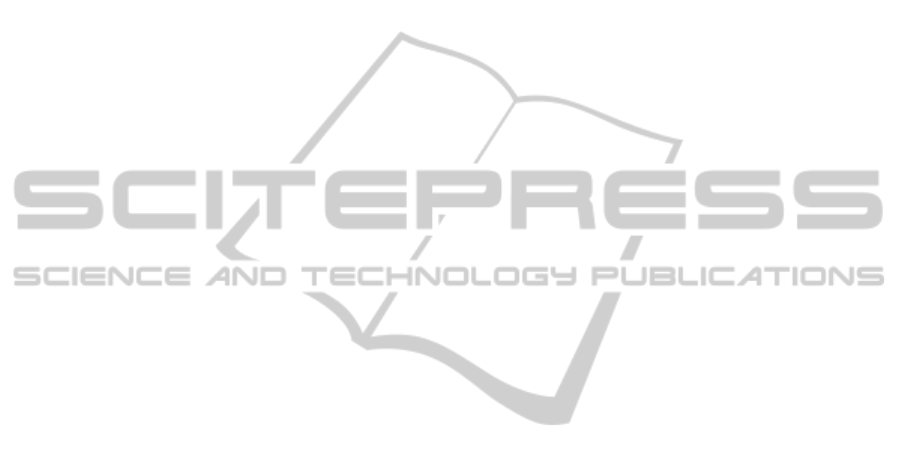
overload” was mention by (Toffler, 1970) to explain
the difficulties associated with decision making due
the presence of excessive information. After that, a
concern for the management and interpretation of
large volumes of data became more relevant;
however, without a proper solution.
When computer systems were developed enough
for recognize or predict patterns on data (Denning,
1990), the scientific community was able to describe
with more details the properties of the BD. The first
known scientists who conceptualized the term were
(Cox and Ellsworth, 1997) and they described it as
the large data sets which exceed the capacities of
main memory, local disk, and even remote disk.
Consequently, the term was mainly associated with
the size or volume of the data but (Laney, 2001)
proposed two additional properties for describe BD
calling them: variety, for refer to the diversity of
data types and; velocity, for indicate the production
rate of data. This concept approach is called the
three V´s of BD and nowadays inspires most of the
BD management strategies. However, other authors
as (Assunção et al., 2013) suggest additional V´s
properties and considerations for BD management
calling them: veracity, value, visualization and
vulnerability.
Regarding to Geospatial data as BD, its use
constitutes a research frontier which is making
conventional processing and spatial data analysis
methods no longer viable. The increasingly data
collection and complexity of sensors aboard the
earth observation satellites and other technology
devices based in Geospatial data production is
nowadays demanding new platforms for processing,
which are now accessible through cloud computing
services (Sultan, 2010). However, the scientific
literature related to this field is not so numerous than
the research done over individual or small
collections of satellite images using conventional
computing methods. A decline on this tendency over
time is concluded by (Hansen et al., 2012), who
affirms that methods in the future will evolve and
adapt to greater data volumes and processing
capabilities; and (Gray, 2009) who anticipated a
revolution of scientific exploration based on data-
intensive and high-performance computing
resources.
The release by NASA and the USGS of a new
Landsat Data Distribution Policy (National
Geospatial Advisory Committee, 2012) which
enables the free download of the whole available
data collection constitutes an example of a data-
intensive source which demands new approaches for
extract meaningful information. In this sense,
(Potapov et al., 2012) demonstrated the feasibility to
work with large Landsat collections developing a
methodology which enable the quantification of
forest cover loss through the analysis of a set of
8,881 images and a decision tree change detection
model. Moreover, (Flood et al., 2013) proposed an
operational scheme for process a standardized
surface reflectance product for 45,000 Landsat
TM/ETM+ and 2,500 SPOT HRG scenes,
developing an innovative procedure for correct the
atmosphere, bidirectional reflectance and
topographic variability between scenes. However, in
both cases, is unknown the computing strategies
adopted for manage and process such large
collection of images.
Nonetheless, other authors describe with detail
the use of High Performance Computing and
Geospatial data. For instance, (Wang et al., 2011)
develop a prototype of a scientific Cloud computing
project applied in remote sensing, which describes
the requirements and organization of the resources
needed; (Almeer, 2012; Beyene, 2011) investigated
the MapReduce programming paradigm for process
large collection of images; and (Christophe et al.,
2010) describes some benefits of Graphical
processing units (GPU) respect to Multicore Central
Processing Units (CPU) on the processing time of
different algorithms types, commonly used in remote
sensing.
From all the references consulted, these two
approaches were mainly found, in other words,
separating the design of the remote sensing
processing chains from the BD management
strategies. For this reason, this research aims to
couple them on two specific cases of large
Geospatial data collections applied on Ecosystem
monitoring, which involve the design of processing
chains and BD management strategies.
4 METHODOLOGY
As is mention in the section 2, the empirical research
will be applied in two cases, therefore each research
objective has their own specific data sources,
analysis methods, validation procedures and study
areas (except for the third one which is mainly
theoretical). The materials and methods are
summarized in the next paragraphs (subsections 4.1
to 4.4):
Data sources: multispectral and radar remote
sensing products, aerial photography, ancillary
cartography, climatic databases, GPS inventories,
field recognition and surveys.
CLOSER2015-DoctoralConsortium
4