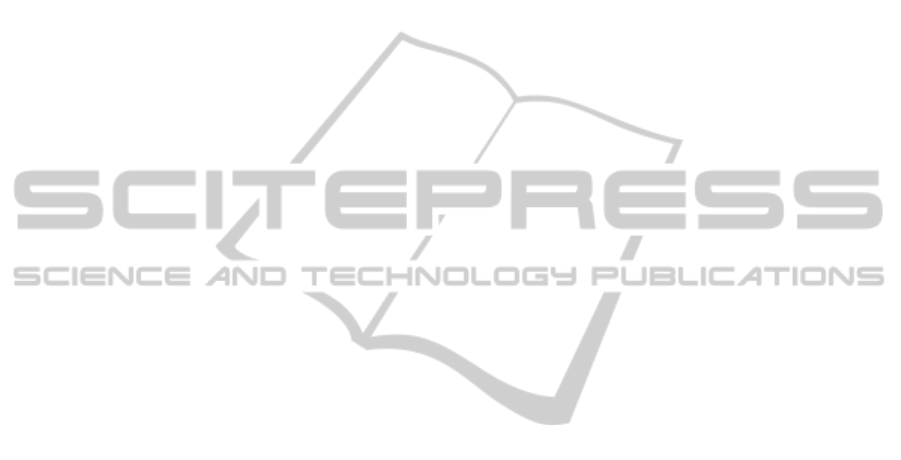
MemoryUsage1 and MemoryUsage6 refers re-
spectively to the MemoryUsage of the machines sup-
porting respectively the web service ”check ware-
house availability” and ”check shipment availability”.
Regarding the huge number and the variety of com-
pute instances offered by Cloud provider, the gener-
ated classification rule presents a means to limit the
range of choices. Referring to our example, enter-
prises should focus only on finding computing in-
stances providing better memory usage for the exe-
cution of the identified web services
5 CONCLUSION
Monitoring SOA based business processes presents
an interesting means for SMEs to analyze, interpret
and ameliorate the business process performance. De-
spite the evolving number of researches focusing on
that issue, realizing a method for identifying essen-
tial processes to monitor, identifying and monitoring
business and Qos metrics is not tackled in the best of
our knowledge. Thus, the framework presents a perti-
nent solution to assist business/IT experts for achiev-
ing enterprise goals. The tool we presented in this
paper is characterized by its top down aspect. In fact,
it links between business and IT levels helping ex-
perts to depict the relation between eventual KPI vi-
olation and the concerned IT properties. Moreover,
the framework identify the outsourcing to the Cloud
requirements. The presented framework is beneficial
for both IT and business levels for displaying, analyz-
ing and enhancing different business processes data.
We are working on defining an outsourcing algo-
rithm allowing to depict most relevant business pro-
cess parts to outsource and the evaluation the perfor-
mance of business processes executed in the Cloud.
REFERENCES
Barth, W. (2006). Nagios. System and Network Monitoring.
No Starch Press, u.s. ed edition.
Comes, D., Bleul, S., Weise, T., and Geihs, K. (2009). A
flexible approach for business processes monitoring.
In Senivongse, T. and Oliveira, R., editors, Distributed
Applications and Interoperable Systems, volume 5523
of Lecture Notes in Computer Science, pages 116–
128. Springer Berlin Heidelberg.
del Ro-Ortega, A., Resinas, M., and Ruiz-Corts, A. (2010).
Defining process performance indicators: An ontolog-
ical approach. In Meersman, R., Dillon, T., and Her-
rero, P., editors, On the Move to Meaningful Internet
Systems: OTM 2010, volume 6426 of Lecture Notes
in Computer Science, pages 555–572. Springer Berlin
Heidelberg.
del Ro-Ortega, A., Resinas Arias de Reyna, M., Durn Toro,
A., and Ruiz-Corts, A. (2012). Defining process per-
formance indicators by using templates and patterns.
In Barros, A., Gal, A., and Kindler, E., editors, Busi-
ness Process Management, volume 7481 of Lecture
Notes in Computer Science, pages 223–228. Springer
Berlin Heidelberg.
Delgado, A., Weber, B., Ruiz, F., de Guzmn, I. G.-R., and
Piattini, M. (2014). An integrated approach based on
execution measures for the continuous improvement
of business processes realized by services. Informa-
tion and Software Technology, 56(2):134 – 162.
Friedenstab, J., Janiesch, C., Matzner, M., and Muller, O.
(2012). Extending bpmn for business activity moni-
toring. In 2012 45th Hawaii International Conference
on System Science (HICSS), pages 4158–4167.
Grati, R., Boukadi, K., and Ben-Abdallah, H. (2012). A qos
monitoring framework for composite web services in
the cloud. In In The Sixth International Conference on
Advanced Engineering Computing and Applications
in Sciences (Advcomp12).
Hall, M., Frank, E., Holmes, G., Pfahringer, B., Reutemann,
P., and Witten, I. H. (2009). The weka data min-
ing software: An update. SIGKDD Explor. Newsl.,
11(1):10–18.
Josuttis, N. (2007). Soa in Practice: The Art of Distributed
System Design. O’Reilly Media, Inc.
Mell, P. M. and Grance, T. (2011). Sp 800-145. the nist defi-
nition of cloud computing. Technical report, Gaithers-
burg, MD, United States.
(OMG), O. M. G. (2011). Business process model and no-
tation (bpmn) version 2.0. Technical report.
Pan, W. and Wei, H. (2012). Research on key performance
indicator (kpi) of business process. In 2012 Second
International Conference on Business Computing and
Global Informatization (BCGIN), pages 151–154.
Quinlan, J. R. (1986). Induction of decision trees. Mach.
Learn., 1(1):81–106.
Ruijie, L. and Hong, H. (2010). Business process metrics
modeling based on model-driven. In 2010 IEEE In-
ternational Conference on Software Engineering and
Service Sciences (ICSESS), pages 327–330.
Wetzstein, B., Leitner, P., Rosenberg, F., Dustdar, S., and
Leymann, F. (2011). Identifying influential factors of
business process performance using dependency anal-
ysis. Enterprise IS, 5(1):79–98.
Wetzstein, B., Strauch, S., and Leymann, F. (2009). Mea-
suring performance metrics of ws-bpel service com-
positions. International conference on Networking
and Services (ICNS’06), 0:49–56.
Yang, D.-H., Kim, S., Nam, C., and Min, J.-W. (2007).
Developing a decision model for business process
outsourcing. Computers and Operations Research,
34(12):3769 – 3778. Operations Research and Out-
sourcing.
Zhang, J., Nie, W., Panahi, M., Chang, Y., and Lin, K.-J.
(2009). Business process composition with qos op-
timization. In Commerce and Enterprise Computing,
2009. CEC ’09. IEEE Conference on, pages 499–502.
zur Muehlen, M. and Shapiro, R. (2010). Business process
analytics. In Handbook on Business P rocess Manage-
ment 2, pages 137–157. Springer Berlin Heidelberg.
MeasurementFrameworkforBusinessProcessOutsourcingtotheCloud
55