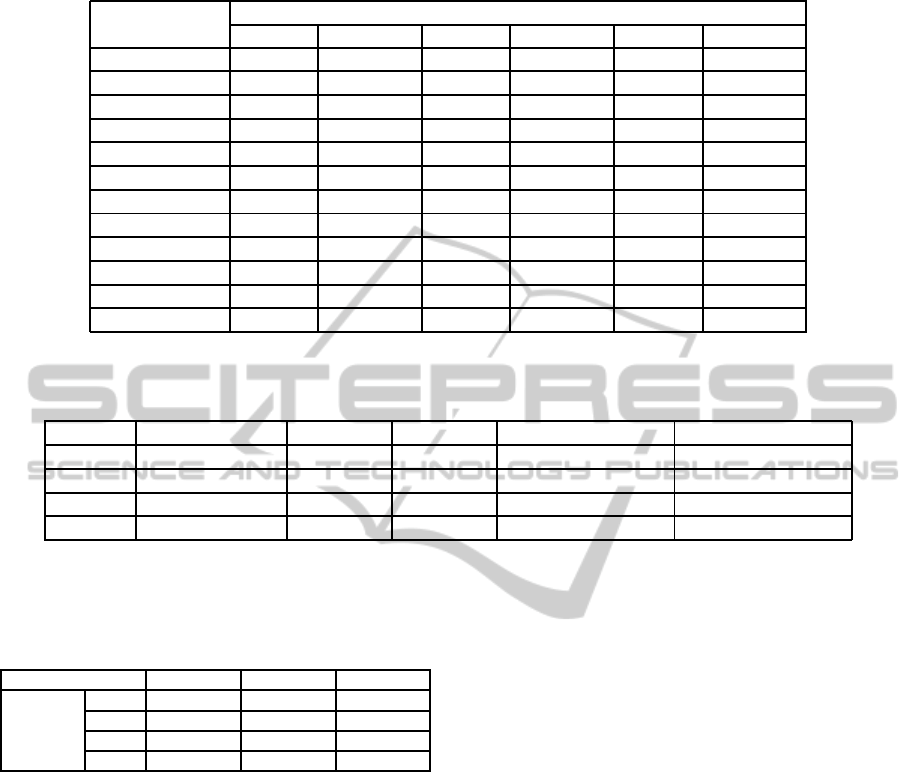
Table 4: Basic data(P
trans
is 0.09).
infected cases
0-4 5-18 19-29 30-64 65+ total
30%(baseline) 239,745 1,706,801 956,525 3,441,096 954,530 7,298,697
30%(p1) 220,505 1,630,385 882,697 3,165,843 673,903 6,573,333
30%(p2) 194,734 1,093,766 811,178 2,877,378 793,026 5,770,082
20%(baseline) 225,593 1,669,545 955,546 3,410,050 915,534 7,176,268
20%(p1) 206,630 1,587,315 875,068 3,113,949 620,845 6,403,807
20%(p2) 177,356 1,005,881 789,590 2,773,512 739,373 5,485,712
10%(baseline) 208,842 1,622,788 955,966 3,371,757 873,679 7,033,032
10%(p1) 191,289 1,542,433 873,912 3,072,764 584,062 6,264,460
10%(p2) 161,939 958,344 781,978 2,712,316 698,209 5,312,786
00%(baseline) 199,815 1,567,902 847,755 3,034,015 717,711 6,367,198
00%(p1) 187,902 1,506,673 783,991 2,801,450 462,770 5,742,786
00%(p2) 141,077 832,923 640,380 2,246,715 525,263 4,386,358
p1 is elder only.
p2 is student only.
Table 5: Cost in Meltzer’s work(P
trans
is 0.09).
EC ratio baseline(x10
9
$) p1(x10
9
$) p2(x10
9
$) baseline-p1(x10
9
$) baseline-p2(x10
9
$)
30% 54.03 48.18 44.16 5.85 9.87
20% 53.24 47.01 42.23 6.23 11.01
10% 52.34 46.12 41.06 6.22 11.28
0.0% 46.78 41.70 33.63 5.08 13.15
p1 is elder only.
p2 is student only.
Table 6: The equilibrium point under different transmission
probability and EC ratio.
P
trans
0.09 P
trans
0.10 P
trans
0.11
EC ratio
30% 7.74 3.99 2.85
20% 8.74 4.16 2.93
10% 9.34 4.40 3.03
0.0% 22.71 7.15 4.39
distribution well. Interval estimations of normal dis-
tribution can then be applied.
Second, it is observed that policy options depends
on the transmissibility of the virus which is not ob-
servable before the pandemic starts. Therefore, a
carefully designed early estimation process is very
important. The process will utilize the early data
about the epidemic to predict important parameters
which are important for decision makers. We believe
that early data, say for the first 2 months, can be ap-
plied to get good estimations. But more experiments
are necessary to ensure it.
Third, more policy options should be evaluated. In
this study, we only consider two options. To make real
life recommendations, more options should be evalu-
ated. Forth, it is beneficial to design good visualiza-
tion methods to facilitate decision process.
REFERENCES
Fu, Y.-c., Wang, D.-W., and Chuang, J.-H. (2012). Repre-
sentative contact diaries for modeling the spread of in-
fectious diseases in taiwan. PLoS One, 7(10):e45113.
Geard, N., McCaw, J. M., Dorin, A., Korb, K. B., and
McVernon, J. (2013). Synthetic population dynam-
ics: A model of household demography. Journal of
Artificial Societies and Social Simulation, 16(1):8.
Germann, T. C., Kadau, K., Longini, I. M., and Macken,
C. A. (2006). Mitigation strategies for pandemic in-
fluenza in the united states. Proceedings of the Na-
tional Academy of Sciences, 103(15):5935–5940.
House, T. and Keeling, M. J. (2009). Household struc-
ture and infectious disease transmission. Epidemiol-
ogy and infection, 137(05):654–661.
Meltzer, M. I., Cox, N. J., Fukuda, K., et al. (1999). The
economic impact of pandemic influenza in the united
states: priorities for intervention. Emerging infectious
diseases, 5:659–671.
OECD (2011). Doing better for families. Technical report,
Paris.
Trends, C. (2013). World family map 2013: Mapping family
change and child well-being outcomes. World Family
Map.
Tsai, M.-T., Chern, T.-C., Chuang, J.-H., Hsueh, C.-W.,
Kuo, H.-S., Liau, C.-J., Riley, S., Shen, B.-J., Shen,
C.-H., Wang, D.-W., et al. (2010). Efficient simula-
tion of the spatial transmission dynamics of influenza.
PloS one, 5(11):e13292.
TheImpactofHouseholdStructuresonPandemicInfluenzaVaccinationPriority
487