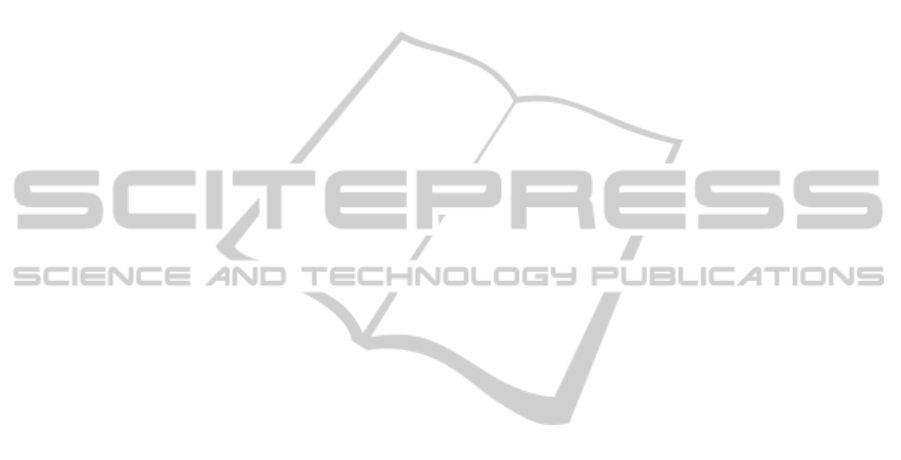
It has been observed from Figure 2, Figure 3
and Figure 4 that the performance of ADDIF is
substantially superior to that of non adaptive
DDIF as the RMSE for all three states
converged to a lower steady state value within
comparatively less time. RMSE of non
adaptive DDIF deteriorates as the Q remains
unknown due to unknown turn rate.
It is to be also pointed out that though the
elements of Q related to position and velocity
are known RMSE of position and velocity for
the non adaptive DDIF is degraded because of
the implicit influence of poorly estimated turn
rate.
Figure 5 indicates that for ADDIF the unknown
process noise element is converged to the truth
value in about 30 sec.
The RMSE results of ADDIF are also
compared with non adaptive DDIF in the ideal
situation when q is known only to the latter.
Though this comparison may sound unusual,
this comparison illumines on how far the
performance ADDIF even with unknown Q is
close to the performance of traditional filter in
ideal situation with known Q. It is
demonstrated that the RMSE of ADDIF for all
the states are very closed to that nature of
RMSE of non adaptive filter in ideal condition.
The initial mismatch in RMSE is because of
the time taken for adapted Q to converge.
It is also found from the Monte Carlo
simulation that the track loss cases cannot be
ruled out even for the ideal situation when the
non adaptive DDIF has the knowledge of Q. In
the MC simulation 1.7% of track loss has been
observed for the ideal case. When Q is
unknown, the percentage of track loss for
ADDIF is 2.2% and that for non adaptive
DDIF is 15%. The track loss percentage for
ADDIF is comparable with the ideal case and
substantially low compared its non adaptive
version which is prone to track loss cases.
These observations indicate the superiority of
ADDIF over non adaptive DDIF when Q remains
unknown for parametric uncertainties.
4 CONCLUSIONS
An Adaptive Divided Difference Information filter
has been proposed for multiple sensor fusion in face
of unknown parameter variation and exemplified
with the help of an aircraft tracking problem. The
proposed filter is found to carry out multiple sensor
estimation successfully by online adaptation of
process noise covariance (Q) where the knowledge
of Q remains unavailable due to parametric
uncertainty. The adapted Q from the filter converges
on the true value of Q and continues to track it for
subsequent time. The results from Monte Carlo
study indicate that the RMS error performance of the
proposed filter, as expected, is significantly superior
to the non adaptive Divided Difference Information
filter in face of unknown Q. Because of the
capability of adaptation, flexibility for multiple
sensor estimation and good error settling
performance the proposed filter may be a
recommended for multiple sensor fusion for the
systems affected by unknown parameter variation.
ACKNOWLEDGEMENTS
The First author thanks Council of Scientific &
Industrial Research (CSIR), New Delhi, India for
financial support and expresses his gratitude to
Centre for Knowledge Based System, Jadavpur
University, Kolkata, India for infrastructural
support.
REFERENCES
T. Vercauteren, and X. Wang., 2005. Decentralized sigma-
point information filters for target tracking in
collaborative sensor networks. In IEEE Trans. Signal
Processing
, 53 (8), 2997-3009.
D. J. Lee, 2008. Nonlinear estimation and multiple sensor
fusion using unscented information filtering. In
IEEE
Signal Processing Letters
, 15, 861-864.
B. Jia, M. Xin, K. Pham, E. Blasch, & G. Chen, 2013.
Multiple sensor estimation using a high-degree
cubature information filter. In conference
SPIE
Defense, Security, and Sensing. International Society
for Optics and Photonics
, 87390T-87390T.
G. Liu, F. Worgotter and I. Markelic, 2011. Nonlinear
estimation using central difference information filter.
In
IEEE Workshop Statistical Signal Processing. 593-
596.
Q. Ge, Daxing Xu, and Chenglin Wen, 2014. Cubature
information filters with correlated noises and their
applications in decentralized fusion. In
Journal of
Signal Processing
, 94, 434-444.
B. D. O. Anderson and J.B. Moore, 1979.
Optimal
Filtering
, Prentice Hall, Englewood Cliffs, 1
st
edition.
M. Nørgaard, N. K. Poulsen, and O. Ravn, 2000. New
developments in state estimation for nonlinear
systems. In
Automatica, 36 (11), 1627-1638.
MultipleSensorFusionusingAdaptiveDividedDifferenceInformationFilter
405