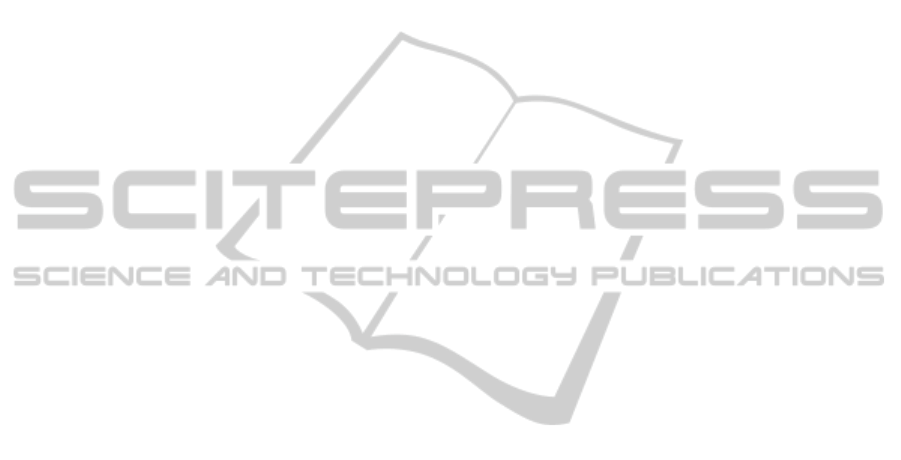
function’s plans are finalized, they are
communicated to other functions. However, during
execution of these plans, priorities of individual
functions do clash. Supply management may plan all
the material at once to reduce the ordering cost,
impacting the raw material inventory carrying cost.
Similarly, production may plan to manufacture the
maximum quantity so as to reduce cost related to
changeover, set-up etc. This increases the finished
good carrying cost. Also, it may deprive machine for
getting timely preventive maintenance leading to
catastrophic machine failure. Such failures calls for
prolonged corrective maintenance actions and also
affects quality of produced goods.
It is thus evident that performance of one
function severely affects performance of the others.
It therefore becomes imperative that the planning of
these functions must be carried out using an
integrated approach. Present paper aims to integrate
decisions related to supply management with that of
production, maintenance and inventory. In
particular, it aim to integrates supplier selection and
order quantity with shopfloor decisions like job
production sequence, manufacturing lot size and
preventive maintenance schedule.
2 LITERATURE REVIEW AND
PAPER CONTRIBUTION
Operations’ planning has gradually evolved from
optimization of individual parameters in a simplistic
environment to multi criteria optimization under
much complex environment. On scheduling, (Jones
et al., 1999) and (Chan et al., 2013), has
exhaustively reviewed the models developed for job
shop scheduling. Literature can also be found on
development of scheduling models for specific
objectives like minimizing job tardiness (Adamu and
Adewumi, 2014), or sequencing under uncertain
environment (Mula et al., 2006).
On the maintenance front the research expands
from maintenance optimization (Sharma et al., 2011)
to maintenance performance (Kumar et al., 2013).
Brief consolidation of the development in the field
of maintenance is mentioned in review by (Garg and
Deshmukh, 2006) for identifying the on-going trend
and future directions.
Concurrently, research has also progressed in the
field for supply management. Numerous decision
making approaches were proposed for optimizing
the decisions related to supplier selection as
mentioned in (Wilson, 1994). Review by (Aissaoui
et al., 2007) concentrates on mainly such models. It
proposes different classifications of the multiple
models which were published over the time.
Recent literature reflects that current focus of
researchers is towards the development of
“integrated” approaches (Hadidi et al., 2012).
There exist models which successfully integrate:
Production and Maintenance (Zhao et al., 2014)
Maintenance and Quality (Alfares et al., 2005)
Quality and Inventory (Peters et al., 1988)
Literature mentioned above demonstrates superiority
of integrated models compared to conventional
models. However, it can be noticed that all the
efforts for integration were confined mainly to
production, maintenance, inventory and quality. But,
there exists other equally critical functions beyond
those mentioned above, which also contributes
significantly towards the overall performance of
organization, but have been overlooked for
integration. Current work is an attempt to extend the
existing integrated models by incorporating one such
function namely Procurement Planning / Supply
Planning.
3 INDUSTRIAL PROBLEM
DISCRIPTION
The problem considers a multi component machine
as the central element of a small value chain with
customers at its one end and raw material suppliers
at the other end. Each customer can demand variety
of products in random quantity, to be delivered by
specific date. This demand needs to be processed on
a machine in a sequence which optimizes the total
cost of operations. To ensure the availability and
quality of output of machine, maintenance becomes
essential. Timely preventive maintenance is
performed in addition to corrective maintenance,
which is performed at the time of random machine
failure. Aligned with demand from each customer
and accounting for uncertainties, raw materials are
ordered from the set of previously screened
suppliers.
To elaborate further, consider a multi component
machine. Let the component be labelled as C
i
(0
,∈. The components are reliability wise
mutually independent and are in series. Time to
failure for each of these components follows two
parameter Weibull distributions, having shape
parameter and scale parameter as β
k
and η
k
respectively. These distribution parameters, along
with other factors, affect the stochastic failure of
SIMULTECH2015-5thInternationalConferenceonSimulationandModelingMethodologies,Technologiesand
Applications
56