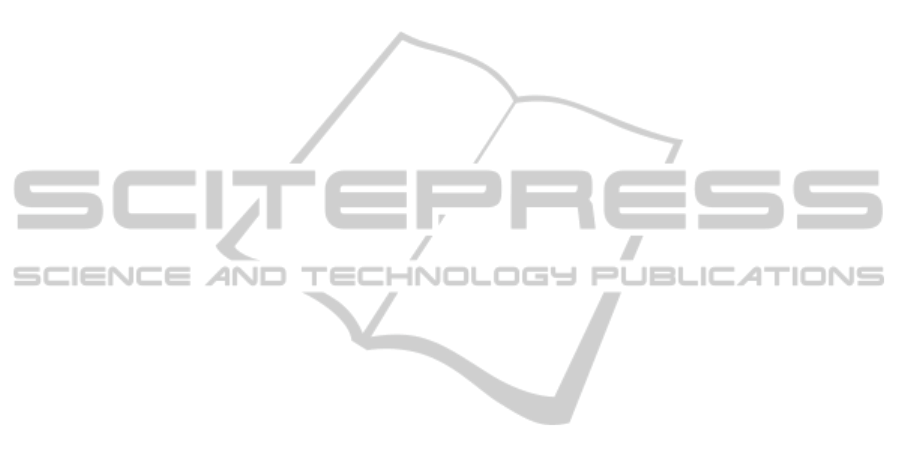
problem with multiple classifiers and the proposal of
a robust Fongbe phoneme classification system which
incorporates a fusion of Naive Bayes and LVQ classi-
fiers using fuzzy logic approach. This proposal builds
on the performance achieved by our fuzzy logic based
approach compared to DBN based approach and es-
pecially because of the limitations of the fixed thresh-
old value in weighted combination. The future of this
work is an automatic continuous speech recognition
from phonetic segmentation in Fongbe language.
ACKNOWLEDGEMENTS
This work is partially supported by Association AS2V
and the Fondation Jacques De Rette, France. The
authors appreciate the help of Jonas DOUMATE for
proof-reading the paper. Fr
´
ejus A. A. LALEYE is
also grateful to Agence Universitaire de la Franco-
phonie (AUF).
REFERENCES
A. Metallinou, S. L. and Narayanan., S. (2010). Decision
level combination of multiple modalities for recogni-
tion and analysis of emotional expression. In IEEE In-
ternational Conference on Acoustics Speech and Sig-
nal Processing (ICASSP), pages 2462–24665.
Ager, M., Cvetkovic, Z., and Sollich, P. (2013). Phoneme
Classification in High-Dimensional Linear Feature
Domains. Computing Research Repository.
Agoli-Agbo, E. O. and Bernard, C. (2009). Les particules
nonciatives du fon. Institut national des langues et
civilisations orientales, Paris, 1st edition.
Akoha., A. B. (2010). Syntaxe et lexicologie du fon-gbe:
B
´
enin. Ed. L’harmattan, page 368.
Bengio, Y., P., L., D., P., and H., L. (2006). Greedy layer-
wise training of deep networks. In Advances in Neural
Information Processing Systems.
Borne, P., Benrejeb, M., and Haggege., J. (2007). Les
rseaux de neurones, pr
´
esentation et applications.
TECHNIP Editions, page 90.
Cho, S.-B. and Kim., J. (1995). Combining multiple neural
networks by fuzzy integral and robust classification.
IEEE Transactions on Systems, Man, and Cybernet-
ics, pages 380–384.
Corradini, A., Mehta, M., Bernsen, N., Martin, J., and
Abrilian., S. (2003). Multimodal input fusion in hu-
mancomputer interaction. In NATO-ASI Conference
on Data Fusion for Situation Monitoring, Incident De-
tection, Alert and Response Management.
Esposito, A., Ezin, E., and Ceccarelli, M. (1996). Prepro-
cessing and neural classification of english stop con-
sonants [b, d, g, p, t, k]. In The 4th International Con-
ference on Spoken Language Processing, pages 1249–
1252, Philadelphia.
Esposito, A., Ezin, E., and Ceccarelli, M. (1998). Phoneme
classification using a rasta-plp preprocessing algo-
rithm and a time delay neural network : Performance
studies. In Proceedings of the 10th Italian Workshop
on Neural Nets, pages 207–217, Salerno,.
Foucher, S., Laliberte, F., Boulianne, G., and Gagnon., L.
(2006). A dempster-shafer based fusion approach for
audio-visual speech recognition with application to
large vocabulary french speech. In IEEE International
Conference on Acoustics, Speech and Signal Process-
ing, volume 1.
Genussov, M., Lavner, Y., and Cohen, I. (2010). Classifica-
tion of unvoiced fricative phonemes using geometric
methods. In 12th International Workshop on Acoustic
Echo and Noise Control. Tel-Aviv, Israel.
Hinton, G., S., O., and Teh, Y. (2006). A fast learning algo-
rithm for deep belief nets. Neural Comput, 18:1527–
1554.
Iyengar, G., Nock, H., and Neti., C. (2003). Audio-
visual synchrony for detection of monologue in video
archives. In IEEE International Conference on Multi-
media and Expo, volume 1, pages 329–332.
Jacobs., R. (1995). Methods for combining experts’s prob-
ability assessments. Neural Computation, pages 867–
888.
Jacobs, R., Jordan, M., Nowlan, S., and Hinton., G. (1991).
Adaptive mixture of local experts. Neural Computa-
tion, pages 79–87.
Kittler, J., Hatef, M., Duin, R., and Matas., J. (1998). On
combining classifiers. IEEE Transactions on Patterns
Analysis and Machine Intelligence, pages 226–239.
Kohonen., T. (1988). An introduction to neural computing.
Neural Networks, 1:3–16.
LALEYE, F. A. A., EZIN, E. C., and MOTAMED, C.
(2014). Weighted combination of naive bayes and lvq
classifier for fongbe phoneme classification. In Tenth
International Conference on Signal-Image Technol-
ogy & Internet-Based Systems, pages 7 – 13, Mar-
rakech. IEEE.
Le, V.-B. and L, B. (2009). Automatic speech recognition
for under-resourced languages: Application to viet-
namese language. In IEEE Transactions on Audio,
Speech, and Language Processing, pages 1471–1482.
IEEE.
Lefebvre, C. and Brousseau., A. (2001). A grammar of
fonge, de gruyter mouton. page 608.
Lewis, T. W. and Powers., D. M. (2001). Improved speech
recognition using adaptive audio-visual fusion via a
stochastic secondary classifier. International Sym-
posium on Intelligent Multimedia, Video and Speech
Processing, 1:551–554.
Lung, J. W. J., Salam, M. S. H., Amjad Rehman, M. S.
M. R., and Saba, T. (2014). Fuzzy Phoneme Classi-
fication Using Multi-speaker Vocal Tract Length Nor-
malization. IETE Technical Review, London, 2nd edi-
tion.
Malcangi, M., Ouazzane, K., and Patel, K. (2013). Audio-
visual fuzzy fusion for robust speech recognition. In
The 2013 International Joint Conference on Neural
Networks (IJCNN), pages 1 – 8, Dallas. IEEE.
AdaptiveDecision-levelFusionforFongbePhonemeClassificationusingFuzzyLogicandDeepBeliefNetworks
23