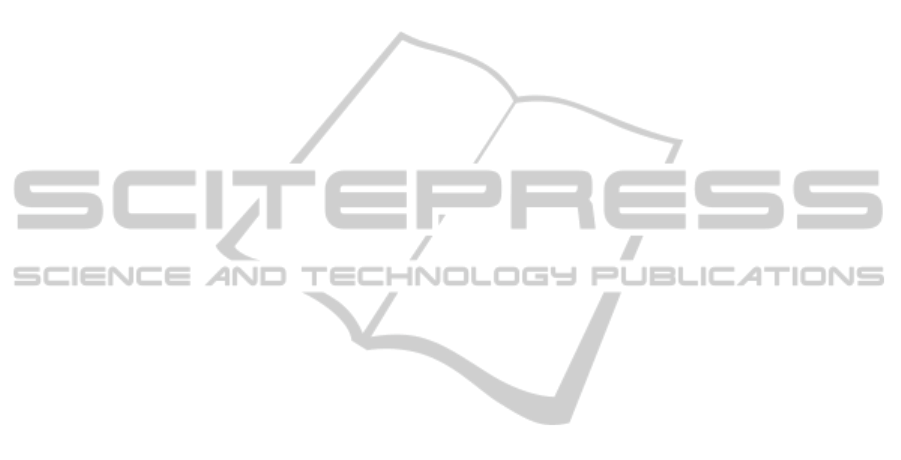
same position accuracy (see Figure 3a) and good con-
sistency (see Figure 3c). From Figure 3b, we can see
that both the GMM-ITS and the CbGMF have signifi-
cantly smaller range errors in the early states than the
MCAEKF.
The target starts from [1050,2500] km (the long
range) in scenario 2. In the GMM-ITS, the measure-
ment likelihood is approximated by N = 12 compo-
nents with α = 3. In this scenario, as shownin Figures
4a, 4b and 4c, the GMM-ITS and the CbGMF have
obviously improved accuracy in position and range
over the MCAEKF and do not exhibit loss in range
accuracy in the early stage of filtering. We can clearly
see that the GMM-ITS and the MCAEKF are consis-
tent in Figure 4c; however, in this case, the CbGMF
has a small degradation in consistency (the NEES is
around 5 instead of 4) (Tian and Bar-Shalom, 2014).
In scenario 3 and 4, the target starts much further
away from [4500,2200] km (the very long range) and
[8500,5500] km (the extremely long range), respec-
tively. Obviously, the contact lens issue is much more
of a problem than in scenario 1 and 2. In scenario
3, the parameters of the GMM-ITS are N = 24 and
α = 3.5. In order to guarantee the consistency of the
GMM-ITS, N = 48 and α = 4 are chosen in scenario
4. Figures 5a and 6a show that the GMM-ITS per-
forms better than the CbGMF and the MCAEKF in
the position RMSE. Furthermore, as shown in Figure
5c and Figure 6c, the GMM-ITS and the MCAEKF
are consistent, but the CbGMF is not.
5 CONCLUSIONS
For very long range target tracking, traditional filters
such as the EKF and the UKF are ill-equipped to solve
the contact lens problem. However, the MCAEKF
maintains consistency by using a bigger standard de-
viation of the range measurement. As a result, the
MCAEKF exhibits significant loss in range accuracy
in the early stage of filtering. The CbGMF represents
the distribution of the target state by a dynamic set
of Gaussian mixtures and can avoid the problem the
MCAEKF suffers. However, the CbGMF is consis-
tent only in the small range scenario. In the GMM-
ITS, both the measurement likelihood and the target
state pdf are approximated by a set of Gaussian mix-
tures. As shown in the simulation experiments, the
GMM-ITS is always consistent in different range sce-
narios and has small errors in positon and range. To
best of our knowledge, no other Gaussian mixture ap-
proach thus far guarantees sufficient consistency and
tracking accuracy, which are crucial to filter perfor-
mance.
ACKNOWLEDGEMENTS
This work was supported by Defense Acquisition Pro-
gram Administration and Agency for Defense Devel-
opment, Korea under the contract UD140081CD.
REFERENCES
Bar-Shalom, Y., Li, X.-R., and Kirubarajan, T. (2001). Esti-
mation with Application to Tracking and Navigation.
John Wiley and Sons.
Blackman, S. and Popoli, R. (1999). Design and Analysis
of Modern Tracking Systems. Artech House.
Julier, S. J. and Uhlmann, J. K. (2004). Unscented filtering
and nonlinear estimation. Proceedings of the IEEE,
92(3):401–422.
Longbin, M., Ziaoquan, S., Yiyu, Z., and Bar-Shalom, Y.
(1998). Unbiased converted measurements for track-
ing. IEEE Transactions on Aerospace and Electronic
Systems, 34(3):1023–1027.
Muˇsicki, D. (2009). Bearings only single-sensor tar-
get tracking using gaussian mixtures. Automatica,
45(9):2088–2092.
Muˇsicki, D. and Evans, R. (2006). Measurement gaussian
sum mixture target tracking. In 9th International Con-
ference on Information Fusion, Florence, Italy.
Ristic, B., Arulampalam, S., and Gordon, N. (2004). Be-
yond the Kalman Filter. Artech House.
Singer, R. A., Sea, R., and Housewright, K. B. (1974).
Derivation and evaluation of improved tracking fil-
ters for use in dense multi-target environments. IEEE
Transactions on Information Theory, 20(4):423–432.
Tian, X. and Bar-Shalom, Y. (2009). Coordinate conver-
sion and tracking for very long range radars. IEEE
Transactions on Aerospace and Electronic Systems,
45(3):1073–1088.
Tian, X. and Bar-Shalom, Y. (2014). A consistency-based
gaussian mixture filtering approach for the contact
lens problem. IEEE Transactions on Aerospace and
Electronic Systems, 50(3):1636–1646.
ICINCO2015-12thInternationalConferenceonInformaticsinControl,AutomationandRobotics
464