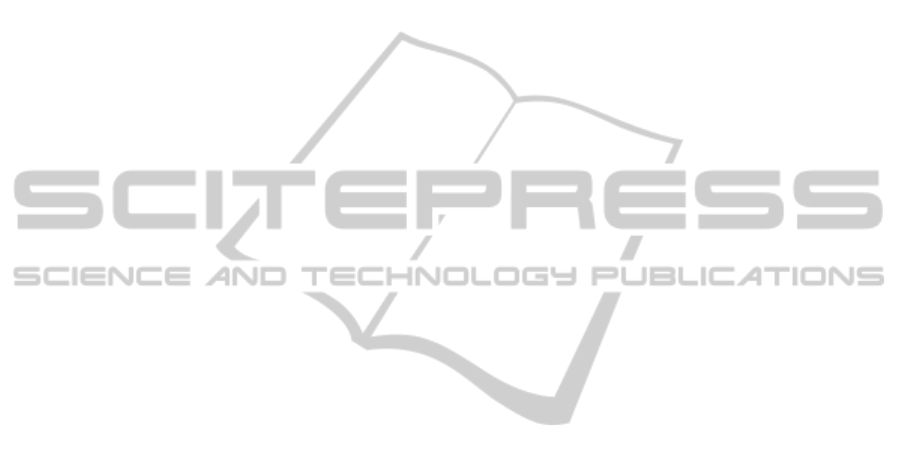
equipment, material, and environmental factors that
could cause accidents, which may affect productivity
and profitability as well as injuries. It is also a
complex task that requires knowledge of both
regulations and experience because of the dynamic
nature of construction environments. Goh and Chua
(2009) indicated that safety experience may assist
workers in improving their safety performance and
preventing recurring mistakes, and those poor
hazard-identification processes are the result of a
lack of experience.
Several studies have successfully employed eye-
tracking devices to evaluate the difference between
the visual search patterns that experienced and
novice drivers employ to identify road hazards
(Hosking et al., 2010). They showed that novice
drivers employ visual strategies that differ from
those used by experienced drivers (Falkmer and
Gregersen, 2005). Furthermore, under risky driving
conditions, the scanning behaviour of novice drivers
is narrower than that of experienced drivers;
moreover, novice drivers typically look directly
ahead, and they fail to perceive and assess hazard
information (Pradhan et al., 2005). Konstantopoulos
et al., (2010) reported that because experienced
drivers are more familiar with hazardous events than
novice drivers are, their fixations are shorter and
more frequent. The poor hazard-identification ability
or risk perception of novice drivers might explain
why novice drivers are involved in more accidents
(Ciceri and Ruscio, 2014).
2 EXPERIMENT DESIGN
To extract the difference between the hazard-
identification ability of experienced workers and that
of novice workers, we prepared four snapshots from
the 3D virtual construction site model that was
developed specifically for this research. The virtual
model allows the experiment to accommodate a
variety of hazards that are infeasible to see on a
single construction site in real life as most
construction sites would already corrected hazards if
they allow us to conduct the experiment there. The
model contains various hazards that were identified
as the most frequently occured hazards in the
construction industry (MOL, 2014). The participants
were presented with the images sequentially, and
they were asked to identify potential hazards. The
identification accuracy and time were recorded for
further analysis. An eye-tracker was used to record
each participant’s fixation. Each participant was
interviewed following the experiment.
We collected cases of 350 construction industry
accidents in Taiwan from 2009 to 2011 (MOL,
2014). We limited our scope to building construction,
and identified 178 accidents fitting that category.
We selected 14 hazards of 6 accident types,
including falls, collapses, electric shocks, lacerations,
explosions, and unsafe actions.
We used Google SketchUp version 8 (Trimble
Navigation, Ltd) (Trimble Navigation, 2014) to
create a virtual three-story building construction site
featuring 8 workplaces that contain 21 hazards.
Among those hazards, snapshots of 14 hazards, as
shown in Fig. 1 (e.g., H1-1, H1-2, and H1-3 in
Workplace 1) located in four workplaces were taken
to be used as the test hazards in this experiment.
Table 1 details the hazards.
Twenty-five paid volunteers participated in the
experiment. Ten participants were experienced
construction workers with an average of 5 years
working experience and 6 hours formal safety
training, which is required annually by the
regulation, and 15 were graduate students studying
construction engineering and management at
National Chiao-Tung University, Taiwan, who had
no work experience and safety training in
construction. The construction workers represented
experienced workers, whereas the graduate students
represented novice workers. All participants had
normal or corrected-to-normal vision, and passed an
eye-tracking calibration test.
A 19-inch liquid-crystal display (LCD) monitor
with a resolution of 1280 × 1024 pixels was
connected to a laptop to display the images. We
recorded eye movement by using the EyeFrame
SceneCamera System model of the ViewPoint
EyeTracker, manufactured by Arrington Research,
Inc. (Arrington Research, 2014) (Figs. 2-1 and 2-2)
at a sampling rate of 30/60 Hz, spatial resolution of
0.15 degrees of the visual arc, and accuracy between
0.25 and 1.00 degrees of the visual arc.
The experiment facilitator assisted each
participant in fitting and calibrating the eye-tracker.
Subsequently, the participant started the experiment
by inspecting the snapshots and using a mouse to
identify potential hazards sequentially from
Workplaces 1 to 4 without time limitations, and
without knowing the total number of target hazards.
A successful identification was recorded only when
a participant clicked on a hazard and correctly
explained the reason for the spot to be a hazard. The
participants’ head and eye movement were
unrestricted during the experiment. An interview
followed the experiment to clarify how the
ICSOFT-EA2015-10thInternationalConferenceonSoftwareEngineeringandApplications
368