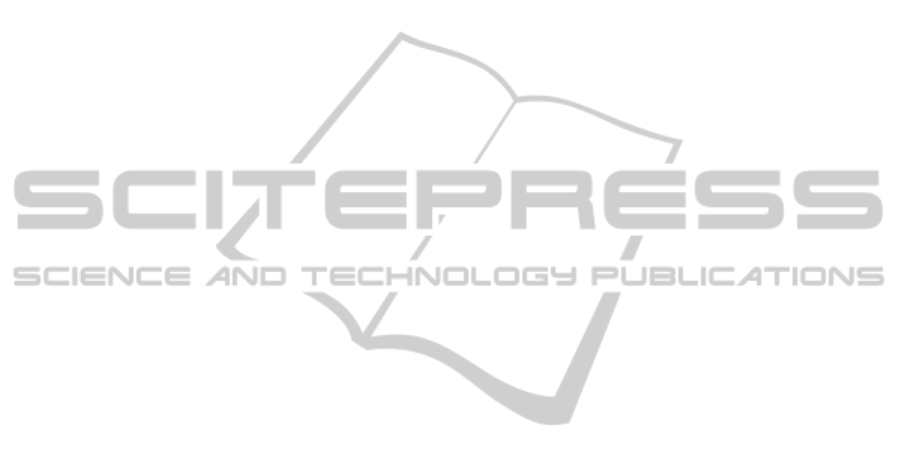
forecast about the upcoming market movements. In
return, agents can anticipate the changes in the future
investors’ actions and adjust their transaction
strategies to maximize profits.
The continuing refinement of the decision rules,
will see a replacement of the single stock trading
signaling mechanism with a multiple stock position
advising one. As a result, this model will have
practical values in the portfolio management as well.
This improved CAS model can be very helpful with
defining different parameters that best characterize
agents’ trading strategies, discovering and suggesting
suitable positions for different stocks at different
times, and discovering the factors affecting an
optimal portfolio management strategy. Finally,
agents in the future system will be categorized into
individual investors and institutional investors, as the
impact of their transactions differ in the real world.
Another version that allows agents to take
historical data for the training stage is under
development. By the end of the timeframe, agents
will use real-time data to conduct potential
transactions. We believe that agents will be able to
influence the market as we create a portfolio that trade
based on the agents’ signals. In return, agents will
change their trading behaviors corresponding to their
feedback from the market.
ACKNOWLEDGEMENTS
The authors thank the Complex Systems Institute
research group at UNC Charlotte for helpful
discussions, and the IT services at UNC Charlotte for
their provision of High Performance Clusters for our
research.
REFERENCES
Su, Z., Hadzikadic, M., 2014, 5
th
World Congress on Social
Simulation, Application of Complex Adaptive Systems
in Portfolio Management, WCSS 2014 Proceedings.
Tesfatsion, L., 2006. Handbook of Computational
Economics. Agent-based Computational Economics. A
Constructive Approach to Economic Theory. Vol. 2. pp.
831-880.
Johnson, L., Hadzikadic, M., Whitmeyer, J., 2013. The
Future Engaging Complexity and Policy: Afghanistan
Citizen Allegiance Model. International Journal of
Humanities and Social Science. Vol.3. No.10.
Dreau, D. Stanimirov, D.; Carmichael, T.; Hadzikadic, M.
2009, An agent-based model of solid tumor progression.
Bioinformatics and Computational Biology. Vol. 5462.
Holland, J., 1975. Adaption in Natural and Artificial
Systems.
Wilensky, U., 1999, Netlogo, Centre for Connected
Learning and Computer-Based Modeling.
Northwestern University, Evanston, IL.
Fishman, G., 1995. Monte Carlo: Concepts, Algorithms,
and Applications. Springer.
Faber, M., 2010. Relative Strength Strategies for Investing.
Cambria.
Barnes, J. 2003. Active vs. Passive Investing. CFA
Magazine. Jan 2003: 28-30.
Scowcroft, A., and Sefton, J., 2005. Understanding
momentum. Financial Analysts Journal, 64-82.
Johnson, N., Jefferies, P., and Hui, P., 2003. Financial
market complexity. OUP Catalogue.
Cappiello, L., Engle, R., and Sheppard, K., 2006.
Asymmetric dynamics in the correlations of global
equity and bond returns. Journal of Financial
econometrics, 4(4), 537-572.
Raberto, M., Cincotti, S., Focardi, S., & Marchesi, M., 2001.
Agent-based simulation of a financial market. Physica
A: Statistical Mechanics and its Applications, 299(1),
319-327.
Bonabeau, E., 2002. Agent-based modeling: Methods and
techniques for simulating human systems. Proceedings
of the National Academy of Sciences of the United
States of America, 99(Suppl 3), 7280-7287.
Hadzikadic, M., Carmichael, T., and Curtin, C., 2010.
Complex adaptive systems and game theory: An
unlikely union. Complexity, 16(1), 34-42.
Kodia, Z., Said, L., and Ghedira, K., 2010. A study of stock
market trading behavior and social interactions
through a multi agent based simulation. Agent and
Multi-Agent Systems: Technologies and Applications
(pp. 302-311). Springer Berlin Heidelberg.
Subramanian, H., Ramamoorthy, S., Stone, P., and Kuipers,
B., 2006. Designing safe, profitable automated stock
trading agents using evolutionary algorithms.
Proceedings of the 8th annual conference on Genetic
and evolutionary computation (pp. 1777-1784). ACM.
Saad, E., Prokhorov, D., and Wunsch, D., 1998.
Comparative study of stock trend prediction using time
delay, recurrent and probabilistic neural networks.
Neural Networks, IEEE Transactions on, 9(6), 1456-
1470.
Teixeira, L., and De Oliveira, A., 2010. A method for
automatic stock trading combining technical analysis
and nearest neighbor classification. Expert systems
with applications, 37(10), 6885-6890.
Farmer, J., Patelli, P., and Zovko, I., 2005. The predictive
power of zero intelligence in financial markets.
Proceedings of the National Academy of Sciences of
the United States of America, 102(6), 2254-2259.
Darley, V., and Outkin, A., 2007. NASDAQ market
simulation: insights on a major market from the science
of complex adaptive systems. World Scientific
Publishing Co., Inc.
SIMULTECH2015-5thInternationalConferenceonSimulationandModelingMethodologies,Technologiesand
Applications
358