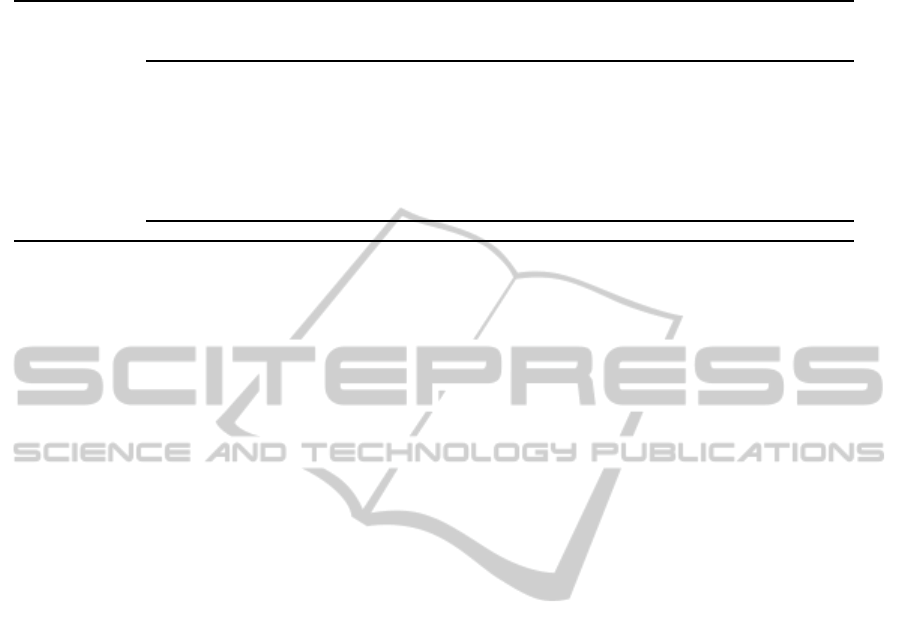
Table 1: Criteria and its sub-criteria description related to the data quality dimensions.
Criteria Sub-Criteria Description Type
Believability (C
B
)
Length of Work Description (C
B1
) Length of the work description related to a work order. I
c
avg
(i)
Work Log Variation (C
B2
) Work Description variation among the different operator reports I
c
sim
(i)
Technician Log Variation (C
B3
) Technical log variation among the different operator reports I
c
sim
(i)
Completeness (C
C
)
Asset Location reported (C
C1
) Location of asset within product where maintenance has been done. I
c
sim
(i)
Description reported (C
C2
) Description of work to be done in particular maintenance work. I
c
sim
(i)
Actual Finish Date reported (C
C3
) Actual Finish date and time of work completed. I
c
sim
(i)
Target Start Date reported (C
C4
) Targeted start date of the maintenance work. I
c
sim
(i)
Target Finish Date reported (C
C5
) Targeted finish date of the maintenance work. I
c
sim
(i)
DLC Code reported (C
C6
) Actual location of the defect within product. I
c
sim
(i)
Schedule Start Date reported (C
C7
) Scheduled start date of the maintenance work. I
c
sim
(i)
Schedule Finish Date reported (C
C8
) Scheduled Finish date of the maintenance work. I
c
sim
(i)
Timeliness (C
T
) This is average delay of reporting on individual site I
c
avg
(i)
– Domain Appropriateness;
– Participant Knowledge Appropriateness;
– Technical Actor Interpretation Enhancement.
2.2 Krogstie’s Framework Adaptation
Given the above definitions, and based on the
OEM company’s requirements, three key con-
cepts/relationships and one assumption lay the
groundwork of our study for Krogstie’s framework
adaptation. First, the study assumption is that the
Physical Quality (cf. Figure 1), and particularly the
externalized model, is 100% persistent and available,
thus enabling participants to make sense of it. In-
deed, the OEM company designed its own mainte-
nance models, report templates, databases, etc., and
is not willing (at a first stage) to assess/study how
persistent their implementations are compared with
the initial expert statements, expressed knowledge,
etc. The OEM company then expressed require-
ments regarding three of the Krogstie’s framework
concepts/relationships, namely:
1. Semantic Quality: one of the OEM company’s re-
quirement matches – to a certain extent – with
the semantic quality dimension since the company
would like to know to which extent the service
data reported by each operator (on each site) can
be trusted, or more exactly can be considered as
“true”, “real” and “credible”, in order to carry out
the planning activities. This is referred to as the
“Believability” criterion (C
B
) in this paper, whose
various facets of the Believability are formalized
in the form of sub-criteria (or Believability quality
indicators) denoted by {C
B1
..C
B3
} in Table 1;
2. Language Quality: one of the OEM company’s
requirement matches – to a certain extent – with
the language quality dimension since the com-
pany would like to know to which extent the ser-
vice data reported by each operator is complete,
or is of sufficient depth and breadth for the task at
hand (Wang and Strong, 1996). To put it another
way, this criterion, referred to as Completeness
(C
C
), reflects the level of details reported by each
operator with regard to each report field that needs
to be entered (in accordance with the company’s
business logic) in the report. Similarly to C
B
, the
facets of Completeness are denoted {C
C1
...C
C8
}
(see Table 1);
3. Knowledge Quality: one of the OEM company’s
requirement matches – to a certain extent – with
the semantic quality dimension since the company
would like to know to which extent the service
data reported by each operator is sufficiently “up
to date”, which is depending on the time differ-
ence between the maintenance work and the work
reporting. This criterion, referred to as Timeliness
C
T
, is based on the assumption that the longer
the time spent to submit the report, the lesser the
quality of the reporting (operator are likely to for-
get key details of the maintenance task over time).
No sub-criterion is defined for this dimension, as
shown in Table 1 (C
T
);
In order to ease the understanding of these three
data quality dimensions, and associated sub-criteria,
we propose to illustrate through Figure 2 the differ-
ent stages that compose our adapted framework. This
figure highlights that maintenance operators carry out
maintenance work/tasks on each OEM site (sites de-
noted by Site 1... Site z) and generate multiple re-
ports. A zoom on reports from Site 1 and n is pro-
posed in Figure 2 so as to compare both sets of re-
ports based on the criteria defined in Table 1. It al-
lows for an understanding of when a report, or field
content, impacts positively on the company’s mainte-
nance reporting quality, and when it does impact neg-
atively (see “smileys” and associated explanation in
Figure 2).
In this paper, a simple and effective MCDM tech-
nique is used as support of the arithmetic framework
to handle the integration/aggregation of the various
DATA2015-4thInternationalConferenceonDataManagementTechnologiesandApplications
164